1. (Proximal Gradient Descent) In this problem, we will show the sublinear convergence for proximal gradient descent. To be precise, we assume that the objective f(x) can be written as f(x) = g(x) + h(x), where (a) g is convex, differentiable, and dom(g) € Rd. (b) Vg is Lipschitz, with constant L > 0. (c) h is convex, not necessarily differentiable, and we take dom(h) = Rd for simplicity. By defining the generalized gradient to be: G(x) = L(xk – X+1), where xk+1 is next iterate obtained from applying PGD to xk. Show that f(xk+1) - f(x*) ≤ ( |x − x*||² — ||×xk+1 − x*||²), where x* is the minimizer of f, and use it to conclude L f(xk) - f(x*) ≤ xo-x*||². 2k That is, the proximal descent method achieves O(1/k) accuracy at the k-th iteration. Hint: You can freely use the following lemma, which shows that the PGD is also a "descent method": Lemma 1 (Proximal Descent Lemma). 1 f(xk+1) − f(z) ≤ G(xk)¯(xk — z) - - ||G(x)||2, VzЄR". 2L
1. (Proximal Gradient Descent) In this problem, we will show the sublinear convergence for proximal gradient descent. To be precise, we assume that the objective f(x) can be written as f(x) = g(x) + h(x), where (a) g is convex, differentiable, and dom(g) € Rd. (b) Vg is Lipschitz, with constant L > 0. (c) h is convex, not necessarily differentiable, and we take dom(h) = Rd for simplicity. By defining the generalized gradient to be: G(x) = L(xk – X+1), where xk+1 is next iterate obtained from applying PGD to xk. Show that f(xk+1) - f(x*) ≤ ( |x − x*||² — ||×xk+1 − x*||²), where x* is the minimizer of f, and use it to conclude L f(xk) - f(x*) ≤ xo-x*||². 2k That is, the proximal descent method achieves O(1/k) accuracy at the k-th iteration. Hint: You can freely use the following lemma, which shows that the PGD is also a "descent method": Lemma 1 (Proximal Descent Lemma). 1 f(xk+1) − f(z) ≤ G(xk)¯(xk — z) - - ||G(x)||2, VzЄR". 2L
Algebra & Trigonometry with Analytic Geometry
13th Edition
ISBN:9781133382119
Author:Swokowski
Publisher:Swokowski
Chapter7: Analytic Trigonometry
Section7.6: The Inverse Trigonometric Functions
Problem 91E
Related questions
Question

Transcribed Image Text:1. (Proximal Gradient Descent) In this problem, we will show the sublinear convergence for proximal gradient descent.
To be precise, we assume that the objective f(x) can be written as f(x) = g(x) + h(x), where
(a) g is convex, differentiable, and dom(g) € Rd.
(b) Vg is Lipschitz, with constant L > 0.
(c) h is convex, not necessarily differentiable, and we take dom(h) = Rd for simplicity.
By defining the generalized gradient to be:
G(x) = L(xk – X+1),
where xk+1 is next iterate obtained from applying PGD to xk. Show that
f(xk+1) - f(x*) ≤ ( |x − x*||² — ||×xk+1 − x*||²),
where x* is the minimizer of f, and use it to conclude
L
f(xk) - f(x*) ≤ xo-x*||².
2k
That is, the proximal descent method achieves O(1/k) accuracy at the k-th iteration.
Hint: You can freely use the following lemma, which shows that the PGD is also a "descent method":
Lemma 1 (Proximal Descent Lemma).
1
f(xk+1) − f(z) ≤ G(xk)¯(xk — z) -
-
||G(x)||2, VzЄR".
2L
Expert Solution

This question has been solved!
Explore an expertly crafted, step-by-step solution for a thorough understanding of key concepts.
Step by step
Solved in 2 steps with 1 images

Recommended textbooks for you
Algebra & Trigonometry with Analytic Geometry
Algebra
ISBN:
9781133382119
Author:
Swokowski
Publisher:
Cengage
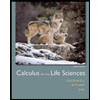
Calculus For The Life Sciences
Calculus
ISBN:
9780321964038
Author:
GREENWELL, Raymond N., RITCHEY, Nathan P., Lial, Margaret L.
Publisher:
Pearson Addison Wesley,
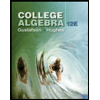
College Algebra (MindTap Course List)
Algebra
ISBN:
9781305652231
Author:
R. David Gustafson, Jeff Hughes
Publisher:
Cengage Learning
Algebra & Trigonometry with Analytic Geometry
Algebra
ISBN:
9781133382119
Author:
Swokowski
Publisher:
Cengage
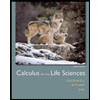
Calculus For The Life Sciences
Calculus
ISBN:
9780321964038
Author:
GREENWELL, Raymond N., RITCHEY, Nathan P., Lial, Margaret L.
Publisher:
Pearson Addison Wesley,
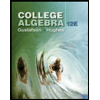
College Algebra (MindTap Course List)
Algebra
ISBN:
9781305652231
Author:
R. David Gustafson, Jeff Hughes
Publisher:
Cengage Learning
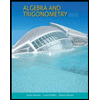
Algebra and Trigonometry (MindTap Course List)
Algebra
ISBN:
9781305071742
Author:
James Stewart, Lothar Redlin, Saleem Watson
Publisher:
Cengage Learning