PracticeProblems_FractionalFactorialDesigns
.pdf
keyboard_arrow_up
School
University of Michigan *
*We aren’t endorsed by this school
Course
503
Subject
Business
Date
Jan 9, 2024
Type
Pages
16
Uploaded by Dorothy.D
8.51.
A 16-run fractional factorial experiment in nine factors was conducted by Chrysler Motors Engineering and described in the article “Sheet Molded Compound Process Improvement,” by P.I. Hsieh and D.E. Goodwin (
Fourth
Symposium on Taguchi Methods
, American Supplier Institute, Dearborn, MI, 1986, pp. 13-21). The purpose was to reduce the number of defects in the finish of sheet-molded grill opening panels. The design, and the resulting number of defects, c
, observed on each run, is shown in Table P8.10. This is a resolution III fraction with generators E=BD, F=BCD, G=AC, H=ACD
, and J=AB.
Table P8.10
Run A B C D E F G H J c c
F&T’s Modification 1 - - - - + - + - + 56 7.48 7.52 2 + - - - + - - + - 17 4.12 4.18 3 - + - - - + + - - 2 1.41 1.57 4 + + - - - + - + + 4 2.00 2.12 5 - - + - + + - + + 3 1.73 1.87 6 + - + - + + + - - 4 2.00 2.12 7 - + + - - - - + - 50 7.07 7.12 8 + + + - - - + - + 2 1.41 1.57 9 - - - + - + + + + 1 1.00 1.21 10 + - - + - + - - - 0 0.00 0.50 11 - + - + + - + + - 3 1.73 1.87 12 + + - + + - - - + 12 3.46 3.54 13 - - + + - - - - + 3 1.73 1.87 14 + - + + - - + + - 4 2.00 2.12 15 - + + + + + - - - 0 0.00 0.50 16 + + + + + + + + + 0 0.00 0.50 (a)
Find the defining relation and the alias relationships in this design. I = ABJ = ACG = BDE = CEF = DGH = FHJ = ABFH = ACDH = ADEJ = AEFG = BCDF = BCGJ = BEGH = CEHJ = DFGJ = ABCEH = ABDFG = ACDFJ = ADEFH = AEGHJ = BCDHJ = BCFGH = BEFGJ = CDEGJ = ABCDEG = ABCEFJ = ABDGHJ = ACFGHJ = BDEFHJ = CDEFGH = ABCDEFGHJ (b)
Estimate the factor effects and use a normal probability plot to tentatively identify the important factors. Residual
Normal % probability
Normal plot of residuals
-0.189813
-0.106313
-0.0228125
0.0606875
0.144187
1
5
10
20
30
50
70
80
90
95
99
2
2
Predicted
Residuals
Residuals vs. Predicted
-0.189813
-0.106313
-0.0228125
0.0606875
0.144187
1.08
1.18
1.27
1.37
1.47
145
The effects are shown below in the Design Expert
output. The normal probability plot of effects identifies factors A
, D
, F
, and interactions AD
, AF
, BC
, BG
as important. Design Expert Output
Term Effect SumSqr % Contribtn Model Intercept Model A -9.375 351.562 7.75573 Model B -1.875 14.0625 0.310229 Model C -3.625 52.5625 1.15957 Model D -14.375 826.562 18.2346 Error E 3.625 52.5625 1.15957 Model F -16.625 1105.56 24.3895 Model G -2.125 18.0625 0.398472 Error H 0.375 0.5625 0.0124092 Error J 0.125 0.0625 0.0013788 Model AD 11.625 540.563 11.9252 Error AE 2.125 18.0625 0.398472 Model AF 9.875 390.063 8.60507 Error AH 1.375 7.5625 0.166834 Model BC 11.375 517.563 11.4178 Model BG -12.625 637.562 14.0651 Lenth's ME 13.9775 Lenth's SME 28.3764 (c)
Fit an appropriate model using the factors identified in part (b) above. The analysis of variance and corresponding model is shown below. Factors B
, C
, and G
are included for hierarchal purposes. Design Expert Output
Response:
c
ANOVA for Selected Factorial Model
Analysis of variance table [Partial sum of squares]
Sum of
Mean
F
Source
Squares
DF
Square
Value
Prob > F
Model 4454.13 10 445.41 28.26 0.0009 significant A
351.56
1
351.56
22.30
0.0052
B
14.06
1
14.06
0.89
0.3883
C
52.56
1
52.56
3.33
0.1274
D
826.56
1
826.56
52.44
0.0008
F
1105.56
1
1105.56
70.14
0.0004
G
18.06
1
18.06
1.15
0.3333
AD
540.56
1
540.56
34.29
0.0021
DESIGN-EXPERT Plot
c
A: A
B: B
C: C
D: D
E: E
F: F
G: G
H: H
J: J
N o rm a l p lo t
N o rm a l % p ro b a b ility
E ffe c t
-1 6 .6 2
-9 .5 6
-2 .5 0
4 .5 6
1 1 .6 2
1
5
1 0
2 0
3 0
5 0
7 0
8 0
9 0
9 5
9 9
A
D
F
AD
AF
B C
B G
146
AF
390.06
1
390.06
24.75
0.0042
BC
517.56
1
517.56
32.84
0.0023
BG
637.56
1
637.56
40.45
0.0014
Residual 78.81 5 15.76 Cor Total 4532.94 15 The Model F-value of 28.26 implies the model is significant. There is only a 0.09% chance that a "Model F-Value" this large could occur due to noise. Std. Dev. 3.97 R-Squared 0.9826 Mean 10.06 Adj R-Squared 0.9478 C.V. 39.46 Pred R-Squared 0.8220 PRESS 807.04 Adeq Precision 17.771 Coefficient
Standard
95% CI
95% CI
Factor
Estimate
DF
Error
Low
High
VIF
Intercept 10.06 1 0.99 7.51 12.61 A-A -4.69 1 0.99 -7.24 -2.14 1.00 B-B -0.94 1 0.99 -3.49 1.61 1.00 C-C -1.81 1 0.99 -4.36 0.74 1.00 D-D -7.19 1 0.99 -9.74 -4.64 1.00 F-F -8.31 1 0.99 -10.86 -5.76 1.00 G-G -1.06 1 0.99 -3.61 1.49 1.00 AD 5.81 1 0.99 3.26 8.36 1.00 AF 4.94 1 0.99 2.39 7.49 1.00 BC 5.69 1 0.99 3.14 8.24 1.00 BG -6.31 1 0.99 -8.86 -3.76 1.00 Final Equation in Terms of Coded Factors:
c = +10.06 -4.69 * A -0.94 * B -1.81 * C -7.19 * D -8.31 * F -1.06 * G +5.81 * A * D +4.94 * A * F +5.69 * B * C -6.31 * B * G Final Equation in Terms of Actual Factors:
c = +10.06250 -4.68750 * A -0.93750 * B -1.81250 * C -7.18750 * D -8.31250 * F -1.06250 * G +5.81250 * A * D +4.93750 * A * F +5.68750 * B * C -6.31250 * B * G (d)
Plot the residuals from this model versus the predicted number of defects. Also, prepare a normal probability plot of the residuals. Comment on the adequacy of these plots. 147
There is a significant problem with inequality of variance. This is likely caused by the response variable being a count. A transformation may be appropriate. (e)
In part (d) you should have noticed an indication that the variance of the response is not constant (considering that the response is a count, you should have expected this). The previous table also shows a transformation on c, the square root, that is a widely used variance stabilizing transformation for count data (refer to the discussion of variance stabilizing transformations in Chapter 3). Repeat parts (a) through (d) using the transformed response and comment on your results. Specifically, are the residual plots improved? Design Expert Output Term Effect SumSqr % Contribtn Model Intercept Error A -0.895 3.2041 4.2936 Model B -0.3725 0.555025 0.743752 Error C -0.6575 1.72922 2.31722 Model D -2.1625 18.7056 25.0662 Error E 0.4875 0.950625 1.27387 Model F -2.6075 27.1962 36.4439 Model G -0.385 0.5929 0.794506 Error H 0.27 0.2916 0.390754 Error J 0.06 0.0144 0.0192965 Error AD 1.145 5.2441 7.02727 Error AE 0.555 1.2321 1.65106 Error AF 0.86 2.9584 3.96436 Error AH 0.0425 0.007225 0.00968175 Error BC 0.6275 1.57502 2.11059 Model BG -1.61 10.3684 13.894 Lenth's ME 2.27978 Lenth's SME 4.62829 The analysis of the data with the square root transformation identifies only D
, F
, the BG
interaction as being significant. The original analysis identified factor A
and several two factor interactions as being significant. Residual
Normal % probability
Normal plot of residuals
-3.8125
-1.90625
0
1.90625
3.8125
1
5
10
20
30
50
70
80
90
95
99
Predicted
Residuals
Residuals vs. Predicted
-3.8125
-1.90625
0
1.90625
3.8125
-3.81
10.81
25.44
40.06
54.69
148
Design Expert Output Response:
sqrt
ANOVA for Selected Factorial Model
Analysis of variance table [Partial sum of squares]
Sum of
Mean
F
Source
Squares
DF
Square
Value
Prob > F
Model 57.42 5 11.48 6.67 0.0056 significant B
0.56
1
0.56
0.32
0.5826
D
18.71
1
18.71
10.87
0.0081
F
27.20
1
27.20
15.81
0.0026
G
0.59
1
0.59
0.34
0.5702
BG
10.37
1
10.37
6.03
0.0340
Residual 17.21 10 1.72 Cor Total 74.62 15 The Model F-value of 6.67 implies the model is significant. There is only a 0.56% chance that a "Model F-Value" this large could occur due to noise. Std. Dev. 1.31 R-Squared 0.7694 Mean 2.32 Adj R-Squared 0.6541 C.V. 56.51 Pred R-Squared 0.4097 PRESS 44.05 Adeq Precision 8.422 Coefficient
Standard
95% CI
95% CI
Factor
Estimate
DF
Error
Low
High
VIF
Intercept 2.32 1 0.33 1.59 3.05 B-B -0.19 1 0.33 -0.92 0.54 1.00 D-D -1.08 1 0.33 -1.81 -0.35 1.00 F-F -1.30 1 0.33 -2.03 -0.57 1.00 G-G -0.19 1 0.33 -0.92 0.54 1.00 BG -0.80 1 0.33 -1.54 -0.074 1.00 Final Equation in Terms of Coded Factors:
sqrt = +2.32 -0.19 * B -1.08 * D -1.30 * F -0.19 * G -0.80 * B * G Final Equation in Terms of Actual Factors:
sqrt = DESIGN-EXPERT Plot
sqrt
A: A
B: B
C: C
D: D
E: E
F: F
G: G
H: H
J: J
N o rm a l p lo t
N o rm a l % p ro b a b ility
E ffe c t
-2 .6 1
-1 .6 7
-0 .7 3
0 .2 1
1 .1 4
1
5
1 0
2 0
3 0
5 0
7 0
8 0
9 0
9 5
9 9
D
F
B G
149
+2.32125 -0.18625 * B -1.08125 * D -1.30375 * F -0.19250 * G -0.80500 * B * G The residual plots are acceptable; although, there appears to be a slight “u” shape to the residuals versus predicted plot. (f)
There is a modification to the square root transformation proposed by Freeman and Tukey (“Transformations Related to the Angular and the Square Root,” Annals of Mathematical Statistics
, Vol. 21, 1950, pp. 607-611) that improves its performance. F&T’s modification to the square root transformation is: [
]
1
2
1
+
+
c
c
Rework parts (a) through (d) using this transformation and comment on the results. (For an interesting discussion and analysis of this experiment, refer to “Analysis of Factorial Experiments with Defects or Defectives as the Response,” by S. Bisgaard and H.T. Fuller, Quality Engineering
, Vol. 7, 1994-5, pp. 429-443.) Design Expert Output Term Effect SumSqr % Contribtn Model Intercept Error A -0.86 2.9584 4.38512 Model B -0.325 0.4225 0.626255 Error C -0.605 1.4641 2.17018 Model D -1.995 15.9201 23.5977 Error E 0.5025 1.01002 1.49712 Model F -2.425 23.5225 34.8664 Model G -0.4025 0.648025 0.960541 Error H 0.225 0.2025 0.300158 Error J 0.0275 0.003025 0.00448383 Error AD 1.1625 5.40562 8.01254 Error AE 0.505 1.0201 1.51205 Error AF 0.8825 3.11523 4.61757 Error AH 0.0725 0.021025 0.0311645 Error BC 0.7525 2.26503 3.35735 Residual
Normal % probability
Normal plot of residuals
-2.1125
-1.09062
-0.06875
0.953125
1.975
1
5
10
20
30
50
70
80
90
95
99
Predicted
Residuals
Residuals vs. Predicted
-2.1125
-1.09062
-0.06875
0.953125
1.975
-1.25
0.44
2.13
3.83
5.52
150
Your preview ends here
Eager to read complete document? Join bartleby learn and gain access to the full version
- Access to all documents
- Unlimited textbook solutions
- 24/7 expert homework help
Related Questions
A manufacturing company needs a Pareto diagram for the analysis of thefollowing design department cost of poor quality :
using the image attach
Analyze the constructed Pareto Diagram.
arrow_forward
In what ways could each of the factors listed contribute to poor quality?f. Product design
arrow_forward
82
arrow_forward
why does the control the experiement not fall within the expected ranges of controls (quality control)?
arrow_forward
The Blitz Ltd.,an oil manufacturer. In their QA plan, It was mentioned that the the pouring temperature is very critical characteristic, thus required monitoring of Cp and Cpk. The specification of pouring temperature is 1400 +/- 10 degrees Celsius. The process engineer performed the inspection and came up with the following summary:
mean range = 1.45d2 value (from the table) = 2.326 ( for n of 5)mean temp = 1400.42
compute for estimated standard deviation
compute for Cp and Cpk values
arrow_forward
Subject : engineering design - DFMA
arrow_forward
Explain Why testing can detect the presence of errors, not their absence.
arrow_forward
when preparing QFD on a soft drink, which of the following is least effective to understand customer requirements about Cannes material characteristics?
a stays cool
b attractive
c reusable
d does not change shape
arrow_forward
List and briefly explain:b. The determinants of quality
arrow_forward
What is the characteristic of a set of Specifications?
arrow_forward
Distinguish between the expenses of conformity and nonconformance, and explain how they both affect the overall cost of quality.
arrow_forward
Explain can quality improvement dramatically increase revenue?
arrow_forward
In what ways could each of the factors listed contribute to poor quality?e. Control and monitoring
arrow_forward
why quality maanagement is so important in supply chain ?
arrow_forward
Problem 12-7 (Algo)
The following table lists all costs of quality incurred by Sam's Surf Shop last year.
Annual inspection costs
Annual cost of scrap materials
Annual rework cost
Annual cost of quality training
Annual warranty cost
Annual testing cost
$ 170,000
346,000
Appraisal cost
94, 679
516, 000
1, 666, 000
532, 000
What was Sam's appraisal cost for quality last year?
arrow_forward
Processing times for new accounts at a bank are shown in the following table. Five samples of
four observations each have been taken. Use the sample data in conjunction with the Table of
Constants for Control Charts (provided) to construct upper and lower control limits for both a
mean chart and a range chart. Do the results suggest that the process is in control?
Total
Sample 1
10.2
9.9
9.8
10.1
40.0
Sample 2
10.3
9.8
9.9
10.4
40.4
Sample 3
9.7
9.9
9.9
10.1
39.6
Sample 4
9.9
10.3
10.1
10.5
40.8
Sample 5
9.8
10.2
10.3
9.7
40.0
arrow_forward
Objective: Share knowledge and understanding of key quality improvement concepts.
explain the concept of quality improvement and to convey its importance.
arrow_forward
How does the lack of quality, affects the entire organization from supplier to customer and from product design to maintenance?
arrow_forward
Help me answer this question. Thank you!
arrow_forward
Alpesh
arrow_forward
Identify which of the Seven QC Tools would be most useful in addressing each of the following situations:
A copy machine suffers frequent paper jams, and users are often confused as to how to fix the problem.
The publication team for an engineering department wants to improve the accuracy of its user documentation but is unsure of why documents are not error-free.
A bank needs to determine how many teller positions, drive-through stations, and ATM machines it needs for a new branch bank in a certain busy location. Its information includes the average numbers and types of customers served by other similar facilities as well as demographic information to suggest the level of customer traffic to the new facility.
A contracting agency wants to investigate why it had so many changes in its contracts. The company believes that the number of changes may be related to the dollar value of the original contract or the days between the request for proposal and the contract award.
A travel…
arrow_forward
Pareto solution
The following table shows the defects identified in the final inspection, during a month, in the soft drink bottles, as well as the number of times these have occurred.
arrow_forward
SEE MORE QUESTIONS
Recommended textbooks for you
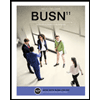
BUSN 11 Introduction to Business Student Edition
Business
ISBN:9781337407137
Author:Kelly
Publisher:Cengage Learning
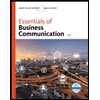
Essentials of Business Communication (MindTap Cou...
Business
ISBN:9781337386494
Author:Mary Ellen Guffey, Dana Loewy
Publisher:Cengage Learning
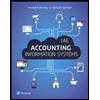
Accounting Information Systems (14th Edition)
Business
ISBN:9780134474021
Author:Marshall B. Romney, Paul J. Steinbart
Publisher:PEARSON
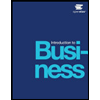
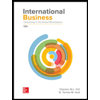
International Business: Competing in the Global M...
Business
ISBN:9781259929441
Author:Charles W. L. Hill Dr, G. Tomas M. Hult
Publisher:McGraw-Hill Education
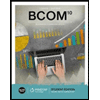
Related Questions
- A manufacturing company needs a Pareto diagram for the analysis of thefollowing design department cost of poor quality : using the image attach Analyze the constructed Pareto Diagram.arrow_forwardIn what ways could each of the factors listed contribute to poor quality?f. Product designarrow_forward82arrow_forward
- why does the control the experiement not fall within the expected ranges of controls (quality control)?arrow_forwardThe Blitz Ltd.,an oil manufacturer. In their QA plan, It was mentioned that the the pouring temperature is very critical characteristic, thus required monitoring of Cp and Cpk. The specification of pouring temperature is 1400 +/- 10 degrees Celsius. The process engineer performed the inspection and came up with the following summary: mean range = 1.45d2 value (from the table) = 2.326 ( for n of 5)mean temp = 1400.42 compute for estimated standard deviation compute for Cp and Cpk valuesarrow_forwardSubject : engineering design - DFMAarrow_forward
- Explain Why testing can detect the presence of errors, not their absence.arrow_forwardwhen preparing QFD on a soft drink, which of the following is least effective to understand customer requirements about Cannes material characteristics? a stays cool b attractive c reusable d does not change shapearrow_forwardList and briefly explain:b. The determinants of qualityarrow_forward
arrow_back_ios
SEE MORE QUESTIONS
arrow_forward_ios
Recommended textbooks for you
- BUSN 11 Introduction to Business Student EditionBusinessISBN:9781337407137Author:KellyPublisher:Cengage LearningEssentials of Business Communication (MindTap Cou...BusinessISBN:9781337386494Author:Mary Ellen Guffey, Dana LoewyPublisher:Cengage LearningAccounting Information Systems (14th Edition)BusinessISBN:9780134474021Author:Marshall B. Romney, Paul J. SteinbartPublisher:PEARSON
- International Business: Competing in the Global M...BusinessISBN:9781259929441Author:Charles W. L. Hill Dr, G. Tomas M. HultPublisher:McGraw-Hill Education
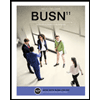
BUSN 11 Introduction to Business Student Edition
Business
ISBN:9781337407137
Author:Kelly
Publisher:Cengage Learning
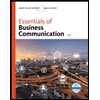
Essentials of Business Communication (MindTap Cou...
Business
ISBN:9781337386494
Author:Mary Ellen Guffey, Dana Loewy
Publisher:Cengage Learning
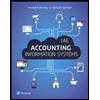
Accounting Information Systems (14th Edition)
Business
ISBN:9780134474021
Author:Marshall B. Romney, Paul J. Steinbart
Publisher:PEARSON
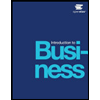
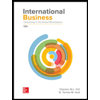
International Business: Competing in the Global M...
Business
ISBN:9781259929441
Author:Charles W. L. Hill Dr, G. Tomas M. Hult
Publisher:McGraw-Hill Education
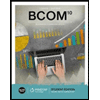