o. Sometimes you can reduce multicollinearity by re-specifying the model, for instance, create a combination of multicollinear variables represents transforming the specification error. p. Inclusion of irrelevant variable(s) and error of measurement represents specification errors in a regression model.
o. Sometimes you can reduce multicollinearity by re-specifying the model, for instance, create a combination of multicollinear variables represents transforming the specification error. p. Inclusion of irrelevant variable(s) and error of measurement represents specification errors in a regression model.
Linear Algebra: A Modern Introduction
4th Edition
ISBN:9781285463247
Author:David Poole
Publisher:David Poole
Chapter3: Matrices
Section3.5: Subspaces, Basis, Dimension, And Rank
Problem 14EQ
Related questions
Question
Question and problem are on two different image files

Transcribed Image Text:o. Sometimes you can reduce multicollinearity by re-specifying the model, for instance,
create a combination of multicollinear variables represents transforming the specification
error.
p. Inclusion of irrelevant variable(s) and error of measurement represents specification
errors in a regression model.

Transcribed Image Text:State if the following is True or false and provide a brief explanation for your answer.
Consider Population modelY = Bo+ B1X1+ B2X2 + B3X3 +µ. Now consider the
following statements a to c. Assumption MLR 1– 4 is satisfied if and only if:
Expert Solution

This question has been solved!
Explore an expertly crafted, step-by-step solution for a thorough understanding of key concepts.
Step by step
Solved in 3 steps

Recommended textbooks for you
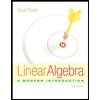
Linear Algebra: A Modern Introduction
Algebra
ISBN:
9781285463247
Author:
David Poole
Publisher:
Cengage Learning
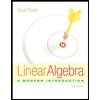
Linear Algebra: A Modern Introduction
Algebra
ISBN:
9781285463247
Author:
David Poole
Publisher:
Cengage Learning