According to Stuart (2010), which of the following statements about observational study designs for causal inference are typically true? Choose all that apply. Including propensity score estimates as a predictor in a regression model on outcomes can help resolve imbalance between treatment and control groups for covariates used in the propensity score model Standard diagnostic tools for binary prediction or classification models (e.g. logistic regression or classification trees) are similarly used in evaluating propensity score estimates When conditional ignorability holds given the observed covariates, then the treatment assignment will also be ignorable conditioned on the propensity scores Overfitting in propensity score estimation can achieve more efficient estimates of treatment effects than using propensity score estimates that are closer to the true propensities Unlike full matching, subclassification, and weighting methods, nearest neighbor matching does not necessarily use all individuals within the area of overlap
According to Stuart (2010), which of the following statements about observational study designs for causal inference are typically true? Choose all that apply. Including propensity score estimates as a predictor in a regression model on outcomes can help resolve imbalance between treatment and control groups for covariates used in the propensity score model Standard diagnostic tools for binary prediction or classification models (e.g. logistic regression or classification trees) are similarly used in evaluating propensity score estimates When conditional ignorability holds given the observed covariates, then the treatment assignment will also be ignorable conditioned on the propensity scores Overfitting in propensity score estimation can achieve more efficient estimates of treatment effects than using propensity score estimates that are closer to the true propensities Unlike full matching, subclassification, and weighting methods, nearest neighbor matching does not necessarily use all individuals within the area of overlap
Glencoe Algebra 1, Student Edition, 9780079039897, 0079039898, 2018
18th Edition
ISBN:9780079039897
Author:Carter
Publisher:Carter
Chapter4: Equations Of Linear Functions
Section4.6: Regression And Median-fit Lines
Problem 4GP
Related questions
Question
3,
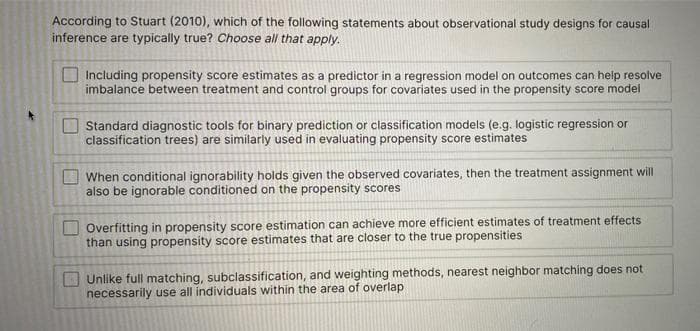
Transcribed Image Text:According to Stuart (2010), which of the following statements about observational study designs for causal
inference are typically true? Choose all that apply.
Including propensity score estimates as a predictor in a regression model on outcomes can help resolve
imbalance between treatment and control groups for covariates used in the propensity score model
Standard diagnostic tools for binary prediction or classification models (e.g. logistic regression or
classification trees) are similarly used in evaluating propensity score estimates
When conditional ignorability holds given the observed covariates, then the treatment assignment will
also be ignorable conditioned on the propensity scores
Overfitting in propensity score estimation can achieve more efficient estimates of treatment effects
than using propensity score estimates that are closer to the true propensities
Unlike full matching, subclassification, and weighting methods, nearest neighbor matching does not
necessarily use all individuals within the area of overlap
Expert Solution

This question has been solved!
Explore an expertly crafted, step-by-step solution for a thorough understanding of key concepts.
Step by step
Solved in 2 steps with 1 images

Recommended textbooks for you
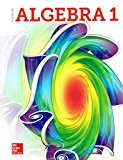
Glencoe Algebra 1, Student Edition, 9780079039897…
Algebra
ISBN:
9780079039897
Author:
Carter
Publisher:
McGraw Hill
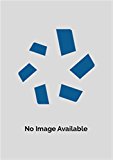
Big Ideas Math A Bridge To Success Algebra 1: Stu…
Algebra
ISBN:
9781680331141
Author:
HOUGHTON MIFFLIN HARCOURT
Publisher:
Houghton Mifflin Harcourt
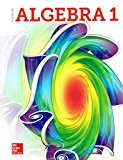
Glencoe Algebra 1, Student Edition, 9780079039897…
Algebra
ISBN:
9780079039897
Author:
Carter
Publisher:
McGraw Hill
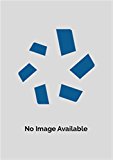
Big Ideas Math A Bridge To Success Algebra 1: Stu…
Algebra
ISBN:
9781680331141
Author:
HOUGHTON MIFFLIN HARCOURT
Publisher:
Houghton Mifflin Harcourt