1 Consider the dataset shown in Table 1 for a binary classification problem. Table 1: Dataset with 20 instances 1.1 MovielD Rental Days Format 1 DVD 2 DVD 3 DVD 4 DVD 5 DVD 6 DVD 7 Online 8 Online Online 1 10 3 6 9 3 1 10911 91012 13 14 15 16 17 18 19 20 10 11 8 6 2253WAW79 2 3 4 3 2 1 5 3 Category Entertainment Online DVD Class Days-equal-depth 0 0 0 0 Comedy Documentary Comedy Comedy Documentary Comedy 0 Comedy 0 Comedy 0 Documentary 0 Comedy 0 Entertainment 1 DVD Online Entertainment 1 Online Documentary 1 Documentary 1 DVD Online Documentary Online Documentary 1 Online Entertainment Online Documentary Online Documentary 1 Discretize the attribute 'Rental Days' to transform it into a categorical attribute with 4 attribute values, a1, a2, a3, a4 (i.e., number of bins = 4) and fill out the last column. Use the equal-depth approach for discretization. 1.1 Compute the Entropy, Gini, and Misclassification Error for the overall collection of training examples. (These will be the impurity measures on the parent node.) 1.3 Compute the combined Entropy, Gini, Misclassification Error of the children nodes for all the three attributes: Rental Days, Format, and Movie Category, using multi-way splits for Rental Days and Movie
1 Consider the dataset shown in Table 1 for a binary classification problem. Table 1: Dataset with 20 instances 1.1 MovielD Rental Days Format 1 DVD 2 DVD 3 DVD 4 DVD 5 DVD 6 DVD 7 Online 8 Online Online 1 10 3 6 9 3 1 10911 91012 13 14 15 16 17 18 19 20 10 11 8 6 2253WAW79 2 3 4 3 2 1 5 3 Category Entertainment Online DVD Class Days-equal-depth 0 0 0 0 Comedy Documentary Comedy Comedy Documentary Comedy 0 Comedy 0 Comedy 0 Documentary 0 Comedy 0 Entertainment 1 DVD Online Entertainment 1 Online Documentary 1 Documentary 1 DVD Online Documentary Online Documentary 1 Online Entertainment Online Documentary Online Documentary 1 Discretize the attribute 'Rental Days' to transform it into a categorical attribute with 4 attribute values, a1, a2, a3, a4 (i.e., number of bins = 4) and fill out the last column. Use the equal-depth approach for discretization. 1.1 Compute the Entropy, Gini, and Misclassification Error for the overall collection of training examples. (These will be the impurity measures on the parent node.) 1.3 Compute the combined Entropy, Gini, Misclassification Error of the children nodes for all the three attributes: Rental Days, Format, and Movie Category, using multi-way splits for Rental Days and Movie
Database System Concepts
7th Edition
ISBN:9780078022159
Author:Abraham Silberschatz Professor, Henry F. Korth, S. Sudarshan
Publisher:Abraham Silberschatz Professor, Henry F. Korth, S. Sudarshan
Chapter1: Introduction
Section: Chapter Questions
Problem 1PE
Related questions
Question
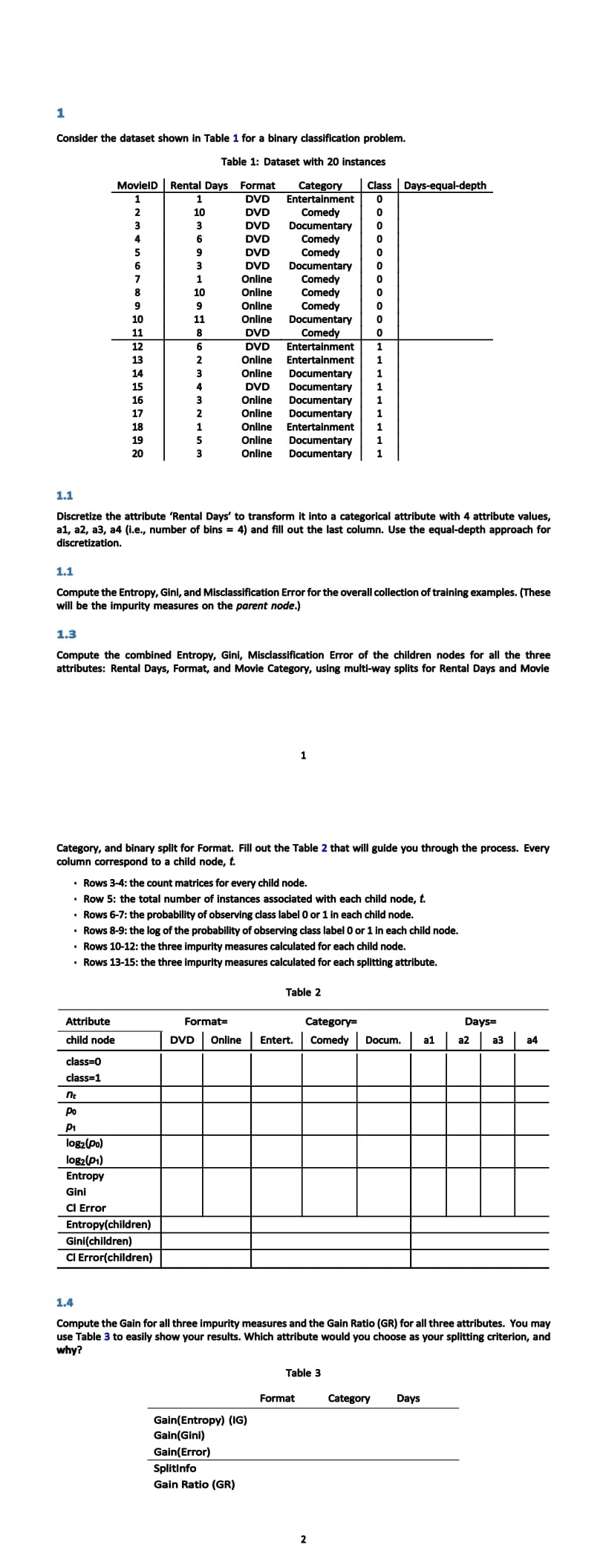
Transcribed Image Text:1
Consider the dataset shown in Table 1 for a binary classification problem.
Table 1: Dataset with 20 instances
1.1
MovielD Rental Days Format Category
DVD
Entertainment
DVD
Comedy
DVD
Documentary
DVD
Comedy
DVD
Comedy
DVD
Online
Online
Online
Online
10
1121314 15 16 17 18 19 20
1
10
3
6
Attribute
child node
class=0
class=1
9
3
1
10
9
11
8
nt
po
P₁
log₂ (po)
log₂ (P1)
Entropy
Gini
Cl Error
6
2
3
4
Entropy(children)
Gini(children)
CI Error(children)
1
5
3
DVD
DVD
Online
Online
DVD
Online
Online
Online
Online
Online
Documentary
Comedy
Comedy
Comedy
Documentary
Comedy
Format=
Discretize the attribute 'Rental Days' to transform it into a categorical attribute with 4 attribute values,
a1, a2, a3, a4 (i.e., number of bins = 4) and fill out the last column. Use the equal-depth approach for
discretization.
1.1
Compute the Entropy, Gini, and Misclassification Error for the overall collection of training examples. (These
will be the impurity measures on the parent node.)
1.3
Compute the combined Entropy, Gini, Misclassification Error of the children nodes for all the three
attributes: Rental Days, Format, and Movie Category, using multi-way splits for Rental Days and Movie
1
Gain(Entropy) (IG)
Gain(Gini)
Gain(Error)
Splitinfo
Gain Ratio (GR)
Class Days-equal-depth
0
0
0
Entertainment
1
Entertainment
1
Documentary
1
Documentary 1
Documentary 1
Documentary
Entertainment
1
1
Documentary
Documentary 1
Category, and binary split for Format. Fill out the Table 2 that will guide you through the process. Every
column correspond to a child node, t.
Category=
DVD Online Entert. Comedy
0
0
• Rows 3-4: the count matrices for every child node.
• Row 5: the total number of instances associated with each child node, t.
Table 2
0
• Rows 6-7: the probability of observing class label 0 or 1 in each child node.
• Rows 8-9: the log of the probability of observing class label 0 or 1 in each child node.
• Rows 10-12: the three impurity measures calculated for each child node.
• Rows 13-15: the three impurity measures calculated for each splitting attribute.
0
0
Table 3
Format
0
0
0
2
Docum.
a1
1.4
Compute the Gain for all three impurity measures and the Gain Ratio (GR) for all three attributes. You may
use Table 3 to easily show your results. Which attribute would you choose as your splitting criterion, and
why?
Category Days
Days=
a2
a3
a4
Expert Solution

This question has been solved!
Explore an expertly crafted, step-by-step solution for a thorough understanding of key concepts.
This is a popular solution!
Trending now
This is a popular solution!
Step by step
Solved in 4 steps

Follow-up Questions
Read through expert solutions to related follow-up questions below.
Follow-up Question
Can you provide solution for last part (1.4) here? If possible 1.1 again.
Solution
Knowledge Booster
Learn more about
Need a deep-dive on the concept behind this application? Look no further. Learn more about this topic, computer-science and related others by exploring similar questions and additional content below.Recommended textbooks for you
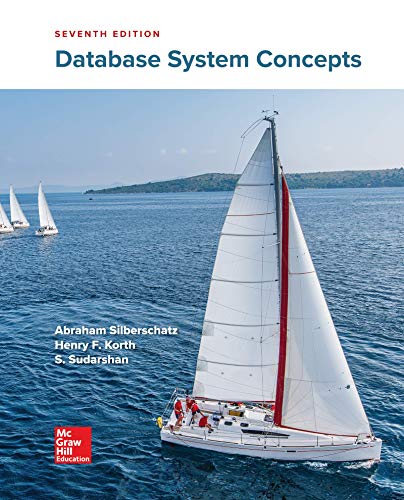
Database System Concepts
Computer Science
ISBN:
9780078022159
Author:
Abraham Silberschatz Professor, Henry F. Korth, S. Sudarshan
Publisher:
McGraw-Hill Education
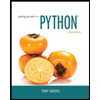
Starting Out with Python (4th Edition)
Computer Science
ISBN:
9780134444321
Author:
Tony Gaddis
Publisher:
PEARSON
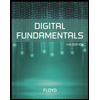
Digital Fundamentals (11th Edition)
Computer Science
ISBN:
9780132737968
Author:
Thomas L. Floyd
Publisher:
PEARSON
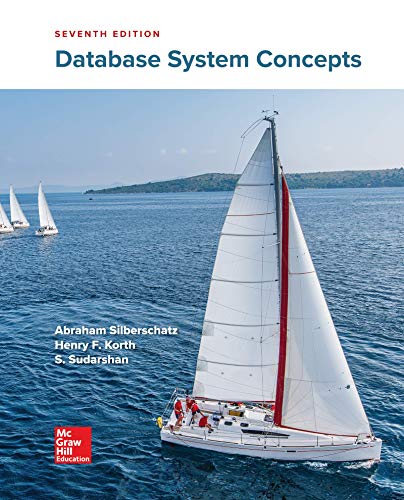
Database System Concepts
Computer Science
ISBN:
9780078022159
Author:
Abraham Silberschatz Professor, Henry F. Korth, S. Sudarshan
Publisher:
McGraw-Hill Education
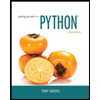
Starting Out with Python (4th Edition)
Computer Science
ISBN:
9780134444321
Author:
Tony Gaddis
Publisher:
PEARSON
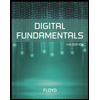
Digital Fundamentals (11th Edition)
Computer Science
ISBN:
9780132737968
Author:
Thomas L. Floyd
Publisher:
PEARSON
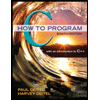
C How to Program (8th Edition)
Computer Science
ISBN:
9780133976892
Author:
Paul J. Deitel, Harvey Deitel
Publisher:
PEARSON
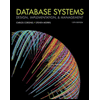
Database Systems: Design, Implementation, & Manag…
Computer Science
ISBN:
9781337627900
Author:
Carlos Coronel, Steven Morris
Publisher:
Cengage Learning
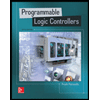
Programmable Logic Controllers
Computer Science
ISBN:
9780073373843
Author:
Frank D. Petruzella
Publisher:
McGraw-Hill Education