4, ii. a probability distribution function m(x) for a discrete random variable X such that a discrete random variable X with this distribution function will have μ = 0, o = 1, and satisfy P(|X| ≥ 2) = 1
4, ii. a probability distribution function m(x) for a discrete random variable X such that a discrete random variable X with this distribution function will have μ = 0, o = 1, and satisfy P(|X| ≥ 2) = 1
A First Course in Probability (10th Edition)
10th Edition
ISBN:9780134753119
Author:Sheldon Ross
Publisher:Sheldon Ross
Chapter1: Combinatorial Analysis
Section: Chapter Questions
Problem 1.1P: a. How many different 7-place license plates are possible if the first 2 places are for letters and...
Related questions
Question
give solution for 3. part (ii)
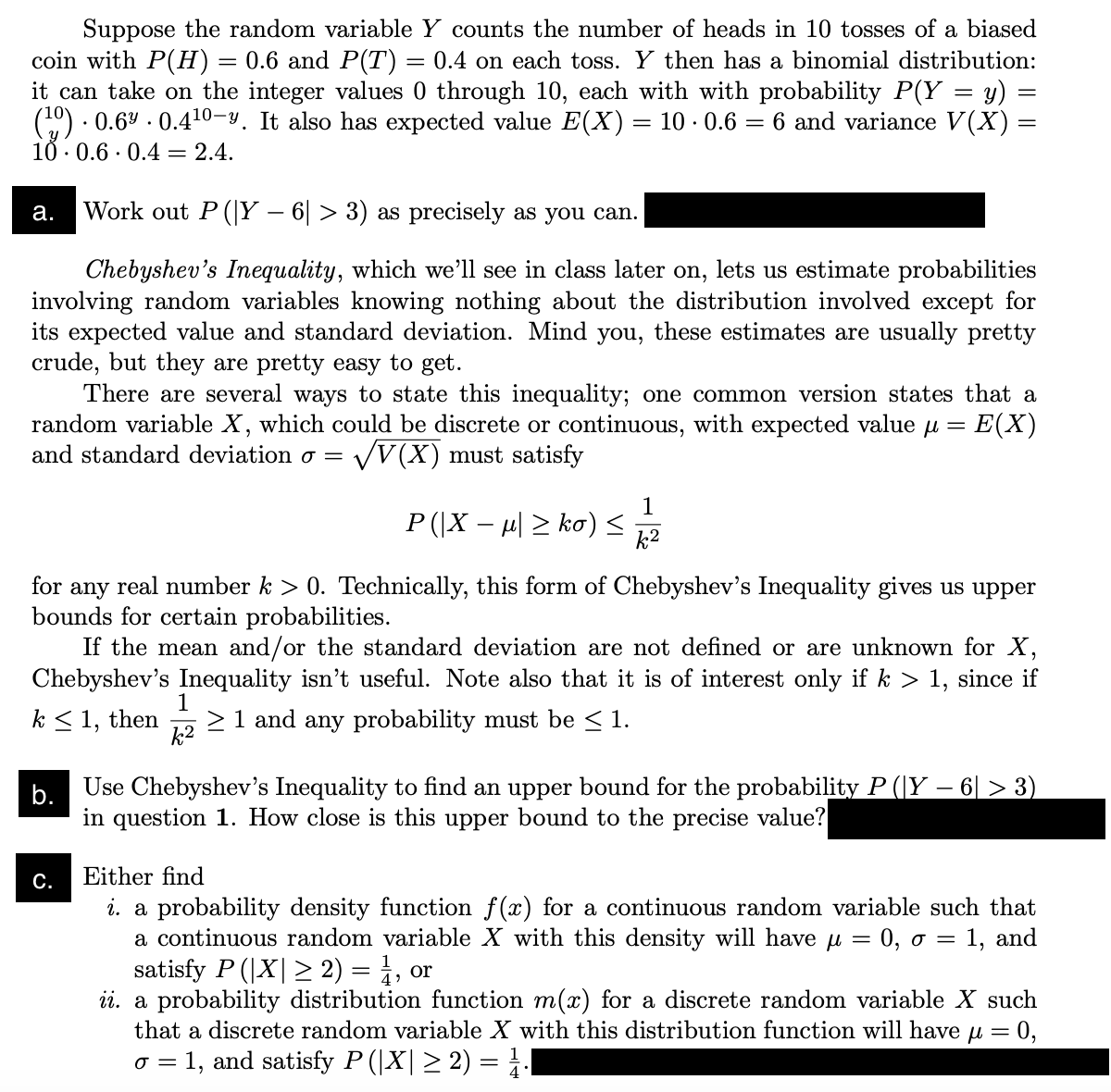
Transcribed Image Text:Suppose the random variable Y counts the number of heads in 10 tosses of a biased
coin with P(H) = 0.6 and P(T) = 0.4 on each toss. Y then has a binomial distribution:
it can take on the integer values 0 through 10, each with with probability P(Y = y)
10 0.66 and variance V(X) =
1.
(¹0) 0.6 0.4¹0-y. It also has expected value E(X)
10.0.6 0.4 2.4.
.
=
a. Work out P (Y− 6| > 3) as precisely as you can.
Chebyshev's Inequality, which we'll see in class later on, lets us estimate probabilities
involving random variables knowing nothing about the distribution involved except for
its expected value and standard deviation. Mind you, these estimates are usually pretty
crude, but they are pretty easy to get.
There are several ways to state this inequality; one common version states that a
random variable X, which could be discrete or continuous, with expected value μ = : E(X)
and standard deviation σ = √V(X) must satisfy
P(|X − µ| ≥ ko) ≤
C.
=
1
k²
for any real number k > 0. Technically, this form of Chebyshev's Inequality gives us upper
bounds for certain probabilities.
If the mean and/or the standard deviation are not defined or are unknown for X,
Chebyshev's Inequality isn't useful. Note also that it is of interest only if k > 1, since if
1
k≤ 1, then ≥ 1 and any probability must be ≤ 1.
k²
b.
Use Chebyshev's Inequality to find an upper bound for the probability P (|Y − 6| > 3)
in question 1. How close is this upper bound to the precise value?
Either find
i. a probability density function f(x) for a continuous random variable such that
=
= 0, o = 1, and
a continuous random variable X with this density will have μ
satisfy P (|X| ≥ 2) = ½, or
ii. a probability distribution function m(x) for a discrete random variable X such
that a discrete random variable X with this distribution function will have μ = 0,
= 1, and satisfy P(|X| ≥ 2) = ¹.
0 =
Expert Solution

This question has been solved!
Explore an expertly crafted, step-by-step solution for a thorough understanding of key concepts.
Step by step
Solved in 4 steps with 7 images

Recommended textbooks for you
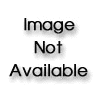
A First Course in Probability (10th Edition)
Probability
ISBN:
9780134753119
Author:
Sheldon Ross
Publisher:
PEARSON
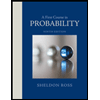
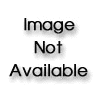
A First Course in Probability (10th Edition)
Probability
ISBN:
9780134753119
Author:
Sheldon Ross
Publisher:
PEARSON
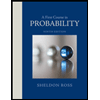