Assignment4Sol
.pdf
keyboard_arrow_up
School
New York University *
*We aren’t endorsed by this school
Course
0266
Subject
Economics
Date
Apr 3, 2024
Type
Pages
5
Uploaded by ChancellorLapwing4188 on coursehero.com
Assignment 4: ECON-UA 266 - Intro to Econometrics
Sahar Parsa
Spring 2024
The solution to this assignment will be released on Friday March 1, 2024. It covers the material related to
OLS, OLS interpretation and economic significance.
Question 1
Sir Francis Galton, a cousin of James Darwin, examined the relationship between the height of children and
their parents towards the end of the 19th century. It is from this study that the name “regression’ ’ originated.
You decide to update his findings by collecting data from 110 college students, and estimate the following
relationship:
Student
−
height
= 19
.
6 + 0
.
73
×
Midpar
−
height, R
2
= 0
.
45
, SER
= 2
.
0
, where the standard error of the
intercept is
7
.
2
and the standard error of the slope is
0
.
1
.
where Student-height is the height of students in inches, and Midpar-height is the average of the parental
heights. Values in parentheses are standard errors.
(Following Galton’s methodology, both variables were
adjusted so that the average height of parents was equal to the average height of students — We will more
generally assume the heights come from the same distribution.)
a.
Explain the elasticity method to the interpretation of the estimated coefficient?
What about the
standardized coefficient method? Why do we need these methods to interpret the economic significance
of the estimated coefficient?
Answer
The elasticity method is given by the formula:
χ
Y X
=
∆
Y
i
Y
i
∆
X
i
X
i
which corresponds to the
%
change in
Y
i
to a
1%
change in
X
i
. Noting that
∆
Y
i
∆
X
i
=
β
OLS
we have that:
χ
XY
=
β
OLS
X
i
Y
i
For the standardized coefficient method we first standardized the data (
Y
i
,
X
i
) to (
Y
st
i
,
X
st
i
) where:
X
st
i
=
X
i
−
¯
X
S
X
and
Y
st
i
=
Y
i
−
¯
Y
S
Y
1
This transforms both the X and Y data on the same scale, both having the same mean (0) and standard
deviation (1). We then run the following regression:
Y
st
i
=
β
st
X
st
i
+
ε
st
i
In this case,
β
st
will correspond to the change (in standard deviation units) in
Y
st
i
to a one standard deviation
unit change in
X
st
i
. Alternatively, we know from the lectures that we can find the OLS estimator for
β
st
by
using the
β
st
OLS
=
β
OLS
S
X
S
Y
.
We need these methods to interpret the economic significance of the estimated coefficients as regressions are
not invariant to scaling of the data. For example, if we multiply
Y
i
by a constant
a
then the OLS coefficients
will be scaled by a factor of
a
. However, the elasticity and standardization methods are invariant to the
original scaling of the data and allow us to interpret regressions on a common scale (e.g. the
%
change or the
standard deviation unit change).
b. Interpret the estimated coefficients using the elasticity method.
Answer
Note
∆
Y
i
∆
X
i
=
ˆ
β
= 0
.
73
corresponds to slope of the regression line. So in this case we have
χ
XY
= 0
.
73
X
i
Y
i
In practice we evaluate this at the sample average (
¯
X,
¯
Y
). We assumed in this question that the parents and
the students have the same distribution of heights and
¯
X
=
¯
Y
.
χ
X,Y
= 0
.
73
. As such, if one student has
parents who are on average
1%
taller than the parents of another student, that student is predicted to be
0
.
73%
taller than the student whose parents are shorter.
c. Interpret the estimated coefficients using the standardize method.
Answer
We can use the formula:
β
st
OLS
=
β
OLS
S
X
S
Y
. Again, the parents and the students have the same distribution
of heights and
S
X
=
S
Y
and
β
st
OLS
= 0
.
73
. A one standard deviation change in
X
is associated with a
0
.
73
standard deviation change in
Y
.
d. What is the prediction for the height of a child whose parents have an average height of
70
.
06
inches?
Solution
Subbing in 70.06 for midpar-height we obtain:
ˆ
Student
−
Height
= 19
.
6 + 0
.
73(70
.
06) = 70
.
74
The child is predicted to have a height of 70.74 inches.
d.
Given the positive intercept and the fact that the slope lies between zero and one, what can you say
about the height of students who have quite tall parents? Who have quite short parents?
Solution
We can say that students’ of tall parents are predicted to be taller on average than students’ of short parents.
We can’t necessarily conclude that they are likely to be tall or short in the population distribution of height.
This would depend on knowing the mean of the height distribution and the specific value of mid-par height.
e.
Galton was concerned about the height of the English aristocracy and referred to the above result as
“regression towards mediocrity.” Can you figure out what his concern was? Why do you think that we
refer to this result today as “Galton’s Fallacy?”
2
Solution
He is referring to the fact that if a child’s parents are above (or indeed below) a certain height threshold, the
child is predicted to be smaller (or taller) than his/her parents. To see this explicitly, note that their is a
unique X that solves:
X
= 19
.
6 + 0
.
73(
X
)
Solving for X gives 72.59 inches. Since the slope is less than 1, this means that if the parent’s height is above
72.59 inches the child is predicted to be smaller than the parent. Galton’s Fallacy refers to the fact he inferred
that were as a reduction in the spread (or variance) of height distribution as people are regressing toward the
mean. This is not the case, the likelihood is tall parents will have children that are shorter than them but
there is still a positive chance that this will not be case.
Galton regression is what gave the name regression to a linear regression because of the regression to the
mean: https://rss.onlinelibrary.wiley.com/doi/full/10.1111/j.1740-9713.2011.00509.x
Question 2
Consider the following random sample
{
X
i
, i
= 1
,
· · ·
, N
}
.
1. Write down the formula for the standardized
X
i
, denote it
X
st
i
Answer
X
st
i
=
X
i
−
¯
X
S
X
where
S
X
=
wwwwwww
vvvvvvv
vvvvvvv
uuuuuuu
YYYYYYY
i
(
X
i
−
¯
X
)
2
N
−
1
2.
Show that
X
st
i
has a sample mean of
0
and a sample standard deviation fo
1
. [Notice that this is where
the name standardized comes from.]
Answer
For the sample mean
¯
X
st
=
QQQQQQQ
i
X
st
i
N
=
QQQQQQQ
i
X
i
−
¯
X
S
X
N
Which equals
QQQQQQQ
i
X
i
−
¯
X
S
X
N
= 0
Since
QQQQQQQ
i
X
i
−
¯
X
=0.
For the sample standard deviation
S
x
st
=
ttttttt
QQQQQQQ
i
(
X
st
i
−
¯
X
st
)
2
N
−
1
=
ttttttt
QQQQQQQ
i
(
X
st
i
)
2
N
−
1
Thus we have
3
Your preview ends here
Eager to read complete document? Join bartleby learn and gain access to the full version
- Access to all documents
- Unlimited textbook solutions
- 24/7 expert homework help
Related Questions
Question:
1. Please chose a topic you are interested in within social policy-education, health care, mental health etc. and write out a hypothetical regression estimation for this topic. Make sure to identify the ideal outcome variable, explanatory variable, and control variables.
arrow_forward
Q5. Show that µY = Yµ − µY · 1. Data Mining Regression Evaluation chapter
arrow_forward
A multiple regression model, K = a + bX + cY + dZ, is estimated regression software, which produces the following output:
a. Are the estimates of a, b, c, and d statistically significant at the 1 percent significance level?
b. How much of the total variation is explained by this regression equation?
c. Is the overall regression equation statistically significant at the 1 percent level of significance?
d. If X equals 50, Y equals 200, and Z equals 45, what value do you predict K will take?
arrow_forward
Please make a Data set and regression equation based on Student Debt and inflation with the following variables: Current student debt,interest rates, inflation rates ( will give thumbs up ) thank you so much.
arrow_forward
What are the most important remaining threats to the internal validity of this regression analysis?
arrow_forward
what are the key features , Strength and limitation of following model? and when which model should be used?
Ordinary Least Squares
Logit regression model
Probit regression model
arrow_forward
4- The manager of Collins Import Autos believes the number of cars sold in a day(Q) depends on two factors: (1) the number of hours the dealership is open (H) and (2) the number of salespersons working that day (S ). After collecting data for two months (53 days), the manager estimates the following log-linear model:
Q = aHbSc
-----
a. Explain how to transform this log-linear model into a linear form that can be estimated using multiple regression analysis.
b. How do you interpret coefficients b and c? If the dealership increases the number of salespersons by 20 percent, what will be the percentage increase in daily sales?
c. Test the overall model for statistical significance at the 5 percent significance level.
arrow_forward
Investigate what factors determine the number of times a person logs into Facebook per week. It is argued that these four factors are important: number of friends, age in years, whether the person is employed, and whether the student has a Twitter account. That is:
FACEBOOK LOGIN=f(FRIENDS,AGE,EMPLOYED,TWITTER)
Do you think other relevant explanatory variables should also be included? Name any two such variables and explain why they should be included in the regression.
arrow_forward
Consider the following estimated regression model relating annual salary to years of education and work experience.
Estimated Salary=11,722.40+3182.56(Education)+1202.44(Experience)Estimated Salary=11,722.40+3182.56(Education)+1202.44(Experience)
Suppose an employee with 66 years of education has been with the company for 33 years (note that education years are the number of years after 8th8th grade). According to this model, what is his estimated annual salary?
arrow_forward
In 2017, Philadelphia launched a sweetened beverage tax of 1.5 cents per ounce, raising the cost of a 2-liter soda bottle from about $1.50 to $2.50. One year later, the Philadelphia mayor wants to evaluate if this "sugar tax" improves the health status of Philadelphia
Propose ONE method (i.e. difference-in-difference, instrumental variables, or regression discontinuity) to address these questions. write down its implementation details (the type of data you need, potential sources to get the data, equations) its pros and cons
Only Typing answer please
I need ASAP
arrow_forward
Consider the following estimated regression model relating annual salary to years of education and work experience. Estimated Salary=11,681.31+3418.97(Education)+1194.78(Experience) Suppose two employees at the company have been working there for five years. One has a bachelor's degree (8 years of education) and one has a master's degree (10 years of education). How much more money would we expect the employee with a master's degree to make?
arrow_forward
q9-
Which property of linear regression is related with the size effects of individual units in a cross-section data?
Select one:
a.
Heteroskedasticity
b.
Endogeneity
c.
Autocorrelation
d.
Non-normality
Clear my choice
arrow_forward
Topic: Simple Regression
arrow_forward
Question 15
When the R2 of a regression equation is very high, it indicates that
all the coefficients are statistically significant.
the intercept term has no economic meaning.
a high proportion of the variation in the dependent variable can be accounted for by the variation in the independent variables.
there is a good chance of serial correlation and so the equation must be discarded.
arrow_forward
Demand Estimation for The San Francisco Bread Company
Consider the hypothetical example of The San Francisco Bread Company, a San Francisco-based chain of bakery/cafes. San Francisco Bread Company has initiated an empirical estimation of customer traffic at 30 regional locations to help the firm formulate pricing and promotional plans for the coming year. Annual operating data for the 30 outlets appear in the attached Table 1.
The following regression equation was fit to these data:
Qi = b0 + b1Pi + b2Pxi + b3Adi + b4Ii + uit.
Where: Q is the number of meals served,
P is the average price per meal (customer ticket amount, in dollars),
Px is the average price charged by competitors (in dollars),
Ad is the local advertising budget for each outlet (in dollars),
I is the average income per household in each outlet’s service area,
ui…
arrow_forward
A company wants to use regression analysis to forecast the demand for the next quarter.In such a regression model, demand would be the independent variable. True or false?a. Trueb. False
arrow_forward
Economics: Industrial Economics
Since only 3 questions allowed = Please Answer Parts D. - F. (and include answers to the NOTE PART: sub-questions a.; b.; c. In order to solve the Parts D. - F. questions). Thanks
QUESTION:
You work for the manager of a small single-product firm. Assume that you have data for your firm on costs, capital rental rate, wages and output. You realize through regression analysis that the data fits very well the following equation:
ln(C) = -1.18 + 0.33 ln(r) + 0.67 ln(w) + 0.81 ln(q)
D. Explain why its very likely that you misspecified the production technology.
To account for the possibility of having misspecified the production function, you run a more general regression using the data for all firms. The data fits the following equation:
ln(C) = 1 + 0.33 ln(r) + 0.67 ln(w) + 0.3 ln(q) + 0.03[ln(q)]^2
E.Assuming that w = 25 and r = 500, complete the following table (in excel): q ln(q) ln(C) C AC MC 1,000 2,000 5,000 10,000 20,000 50,000 100,000 200,000…
arrow_forward
1) State in algebraic notation and explain the assumption about the classical linear regression models disturbances that are referred to by the term ‘homoscedasticity’.
arrow_forward
( TRUE OR FALSE help me find the true or false questions )
1. In economic statistics and Econometrics, we do the same thing.( )
2. As same in regression analysis, variables in relation analysis are all random variables.( )
3. Known as residual, "i is an estimate of u , the random disturbance term.( )
4. The slope coefficient of the log-log model measures the elasticity of Y with respect to X.( )
5. In regression of standardized variables, the intercept term is always zero.( )
6. The underlying theory may suggest a particular functional form.( )
7. The disturbance term u is assumed to follow normal distribution.( )
8. White test is used to check if there exists multicollinearity in the disturbance term of a regression function.( )
9. Dummy variable can be used to test the stability of a regression model just as the function of the Chow Test.( )
10. Where there is autocorrelation in the u , the OLS estimators are not BLUE estimators any more.( )
arrow_forward
Econometrics (forecasting) topic:
Explain the difference between a nested and a non-nested model? Provideexamples.
arrow_forward
Hello,
I am trying to find the equations on my calculator for the price-demand and price supply equations. The data is in the attached image.
I think I am doing something wrong, but not sure what.
I found the quadratic regression model for the first set of data using my calculator, but I used the p=D(x) as list one, and x, as list two. I came up with
0.028x^2-23x +5743
is this right? or do I need the reverse the order?
For the price-supply data I but the p=S(x) as list 1 and x as list 2 and I got the linear regression function:
2 5.1x+342
Can you please let me know if I am on the right track?
arrow_forward
In a simple linear regression equation, if X increases by 3:
Select one:
a. Y increases by B1
b. Y increases by B1/3
c. Y increases by 3 * (Bo + B1)
d. none of the above
arrow_forward
Explain carefully why running the regression above might suffer from endogeneity concerns: are their any unobservable variables that might confound the results? Should we be worried about reverse causality? What empirical methods could we use to address these concerns?
arrow_forward
Each cell represents a regression with a different outcome variable from Krueger’s (1993) study of workers who use computers. The left hand side variable is log wages. Answer based on column 2 of the above regression table.
1. Write a sentence that explains the relationship the regression is describing in row 2.
2. What is the number in parenthesis? What is it trying to communicate?
3. Write a causal statement that captures what the authors are trying to argue in row 2.
arrow_forward
18. A multiple regression model, K = a + bX + cY + dZ, is estimated regression software, which produces the following output: D. If X equals 50, Y equals 200, and Z equals 45, what value do you predict K will take?
arrow_forward
What is difference between regression model, and estimated regression equation?
arrow_forward
1-R2 k -1 b-briefly explain the reasons for the following statements to be true or false - The classical linear regression model is concerned with heteroskedacity, autocorelation, and multiple linear connection main mass, which express deviations from its assumptions. If the H0 hypothesis is rejected according to the F test and the H0 hypothesis cannot be rejected for all parameters according to the T test, the problem here is which variables will be excluded from the model. - R2 value is high due to trend effect while working with cross-sectional data in Applied Studies× R2 n-k =ûú6 24ëê ùS2 +(K−3)2é) a - for which purposes in econometric applications of equations or equations mentioned below explain their use = 1- (1-R 2 ) n-1 n-k = n
arrow_forward
SEE MORE QUESTIONS
Recommended textbooks for you
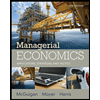
Managerial Economics: Applications, Strategies an...
Economics
ISBN:9781305506381
Author:James R. McGuigan, R. Charles Moyer, Frederick H.deB. Harris
Publisher:Cengage Learning
Related Questions
- Question: 1. Please chose a topic you are interested in within social policy-education, health care, mental health etc. and write out a hypothetical regression estimation for this topic. Make sure to identify the ideal outcome variable, explanatory variable, and control variables.arrow_forwardQ5. Show that µY = Yµ − µY · 1. Data Mining Regression Evaluation chapterarrow_forwardA multiple regression model, K = a + bX + cY + dZ, is estimated regression software, which produces the following output: a. Are the estimates of a, b, c, and d statistically significant at the 1 percent significance level? b. How much of the total variation is explained by this regression equation? c. Is the overall regression equation statistically significant at the 1 percent level of significance? d. If X equals 50, Y equals 200, and Z equals 45, what value do you predict K will take?arrow_forward
- Please make a Data set and regression equation based on Student Debt and inflation with the following variables: Current student debt,interest rates, inflation rates ( will give thumbs up ) thank you so much.arrow_forwardWhat are the most important remaining threats to the internal validity of this regression analysis?arrow_forwardwhat are the key features , Strength and limitation of following model? and when which model should be used? Ordinary Least Squares Logit regression model Probit regression modelarrow_forward
- 4- The manager of Collins Import Autos believes the number of cars sold in a day(Q) depends on two factors: (1) the number of hours the dealership is open (H) and (2) the number of salespersons working that day (S ). After collecting data for two months (53 days), the manager estimates the following log-linear model: Q = aHbSc ----- a. Explain how to transform this log-linear model into a linear form that can be estimated using multiple regression analysis. b. How do you interpret coefficients b and c? If the dealership increases the number of salespersons by 20 percent, what will be the percentage increase in daily sales? c. Test the overall model for statistical significance at the 5 percent significance level.arrow_forwardInvestigate what factors determine the number of times a person logs into Facebook per week. It is argued that these four factors are important: number of friends, age in years, whether the person is employed, and whether the student has a Twitter account. That is: FACEBOOK LOGIN=f(FRIENDS,AGE,EMPLOYED,TWITTER) Do you think other relevant explanatory variables should also be included? Name any two such variables and explain why they should be included in the regression.arrow_forwardConsider the following estimated regression model relating annual salary to years of education and work experience. Estimated Salary=11,722.40+3182.56(Education)+1202.44(Experience)Estimated Salary=11,722.40+3182.56(Education)+1202.44(Experience) Suppose an employee with 66 years of education has been with the company for 33 years (note that education years are the number of years after 8th8th grade). According to this model, what is his estimated annual salary?arrow_forward
- In 2017, Philadelphia launched a sweetened beverage tax of 1.5 cents per ounce, raising the cost of a 2-liter soda bottle from about $1.50 to $2.50. One year later, the Philadelphia mayor wants to evaluate if this "sugar tax" improves the health status of Philadelphia Propose ONE method (i.e. difference-in-difference, instrumental variables, or regression discontinuity) to address these questions. write down its implementation details (the type of data you need, potential sources to get the data, equations) its pros and cons Only Typing answer please I need ASAParrow_forwardConsider the following estimated regression model relating annual salary to years of education and work experience. Estimated Salary=11,681.31+3418.97(Education)+1194.78(Experience) Suppose two employees at the company have been working there for five years. One has a bachelor's degree (8 years of education) and one has a master's degree (10 years of education). How much more money would we expect the employee with a master's degree to make?arrow_forwardq9- Which property of linear regression is related with the size effects of individual units in a cross-section data? Select one: a. Heteroskedasticity b. Endogeneity c. Autocorrelation d. Non-normality Clear my choicearrow_forward
arrow_back_ios
SEE MORE QUESTIONS
arrow_forward_ios
Recommended textbooks for you
- Managerial Economics: Applications, Strategies an...EconomicsISBN:9781305506381Author:James R. McGuigan, R. Charles Moyer, Frederick H.deB. HarrisPublisher:Cengage Learning
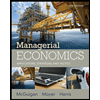
Managerial Economics: Applications, Strategies an...
Economics
ISBN:9781305506381
Author:James R. McGuigan, R. Charles Moyer, Frederick H.deB. Harris
Publisher:Cengage Learning