HW4 - Long term system planning
.docx
keyboard_arrow_up
School
North Carolina State University *
*We aren’t endorsed by this school
Course
300
Subject
Economics
Date
Apr 3, 2024
Type
docx
Pages
5
Uploaded by MegaPelican2226 on coursehero.com
ES 300 – Homework 4 Long term system planning
Note:
all data needed to complete this assignment are in the HW4 Data.xlsx file posted on Moodle. You are chief demand forecaster at the friendly neighborhood electric utility, and are trying to build a model that will predict “peak system demand” in the year 2024. To start with, you have a bunch of historical information for the past 20 years, including peak demand data and records of four other variables that might help explain how peak demand changes year-to-year. Note that 1 GW = 1000 MW.
You decide
to start
with
some exploratory analysis of the pairwise relationships between peak demand and the other variables. 1)
First, create four separate “scatter plots” of peak electricity demand versus (show them in different graphs). You must label each axis to get
credit!
a.
Economic index
Year
Peak
Demand
(GW)
Economic
Index
Population
(million)
Per Capita
Energy use
Metric
Max Average
Daily Temp (F)
2004
19.34
1.10
4.00
1.30
85.46
2005
20.11
1.30
4.08
1.40
86.99
2006
19.83
1.40
4.14
1.40
86.00
2007
20.20
1.50
4.21
1.50
84.47
2008
20.47
1.80
4.27
1.50
86.99
2009
20.70
1.60
4.35
1.40
89.42
2010
20.52
2.67
4.44
1.10
89.42
2011
20.81
1.78
4.55
1.20
90.50
2012
20.20
-0.29
4.64
1.00
86.00
2013
19.78
-2.78
4.72
0.98
86.00
2014
20.81
2.53
4.79
0.98
86.99
2015
20.67
1.60
4.85
0.97
84.47
2016
20.98
2.22
4.90
0.96
86.99
2017
20.49
1.80
4.95
0.94
83.48
2018
20.85
2.00
5.00
0.94
84.56
2019
20.74
2.40
5.06
0.93
83.56
b. Population
c.
Per capita energy use
d.
Maximum average daily temperature
Since no single variable is a perfect predictor of peak demand, you think it would be wise to create a model that uses information from all four variables to help predict demand. You use least squares regression to fit the following model.
Y
T = aw
T
+ bx
T
+ cy
T
+ dz
T
Where, Y
T = predicted peak demand in year T (in GW)
w
T
= economic index in year T
x
T
= population in year T
y
T
= per capita energy use in year T
z
T
= maximum average daily temperature in year T
The “best fit” coefficients are found to be:
a = 0.1758
b = 1.344
c = 0.220
d = 0.1595
With your trusty model in hand, you set out to predict future demand. The person
in the cubicle next to you passes you a series of forecasts that describe the state of the world in the years 2020-2024. Here is what it says:
Year
Economic
Index
Populatio
n (million)
Per Capita
Consumptio
n Metric
2020
0.90
5.15
0.90
2021
0.95
5.25
0.85
2022
1.00
5.40
0.80
2023
1.05
5.55
0.80
2024
1.10
5.75
0.78
What you don’t know is what the maximum average daily temperature will be in these future years (no one does!). You decide a reasonable way to look into this would be to use historical temperature data from 1954-2019:
2. Look at the historical temperature data listed in the ‘HistoricalTemps’ worksheet (look at the bottom of the screen in Excel), please calculate two values:
Your preview ends here
Eager to read complete document? Join bartleby learn and gain access to the full version
- Access to all documents
- Unlimited textbook solutions
- 24/7 expert homework help
Related Questions
Tom Glass forecasts electrical demand for the Flatlands Public Power District (FPPD). The FPPD wants to take its Comstock power plant out of service for maintenance when demand is expected to be low. After shutdown, performing maintenance and getting the plant back on line takes two weeks. The utility has enough other generating capacity to satisfy 1,550 megawatts (MW) of demand while Comstock is out of service. Table shows weekly peak demands (in MW) for the past several autumns. When next in year 6 should the Comstock plant be scheduled for maintenance?
arrow_forward
An investment services company experienced dramatic growth in the last two decades. The following models for the company's revenue R and expenses or costs C (both in millions of dollars) are functions of the years past 1990.
R(t) = 21.4e0.131t and C(t) = 18.6e0.131t
(a) Use the models to predict the company's profit in 2023. (Round your answer to one decimal place.) $ million (b) How long before the profit found in part (a) is predicted to double? (Round your answer to the nearest whole number.) years after 1990
arrow_forward
Bell Greenhouses has estimated its monthly demand for potting soil to be the following:
Assume this trend factor is expected to remain stable in the foreseeable future. The following table contains the monthly seasonal adjustment factors, which have been estimated using actual sales data from the past five years:
MONTH
ADJUSTMENT FACTOR (%)
March
+2
June
+15
August
+10
December
−12
a.
Forecast Bell Greenhouses’ demand for potting soil in March, June, August, and December 2007.
Answer
b.
If the following table shows the forecasted and actual potting soil sales by Bell Greenhouses for April in five different years, determine the seasonal adjustment factor to be used in making an April 2008 forecast.
YEAR
FORECAST
ACTUAL
2007
500
515
2006
452
438
2005
404
420
2004
356
380
2003
308
320
arrow_forward
Consider the “Trip Logistic” example discussed in class (i.e. base case). Please solve the same problem with each of the following changes, and compare the results with those of the basecase:
a) The spot market price (in period 0) is reduced to $0.55 per sq. ft. per year. Assume that everything else remains the same as the base-case.
b) There is a lower demand uncertainty: Demand can go up by 5% with p= 0.5 or down by 5% with 1 − p= 0.5. Assume that everything else remains the same as the base-case.
arrow_forward
A firm keeps a record of sales and prices over the past seven months, resulting in the following table: Price (ZMW/ton) Sales (tons) Nov. 1985 7.5 84.5 Dec. 8.0 82.0 Jan. 1986 8.0 84.0 Feb. 7.2 92.0 March 7.0 95.0 April 8.0 92.0 May 8.5 91.5 Use these observations to estimate demand as a linear function of both price and time. Further, utilise this function to estimate demand for the following month, on the assumption that: (a) price remains unchanged, (b) price increases to ZMW9/ton. Hence estimate the price elasticity of demand between these prices and find the price which would maximise sales revenue. Given the nature of the observations, comment on any difficulties in interpreting your results for decision-making purposes.
arrow_forward
Why is knowing (or estimating) the product demand so crucial for a firm? What are the differences between estimating and forecasting demand? In your response, include an example of a business that has suffered from poorly forecasting the demand of its products. Evaluate how or why the business made such a mistake.To keep our discussion more interesting, please use examples that are not from our textbook.
arrow_forward
Hello
What is the Euler equation used for in this model in equation 7. This is a model from asset pricing.
c_t = Consumption
U = Utility
arrow_forward
Q4 (i) A business manager determines that t months after production begins on a new product, the number of units produced will be P thousand,where P(t) =6t2 + 5t (t + 1)2. What happens to production in the long run ? (ii) A ruptured pipe in a North Sea oil rig produces a circular oil slick that is y meters thick at a distance x meters from the rupture.Turbulence makes it difficult to directly measure the thickness of the slick at the source (where x = 0),but for x > 0,it is found that y =0.5(x2 + 3x) /x3 + x2 + 4x.Assuming the oil slick is continuously distributed,how thick would you expect it to be at the source?
arrow_forward
(i) A business manager determines that t months after production begins on a new product,the number of units produced will be P thousand, where P(t) =6?2 + 5?(? + 1)2.What happens to production in the long run ?(ii) A ruptured pipe in a North Sea oil rig produces a circular oil slick that is y meters thick at adistance x meters from the rupture. Turbulence makes it difficult to directly measure thethickness of the slick at the source (where x = 0), but for x > 0, it is found thaty =0.5(x2 + 3x)x3 + x2 + 4x . Assuming the oil slick is continuously distributed, howthick would you expect it to be at the source?
arrow_forward
Suppose you regress the annual percentage stock price returns on Russell 2000 small cap index for on a the percentage annual returns for a market index. Use the following output from this linear regression to answer questions a. - f.
a. What is the formula for the Russell 2000's characteristic line?
b. You forecast a market return of 6% next year. According to the market model, what is next year's expected return for the Russell 2000 index?
c. What is the correlation between the return on the Russell 2000 and the return on the market Index?
d. How much of the variation in the Russell 2000's returns are explained by the model?
e. Based on these regression results, the Russell 2000 index would be considered what kind of an investment, defensive, aggressive, or market neutral?
f. Does this regression have much explanatory power? Why or why not?
arrow_forward
Describe, in detail; how you can best fix the problem (provide pluses and minuses associated with your supplied problem solution)?
The phase of recession is the phase of business cycle in which the economic activities experience the downward trend. The demand in the economy falls , business investment decreases because of decreased scope of sales and unemployment rises leading to fall in GDP. The root cause inside the case is the declining sales and productivity of Moto's Integrated Controls Division (ICD) because of a drop in worldwide demand for machine devices prompted by the recession and savage rivalry. The ICD detailed a 5% downfall in sales in 1982 and again in the initial a half year of 1983, which put the future of the business in uncertainty. To keep the business running, the company needed to justify spending $3 million which it had done on various kinds of technological improvements and innovations , and there were questions about whether the investment could be…
arrow_forward
Demand for Orange Juice is given as Qd = 5000-2500 P + 1200 I +650E - 255 PS Suppose Income is I = Rs.500, Expectations E = 55, and Price of Ps= Rs 25. Find the Demand Equation. b. Using the demand function from part a., Calculate Elasticity of Demand for price range of Rs.125 and Rs. 155. What will be the 'Price Elasticity of Demand at P = Rs.125? d Interpret the Elasticity of Demand calculated in (C) above.
arrow_forward
OceanGate sells external hard drives for $184 each. Its total fixed costs are $27 million, and its variable costs per unit are $120. The corporate tax rate is 40%. If the economy is strong, the firm will sell 8 million drives, but if there is a recession, it will sell only half as many.
a. What will be the percentage decline in sales if the economy enters a recession (round answer to 2 decimal places)?
Percentage decline in sales
?%
b. What will be the percentage decline in profits if the economy enters a recession (round answer to 2 decimal places)?
Percentage decline in profits
?%
c. Calculate the operating leverage of this firm (round to 2 decimal places).
DOL
arrow_forward
SEE MORE QUESTIONS
Recommended textbooks for you
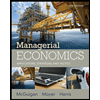
Managerial Economics: Applications, Strategies an...
Economics
ISBN:9781305506381
Author:James R. McGuigan, R. Charles Moyer, Frederick H.deB. Harris
Publisher:Cengage Learning
Related Questions
- Tom Glass forecasts electrical demand for the Flatlands Public Power District (FPPD). The FPPD wants to take its Comstock power plant out of service for maintenance when demand is expected to be low. After shutdown, performing maintenance and getting the plant back on line takes two weeks. The utility has enough other generating capacity to satisfy 1,550 megawatts (MW) of demand while Comstock is out of service. Table shows weekly peak demands (in MW) for the past several autumns. When next in year 6 should the Comstock plant be scheduled for maintenance?arrow_forwardAn investment services company experienced dramatic growth in the last two decades. The following models for the company's revenue R and expenses or costs C (both in millions of dollars) are functions of the years past 1990. R(t) = 21.4e0.131t and C(t) = 18.6e0.131t (a) Use the models to predict the company's profit in 2023. (Round your answer to one decimal place.) $ million (b) How long before the profit found in part (a) is predicted to double? (Round your answer to the nearest whole number.) years after 1990arrow_forwardBell Greenhouses has estimated its monthly demand for potting soil to be the following: Assume this trend factor is expected to remain stable in the foreseeable future. The following table contains the monthly seasonal adjustment factors, which have been estimated using actual sales data from the past five years: MONTH ADJUSTMENT FACTOR (%) March +2 June +15 August +10 December −12 a. Forecast Bell Greenhouses’ demand for potting soil in March, June, August, and December 2007. Answer b. If the following table shows the forecasted and actual potting soil sales by Bell Greenhouses for April in five different years, determine the seasonal adjustment factor to be used in making an April 2008 forecast. YEAR FORECAST ACTUAL 2007 500 515 2006 452 438 2005 404 420 2004 356 380 2003 308 320arrow_forward
- Consider the “Trip Logistic” example discussed in class (i.e. base case). Please solve the same problem with each of the following changes, and compare the results with those of the basecase: a) The spot market price (in period 0) is reduced to $0.55 per sq. ft. per year. Assume that everything else remains the same as the base-case. b) There is a lower demand uncertainty: Demand can go up by 5% with p= 0.5 or down by 5% with 1 − p= 0.5. Assume that everything else remains the same as the base-case.arrow_forwardA firm keeps a record of sales and prices over the past seven months, resulting in the following table: Price (ZMW/ton) Sales (tons) Nov. 1985 7.5 84.5 Dec. 8.0 82.0 Jan. 1986 8.0 84.0 Feb. 7.2 92.0 March 7.0 95.0 April 8.0 92.0 May 8.5 91.5 Use these observations to estimate demand as a linear function of both price and time. Further, utilise this function to estimate demand for the following month, on the assumption that: (a) price remains unchanged, (b) price increases to ZMW9/ton. Hence estimate the price elasticity of demand between these prices and find the price which would maximise sales revenue. Given the nature of the observations, comment on any difficulties in interpreting your results for decision-making purposes.arrow_forwardWhy is knowing (or estimating) the product demand so crucial for a firm? What are the differences between estimating and forecasting demand? In your response, include an example of a business that has suffered from poorly forecasting the demand of its products. Evaluate how or why the business made such a mistake.To keep our discussion more interesting, please use examples that are not from our textbook.arrow_forward
- Hello What is the Euler equation used for in this model in equation 7. This is a model from asset pricing. c_t = Consumption U = Utilityarrow_forwardQ4 (i) A business manager determines that t months after production begins on a new product, the number of units produced will be P thousand,where P(t) =6t2 + 5t (t + 1)2. What happens to production in the long run ? (ii) A ruptured pipe in a North Sea oil rig produces a circular oil slick that is y meters thick at a distance x meters from the rupture.Turbulence makes it difficult to directly measure the thickness of the slick at the source (where x = 0),but for x > 0,it is found that y =0.5(x2 + 3x) /x3 + x2 + 4x.Assuming the oil slick is continuously distributed,how thick would you expect it to be at the source?arrow_forward(i) A business manager determines that t months after production begins on a new product,the number of units produced will be P thousand, where P(t) =6?2 + 5?(? + 1)2.What happens to production in the long run ?(ii) A ruptured pipe in a North Sea oil rig produces a circular oil slick that is y meters thick at adistance x meters from the rupture. Turbulence makes it difficult to directly measure thethickness of the slick at the source (where x = 0), but for x > 0, it is found thaty =0.5(x2 + 3x)x3 + x2 + 4x . Assuming the oil slick is continuously distributed, howthick would you expect it to be at the source?arrow_forward
- Suppose you regress the annual percentage stock price returns on Russell 2000 small cap index for on a the percentage annual returns for a market index. Use the following output from this linear regression to answer questions a. - f. a. What is the formula for the Russell 2000's characteristic line? b. You forecast a market return of 6% next year. According to the market model, what is next year's expected return for the Russell 2000 index? c. What is the correlation between the return on the Russell 2000 and the return on the market Index? d. How much of the variation in the Russell 2000's returns are explained by the model? e. Based on these regression results, the Russell 2000 index would be considered what kind of an investment, defensive, aggressive, or market neutral? f. Does this regression have much explanatory power? Why or why not?arrow_forwardDescribe, in detail; how you can best fix the problem (provide pluses and minuses associated with your supplied problem solution)? The phase of recession is the phase of business cycle in which the economic activities experience the downward trend. The demand in the economy falls , business investment decreases because of decreased scope of sales and unemployment rises leading to fall in GDP. The root cause inside the case is the declining sales and productivity of Moto's Integrated Controls Division (ICD) because of a drop in worldwide demand for machine devices prompted by the recession and savage rivalry. The ICD detailed a 5% downfall in sales in 1982 and again in the initial a half year of 1983, which put the future of the business in uncertainty. To keep the business running, the company needed to justify spending $3 million which it had done on various kinds of technological improvements and innovations , and there were questions about whether the investment could be…arrow_forwardDemand for Orange Juice is given as Qd = 5000-2500 P + 1200 I +650E - 255 PS Suppose Income is I = Rs.500, Expectations E = 55, and Price of Ps= Rs 25. Find the Demand Equation. b. Using the demand function from part a., Calculate Elasticity of Demand for price range of Rs.125 and Rs. 155. What will be the 'Price Elasticity of Demand at P = Rs.125? d Interpret the Elasticity of Demand calculated in (C) above.arrow_forward
arrow_back_ios
SEE MORE QUESTIONS
arrow_forward_ios
Recommended textbooks for you
- Managerial Economics: Applications, Strategies an...EconomicsISBN:9781305506381Author:James R. McGuigan, R. Charles Moyer, Frederick H.deB. HarrisPublisher:Cengage Learning
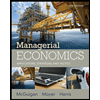
Managerial Economics: Applications, Strategies an...
Economics
ISBN:9781305506381
Author:James R. McGuigan, R. Charles Moyer, Frederick H.deB. Harris
Publisher:Cengage Learning