Consider a binary classification problem using 1-nearest neighbors with the Euclidean dis- and corresponding labels M are in ascend- 6. tance metric. We have N 1-dimensional training points x", . ya, a.. with xl ER and y" e 0. 1. Assume the points x. . ing order by vahue. If there are ties during the 1-NN algorithm, we break ties by choosing the label corresponding to the x" with lower value. Is it possible to build a decision tree that behaves exactly the same as the 1-nearest neighbor classifier? Assume that the decision at each node takes the form of" x st or x >1," wheret eR. O Yes O No (a) If your answer is yes, please explain how you will construct the decision tree. If your answer is no, explain why it's not possible. Your answer: Decision Trees, k-NN, Regression () where x" = k, ) eR and the decision at each node takes the form of" xy stor x) > t," where t eR and / E (1. 2. Give an example with at most 3 training points for which it isn't possible to build a decision tree that behaves exactly the same as a l-nearest neighbor classifier. Let's add a dimension! Now assume the training points are 2-dimensional Your answer:
Consider a binary classification problem using 1-nearest neighbors with the Euclidean dis- and corresponding labels M are in ascend- 6. tance metric. We have N 1-dimensional training points x", . ya, a.. with xl ER and y" e 0. 1. Assume the points x. . ing order by vahue. If there are ties during the 1-NN algorithm, we break ties by choosing the label corresponding to the x" with lower value. Is it possible to build a decision tree that behaves exactly the same as the 1-nearest neighbor classifier? Assume that the decision at each node takes the form of" x st or x >1," wheret eR. O Yes O No (a) If your answer is yes, please explain how you will construct the decision tree. If your answer is no, explain why it's not possible. Your answer: Decision Trees, k-NN, Regression () where x" = k, ) eR and the decision at each node takes the form of" xy stor x) > t," where t eR and / E (1. 2. Give an example with at most 3 training points for which it isn't possible to build a decision tree that behaves exactly the same as a l-nearest neighbor classifier. Let's add a dimension! Now assume the training points are 2-dimensional Your answer:
Computer Networking: A Top-Down Approach (7th Edition)
7th Edition
ISBN:9780133594140
Author:James Kurose, Keith Ross
Publisher:James Kurose, Keith Ross
Chapter1: Computer Networks And The Internet
Section: Chapter Questions
Problem R1RQ: What is the difference between a host and an end system? List several different types of end...
Related questions
Question
6. Consider a binary classification problem using 1-nearest neighbors with the Euclidean distance metric. We have N 1-dimensional training points x(1), x(2), . . . x(N ) and corresponding labels
y(1), y(2), . . . y(N ) with x(i ) ∈ R and y(i ) ∈ {0, 1}. Assume the points x(1), x(2), . . . x(N ) are in ascending order by value. If there are ties during the 1-NN
corresponding to the x(i ) with lower value.
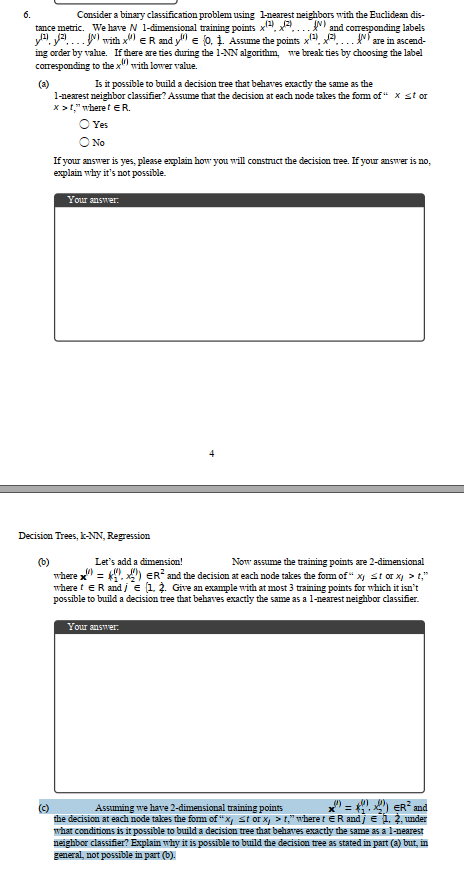
Transcribed Image Text:6.
Consider a binary classification problem using l-nearest neighbors with the Euclidean dis-
tance metric. We have N 1-dimensional training points x", x".... and corresponding labels
are in ascend-
ing order by vahue. If there are ties during the 1-NN algorithm, we break ties by choosing the label
y". P..M with x ER and y" e (0. 1. Assume the points x. x.
coresponding to the x" with lower value.
Is it possible to build a decision tree that behaves exactly the same as the
1-nearest neighbor classifier? Assume that the decision at each node takes the fom of" x st or
x >1," wheret ER.
(a)
Yes
O No
If your answer is yes, please explain how you will construct the decision tree. If your answer is no,
explain why it's not possible.
Your answer.
Decision Trees, k-NN, Regression
Now assume the training points are 2-dimensional
where x" = . ) ER? and the decision at each node takes the form of “ xy st or x, > t,"
where t eR andj e (1. 2. Give an example with at most 3 training points for which it isn't
possible to build a decision tree that behaves exactly the same as a l-nearest neighbor classifier.
Let's add a dimension!
Your answer:
= . ) ER* and
(c)
the decision at each node takes the form of "x, st or x, > 1," wheret ER and j e 1, 2, under
what conditions is it possible to buzild a decision tree that behaves exactly the same as a l-nearest
neighbor classifier? Explain why it is possible to build the decision tree as stated in part (a) but, in
general, not possible in part (6).
Assuming we have 2-dimensional training points
Expert Solution

This question has been solved!
Explore an expertly crafted, step-by-step solution for a thorough understanding of key concepts.
This is a popular solution!
Trending now
This is a popular solution!
Step by step
Solved in 2 steps

Recommended textbooks for you
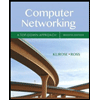
Computer Networking: A Top-Down Approach (7th Edi…
Computer Engineering
ISBN:
9780133594140
Author:
James Kurose, Keith Ross
Publisher:
PEARSON
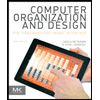
Computer Organization and Design MIPS Edition, Fi…
Computer Engineering
ISBN:
9780124077263
Author:
David A. Patterson, John L. Hennessy
Publisher:
Elsevier Science
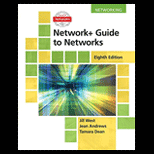
Network+ Guide to Networks (MindTap Course List)
Computer Engineering
ISBN:
9781337569330
Author:
Jill West, Tamara Dean, Jean Andrews
Publisher:
Cengage Learning
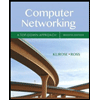
Computer Networking: A Top-Down Approach (7th Edi…
Computer Engineering
ISBN:
9780133594140
Author:
James Kurose, Keith Ross
Publisher:
PEARSON
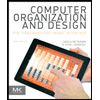
Computer Organization and Design MIPS Edition, Fi…
Computer Engineering
ISBN:
9780124077263
Author:
David A. Patterson, John L. Hennessy
Publisher:
Elsevier Science
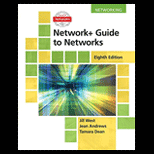
Network+ Guide to Networks (MindTap Course List)
Computer Engineering
ISBN:
9781337569330
Author:
Jill West, Tamara Dean, Jean Andrews
Publisher:
Cengage Learning
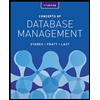
Concepts of Database Management
Computer Engineering
ISBN:
9781337093422
Author:
Joy L. Starks, Philip J. Pratt, Mary Z. Last
Publisher:
Cengage Learning
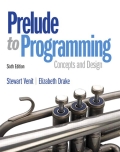
Prelude to Programming
Computer Engineering
ISBN:
9780133750423
Author:
VENIT, Stewart
Publisher:
Pearson Education
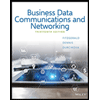
Sc Business Data Communications and Networking, T…
Computer Engineering
ISBN:
9781119368830
Author:
FITZGERALD
Publisher:
WILEY