What is Advantages Disadvantages Applications Bayesian Dimensionality reduction Instances based Clustering Regularization Neural network Ensemble Deep learnine Decision Tree Rules svstem
What is Advantages Disadvantages Applications Bayesian Dimensionality reduction Instances based Clustering Regularization Neural network Ensemble Deep learnine Decision Tree Rules svstem
Computer Networking: A Top-Down Approach (7th Edition)
7th Edition
ISBN:9780133594140
Author:James Kurose, Keith Ross
Publisher:James Kurose, Keith Ross
Chapter1: Computer Networks And The Internet
Section: Chapter Questions
Problem R1RQ: What is the difference between a host and an end system? List several different types of end...
Related questions
Question
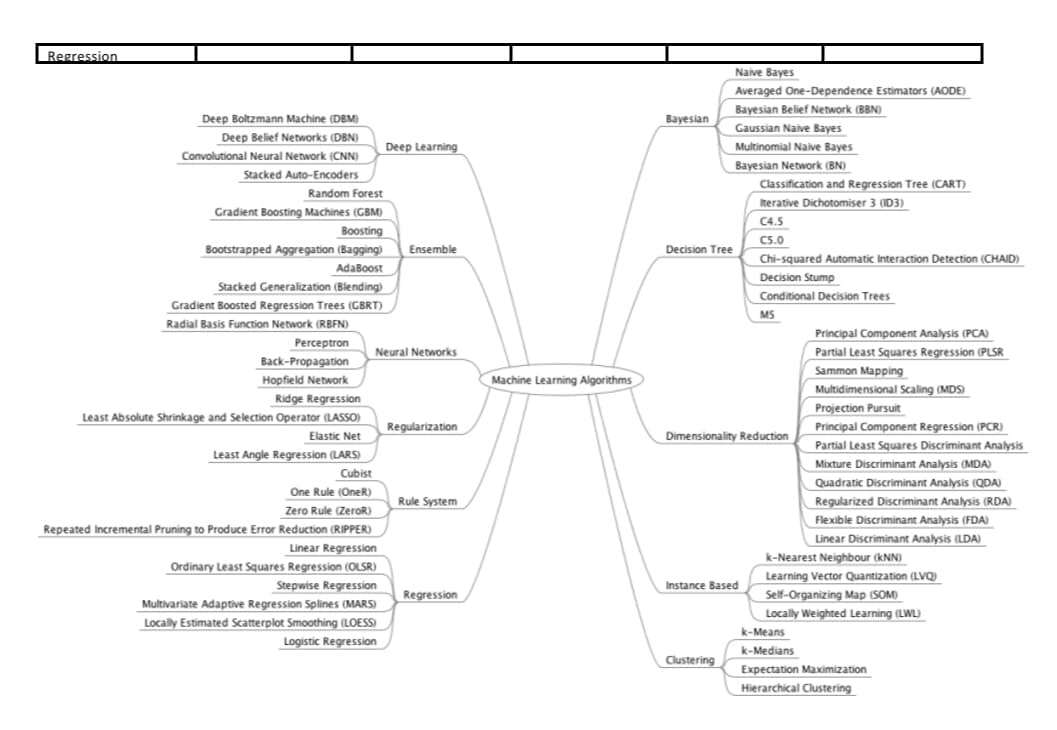
Transcribed Image Text:Regression
Naive Bayes
Averaged One-Dependence Estimators (AODE)
Bayesian Belief Network (BBN)
Deep Boltzmann Machine (DBM)
Bayesian
Gaussian Nalve Bayes
Deep Belief Networks (DBN)
Deep Learning
Multinomial Naive Bayes
Bayesian Network (BN)
Classification and Regression Tree (CART)
Convolutional Neural Network (CNN)
Stacked Auto-Encoders
Random Forest
Iterative Dichotomiser 3 (ID3)
Gradient Boosting Machines (GBM)
Boosting
Bootstrapped Aggregation (Bagging)
C4.5
C5.0
Ensemble
Decision Tree
Chi-squared Automatic Interaction Detection (CHAID)
AdaBoost
Stacked Generalization (Blending)
Decision Stump
Conditional Decision Trees
Gradient Boosted Regression Trees (GBRT)
MS
Radial Basis Function Network (RBFN)
Principal Component Analysis (PCA)
Perceptron
Neural Networks
Partial Least Squares Regression (PLSR
Back-Propagation
Sammon Mapping
Machine Learning Algorithms
Hopfield Network
Ridge Regression
Least Absolute Shrinkage and Selection Operator (LASSO)
Multidimensional Scaling (MDS)
Projection Pursuit
Regularization
Principal Component Regression (PCR)
Elastic Net
Dimensionality Reduction
Partial Least Squares Discriminant Analysis
Least Angle Regression (LARS)
Mixture Discriminant Analysis (MDA)
Cubist
One Rule (OneR)
Zero Rule (ZeroR)
Quadratic Discriminant Analysis (QDA)
Rule System
Regularized Discriminant Analysis (RDA)
Flexible Discriminant Analysis (FDA)
Repeated Incremental Pruning to Produce Error Reduction (RIPPER)
Linear Discriminant Analysis (LDA)
Linear Regression
k-Nearest Neighbour (kNN)
Ordinary Least Squares Regression (OLSR)
Learning Vector Quantization (LVQ)
Stepwise Regression
Instance Based
Regression
Self-Organizing Map (SOM)
Multivariate Adaptive Regression Splines (MARS)
Locally Weighted Learning (LWL)
Locally Estimated Scatterplot Smoothing (LOESS)
k-Means
Logistic Regression
k-Medians
Clustering
Expectation Maximization
Hierarchical Clustering
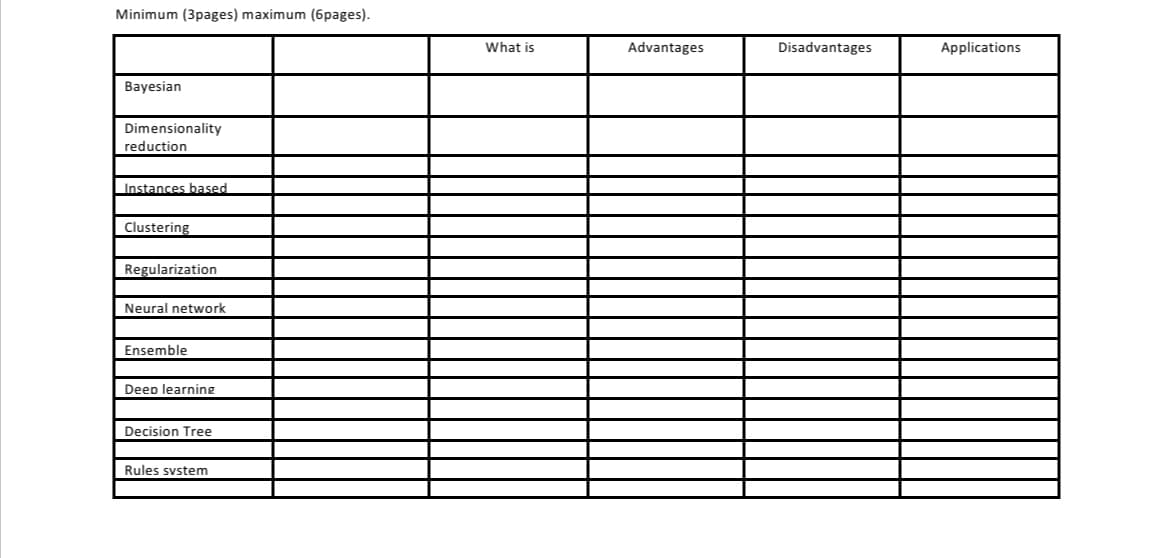
Transcribed Image Text:Minimum (3pages) maximum (6pages).
What is
Advantages
Disadvantages
Applications
Bayesian
Dimensionality
reduction
|Instances based
Clustering
Regularization
Neural network
Ensemble
Deep learning
Decision Tree
Rules svstem
Expert Solution

This question has been solved!
Explore an expertly crafted, step-by-step solution for a thorough understanding of key concepts.
Step by step
Solved in 2 steps

Recommended textbooks for you
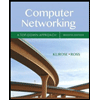
Computer Networking: A Top-Down Approach (7th Edi…
Computer Engineering
ISBN:
9780133594140
Author:
James Kurose, Keith Ross
Publisher:
PEARSON
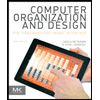
Computer Organization and Design MIPS Edition, Fi…
Computer Engineering
ISBN:
9780124077263
Author:
David A. Patterson, John L. Hennessy
Publisher:
Elsevier Science
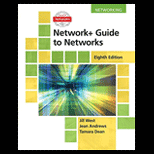
Network+ Guide to Networks (MindTap Course List)
Computer Engineering
ISBN:
9781337569330
Author:
Jill West, Tamara Dean, Jean Andrews
Publisher:
Cengage Learning
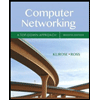
Computer Networking: A Top-Down Approach (7th Edi…
Computer Engineering
ISBN:
9780133594140
Author:
James Kurose, Keith Ross
Publisher:
PEARSON
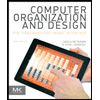
Computer Organization and Design MIPS Edition, Fi…
Computer Engineering
ISBN:
9780124077263
Author:
David A. Patterson, John L. Hennessy
Publisher:
Elsevier Science
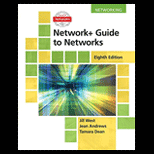
Network+ Guide to Networks (MindTap Course List)
Computer Engineering
ISBN:
9781337569330
Author:
Jill West, Tamara Dean, Jean Andrews
Publisher:
Cengage Learning
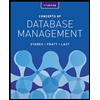
Concepts of Database Management
Computer Engineering
ISBN:
9781337093422
Author:
Joy L. Starks, Philip J. Pratt, Mary Z. Last
Publisher:
Cengage Learning
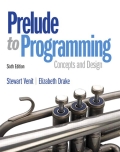
Prelude to Programming
Computer Engineering
ISBN:
9780133750423
Author:
VENIT, Stewart
Publisher:
Pearson Education
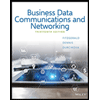
Sc Business Data Communications and Networking, T…
Computer Engineering
ISBN:
9781119368830
Author:
FITZGERALD
Publisher:
WILEY