Number of dogs encounters per day, the number of customers entering a store per hour, the number of bacteria per liter of water, etc. are classically modelled using the Poisson distribution. With an exponential distribution, on the other hand, is used for modelling intervals of between events and how long a light bulb lasts, for example. After learning this, person P decides to model the observations in such a way that the corresponding random variables Y₁,...,Y for a random sample that is, n independent observation, of the exponential distribution Exp >0. The parameter > 0 is used as the statistical model parameter 1 which is the expected value of each observation random variable Y₁ a.) Derive the joint density function f(y; μ) specifying the model. b.) Derive the likelihood function L (μ) and the log likelihood function 1 (μ) c.) Define the maximum likelihood estimate ₁ (y) in the model for the parameter μ and form the maximum likelihood estimator (Y) in the model for the parameter μ. d.) Assume that n = 6 and the observed data (i.e., six dog encounter intervals (min)) is y = (Y1, Y2, ..., 35, 36) = (2.25, 8.40, 5.10, 11.85, 3.30, 8.10) .Use the data to calculate the ML estimate for the expected value μ of the intervals. Also consider what can be deduced from this: On the basis on this analysis of problem, do you think it is possible, i.e. likely, that expected value would actually be 3 (minutes). e.) Calculate the expected value EμM (Y) of the ML estimator ^ (Y) Tell using this (with justification) is the ML estimator unbiased? (Use the definition of unbiased estimator) f.) Calculate the variance varμ₁ (Y) of the ML estimator (Y)
Number of dogs encounters per day, the number of customers entering a store per hour, the number of bacteria per liter of water, etc. are classically modelled using the Poisson distribution. With an exponential distribution, on the other hand, is used for modelling intervals of between events and how long a light bulb lasts, for example. After learning this, person P decides to model the observations in such a way that the corresponding random variables Y₁,...,Y for a random sample that is, n independent observation, of the exponential distribution Exp >0. The parameter > 0 is used as the statistical model parameter 1 which is the expected value of each observation random variable Y₁ a.) Derive the joint density function f(y; μ) specifying the model. b.) Derive the likelihood function L (μ) and the log likelihood function 1 (μ) c.) Define the maximum likelihood estimate ₁ (y) in the model for the parameter μ and form the maximum likelihood estimator (Y) in the model for the parameter μ. d.) Assume that n = 6 and the observed data (i.e., six dog encounter intervals (min)) is y = (Y1, Y2, ..., 35, 36) = (2.25, 8.40, 5.10, 11.85, 3.30, 8.10) .Use the data to calculate the ML estimate for the expected value μ of the intervals. Also consider what can be deduced from this: On the basis on this analysis of problem, do you think it is possible, i.e. likely, that expected value would actually be 3 (minutes). e.) Calculate the expected value EμM (Y) of the ML estimator ^ (Y) Tell using this (with justification) is the ML estimator unbiased? (Use the definition of unbiased estimator) f.) Calculate the variance varμ₁ (Y) of the ML estimator (Y)
Holt Mcdougal Larson Pre-algebra: Student Edition 2012
1st Edition
ISBN:9780547587776
Author:HOLT MCDOUGAL
Publisher:HOLT MCDOUGAL
Chapter11: Data Analysis And Probability
Section: Chapter Questions
Problem 8CR
Related questions
Question
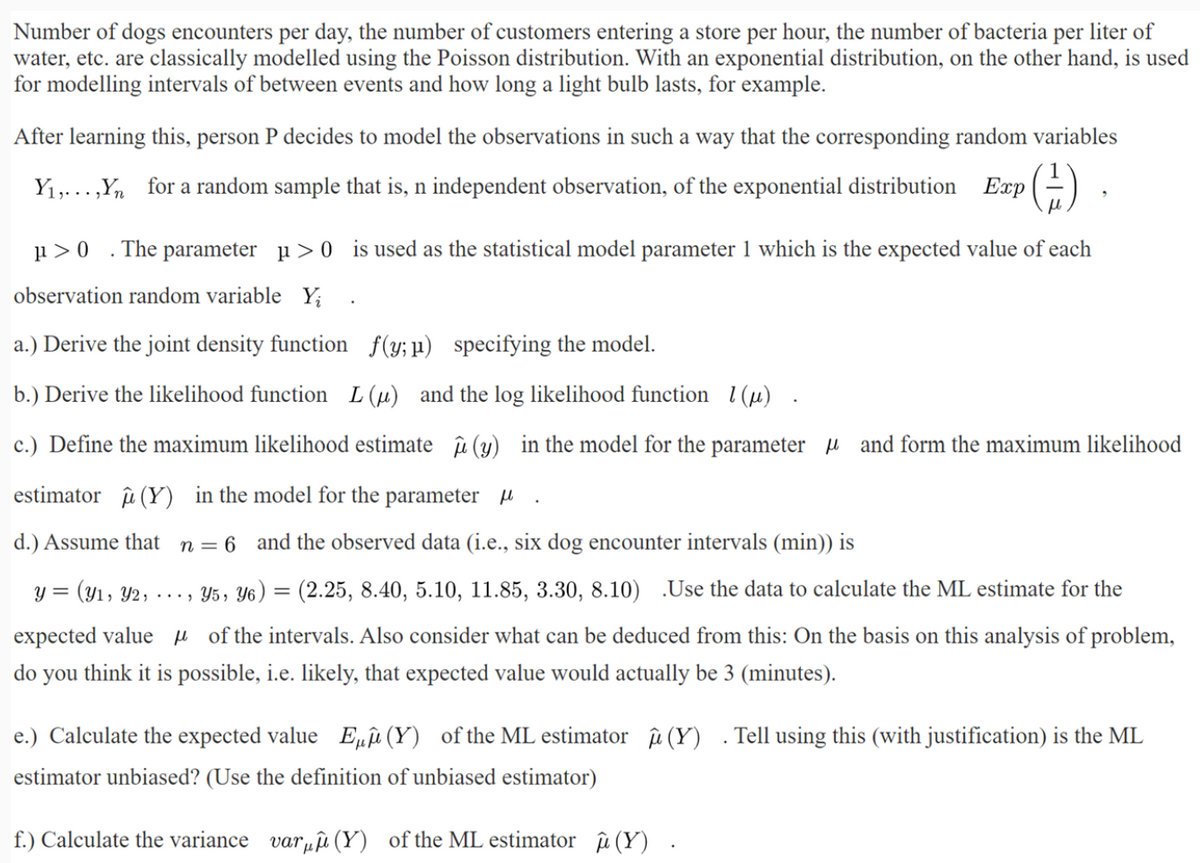
Transcribed Image Text:Number of dogs encounters per day, the number of customers entering a store per hour, the number of bacteria per liter of
water, etc. are classically modelled using the Poisson distribution. With an exponential distribution, on the other hand, is used
for modelling intervals of between events and how long a light bulb lasts, for example.
After learning this, person P decides to model the observations in such a way that the corresponding random variables
Y₁....,Y, for a random sample that is, n independent observation, of the exponential distribution Exp (H)
μ > O
The parameter μ>0 is used as the statistical model parameter 1 which is the expected value of each
observation random variable Y₁.
a.) Derive the joint density function f(y; μ) specifying the model.
b.) Derive the likelihood function L (μ) and the log likelihood function 1(µ) .
c.) Define the maximum likelihood estimate ₁ (y) in the model for the parameter μ and form the maximum likelihood
estimator (Y) in the model for the parameter μ.
d.) Assume that n = 6 and the observed data (i.e., six dog encounter intervals (min)) is
y = (y1, Y2,
=
Y5, 96) (2.25, 8.40, 5.10, 11.85, 3.30, 8.10) .Use the data to calculate the ML estimate for the
expected value μ of the intervals. Also consider what can be deduced from this: On the basis on this analysis of problem,
you think it is possible, i.e. likely, that expected value would actually be 3 (minutes).
do
e.) Calculate the expected value E₁₁ (Y) of the ML estimator ^ (Y) . Tell using this (with justification) is the ML
estimator unbiased? (Use the definition of unbiased estimator)
f.) Calculate the variance varμ^ (Y) of the ML estimator ^ (Y) .
Expert Solution

This question has been solved!
Explore an expertly crafted, step-by-step solution for a thorough understanding of key concepts.
Step 1: Write the given information
VIEWStep 2: Derive the joint density function f(y; μ), the likelihood function and the log likelihood function
VIEWStep 3: Define the maximum likelihood estimate μ^(y)
VIEWStep 4: Calculate the ML estimate for the expected value μ of the intervals using the data
VIEWStep 5: Calculate the expected value and the variance of the ML estimator μ^(Y)
VIEWSolution
VIEWStep by step
Solved in 6 steps with 33 images

Recommended textbooks for you
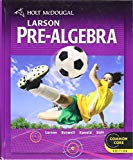
Holt Mcdougal Larson Pre-algebra: Student Edition…
Algebra
ISBN:
9780547587776
Author:
HOLT MCDOUGAL
Publisher:
HOLT MCDOUGAL
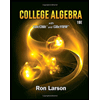
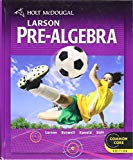
Holt Mcdougal Larson Pre-algebra: Student Edition…
Algebra
ISBN:
9780547587776
Author:
HOLT MCDOUGAL
Publisher:
HOLT MCDOUGAL
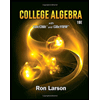