SalazarRodriguezCOOH
pdf
School
Case Western Reserve University *
*We aren’t endorsed by this school
Course
442
Subject
Chemistry
Date
Dec 6, 2023
Type
Pages
7
Uploaded by DeanProton22033
Determination of the pK
a
of
Carboxylic Acids
Allison M. Salazar Rodriguez
DateString
[]
Wed 28 Aug 2013 19:30:32
Research Question
The guiding question for this designated lab is: What single quantum descriptor provides the most
accurate predictor for the pKa of carboxylic acids and what is the pK
a
value of the unknown carboxylic
acid? To further this approach these questions with a solution, knowledge acquired regarding quantita-
tive structure property relationships was utilized as well as an analysis and calculation of linear regres-
sion lines for all six of the observed carboxylic acids.
Scientific Background
In this lab, because computational chemistry is being used to determine the prediction of an unknown
pK
a
value it is important to first understand what computational chemistry helps us achieve and why
we utilize this resource for predictions. So why do we use computational chemistry versus traditional
chemistry? The reason why computational chemistry is so important to achieve solutions to questions
like predictions is that this form of chemistry has the specific ability to predict characteristics and
properties of molecules without having to perform traditional calculations in a laboratory setting or
experiment! Imagine trying to work in a laboratory and finding the prediction to a theoretical prob-
lem
…
you wouldn’t be able to observe anything tangible! This is why in order for the properties of
molecules to be predicted, computational chemists develop procedures and equations for consequen-
tial calculations.
Just like in this lab, the prediction that is trying to be made is a very common molecular property, pK
a
.
pK
a
is a measure of the strength of an acid, basically how strong or weak it is! Even though there is
another tool to observe the strength of an acid, the pH scale, pK
a
is a more reflective measure which
has similar properties: the lower the pK
a
, the more acidic.
Printed by Wolfram Mathematica Student Edition
So now that we’ve discussed why we use computational chemistry and what is the variable property
we are trying to predict today let us discuss the special type of technique that is going to be used as
part of a computational chemist. The specific procedure we are going to be using is called QSPR:
quantitative structure property relationship. But what does this mean?! Well, QSPR essentially just
means using an equation to find a property of a molecule the quantitative statement being the equa-
tion and the computational calculation being the structure.
So how will this be done? What special type of equation will we use for correlation? Well, we are going
to be using none other than a linear regression equation (y = mx + b)! It is between this equation where
two variables will be used to be able to determine the pK
a
of our unknown carboxylic acid. The y in this
instance will be the pK
a
and the x is one of six quantum descriptors, which are other factors and proper-
ties of molecules that we can determine computationally. These quantum descriptors include energy,
dipole moment, positive electrostatic potentials, HOMO energy, LUMO energy, and HOMO/LUMO gap
energy. It is through the determination of the most accurate single quantum descriptor that will pro-
vide the most accurate predictor for pKa, which is determined through the correlation coefficient
closest to 1.
Procedure
Foremost, because this lab contains two portions it is crucial to first determine which of the acquired
single quantum descriptors provides the most accurate predictor for the pK
a
of carboxylic acids. In
order to do so each of these six carboxyl acids given has to be built and optimized via the North Car-
olina High School Computational Chemistry Server via the computer program MOPAC PM3. The lab
required that the acids be built separately in order for them to be properly optimized and symmetrized.
To begin building the molecules, the first approach was to build the carbon backbone with the car-
bonyl group of carbon and oxygen double bond (C=O) attached to then a hydroxide -OH group. Follow-
ing this, a comprehensive cleanup was run with mechanic optimization as the initial form of optimiza-
tion along with the symmetrization of the molecule. Proceeding with this molecular cleanup, a geome-
try optimization under MOPAC PM3 was run congruent with standard procedure for any meaningful
calculation to ensure that the atomic arrangement is stable enough for calculations to be conducted
and has a potential energy surface minimum. Using a “New Job using this geometry” a molecular
orbital calculation was run under the program MOPAC utilizing the same theory of PM3. After this was
accomplished, the necessary single quantum descriptors were recorded which included the energy,
dipole moment, HOMO energy, LUMO energy, and positive electrostatic potential energy for each of
the six molecules. The majority of these descriptors are readily available after viewing the job for a
respective molecule under “Calculated Qualities” but to find the positive electrostatic potential energy
the color scheme for occupied orbitals had to be analyzed. To calculate the HOMO/LUMO gap the
magnitude of the difference between HOMO and LUMO energies were taken. After all these values were
inputted into a chart, three values had to be calculated: the slope, correlation, and intercept for each
descriptor. The most accurate was determined by calculating the correlation between the quantum
2
SalazarRodriguezCOOH.nb
Printed by Wolfram Mathematica Student Edition
descriptor and provided pK
a
value and the “R” value of the linear regression line closest to a value of 1
represents the best descriptor. Lastly, to roughly identify an estimate for the pKa of the unknown
carboxylic acid, a slope intercept equation had to be generated using the generated slope and inter-
cept from recorded values. Because y is the pKa this would predict the pK
a
value.
Calculated Quantities
In[725]:=
data
=
"Cmpd" "pK
a
"
"Energy"
"Dipole" "ElPot"
"HOMO"
"LUMO" "HOMO
/
LUMO Gap"
1
1.23
-
154.87620
0.00
0.0138
-
11.594
-
0.979
10.615
2
4.19
-
36.4087
4.646
0.0482
-
10.62
-
0.7
10.894
3
3.51
-
107.25216
0.00
0.0373
-
11.081
-
1.659
8.961
4
3.1
-
121.67019
2.635
0.0428
-
11.483
-
1.746
9.395
5
4.7
-
70.27334
4.538
0.0556
-
10.677
-
0.423
11.06
;
In[727]:=
Data
=
Delete
[
data, 1
]
;
In[728]:=
{
cmpd, pka, energy, dipole, elpot, HOMO, LUMO, HLgap
} =
Transpose
[
Alldata
]
;
Computational Results: Linear Regression Comparison of Descriptors vs. pK
a
In[734]:=
EnergyData
=
Transpose
[{
energy, pka
}]
;
In[735]:=
EnergyFunction
=
LinearModelFit
[
EnergyData,
{
x1energy
}
,
{
x1energy
}]
Out[735]=
FittedModel
5.83449
+
0.0253678 x1energy
In[732]:=
energyfit
=
EnergyFunction
[
"RSquared"
]
;
In[744]:=
Print
[
"The correlation coefficient for the energies of the
carboxylic acids in relation to their pK
a
is: ", energyfit
]
The correlation coefficient for the energies
of the carboxylic acids in relation to their pK
a
is: 0.764121
In[737]:=
DipoleData
=
Transpose
[{
dipole, pka
}]
;
SalazarRodriguezCOOH.nb
3
Printed by Wolfram Mathematica Student Edition
Your preview ends here
Eager to read complete document? Join bartleby learn and gain access to the full version
- Access to all documents
- Unlimited textbook solutions
- 24/7 expert homework help
In[738]:=
DipoleFunction
=
LinearModelFit
[
DipoleData,
{
x1dipole
}
,
{
x1dipole
}]
Out[738]=
FittedModel
2.29517
+
0.444551 x1dipole
In[740]:=
dipolefit
=
DipoleFunction
[
"RSquared"
]
;
In[745]:=
Print
[
"The correlation coefficient for the dipole moments of
the carboxylic acids in relation to their pK
a
is: ", dipolefit
]
The correlation coefficient for the dipole moments
of the carboxylic acids in relation to their pK
a
is: 0.588781
In[746]:=
ElPotData
=
Transpose
[{
elpot, pka
}]
;
In[747]:=
ElPotFunction
=
LinearModelFit
[
ElPotData,
{
x1elpot
}
,
{
x1elpot
}]
Out[747]=
FittedModel
0.125634
+
81.4458 x1elpot
In[748]:=
elpotfit
=
ElPotFunction
[
"RSquared"
]
;
In[749]:=
Print
[
"The correlation coefficient for the positive electrostatic potentials
of the carboxylic acids in relation to their pK
a
is: ", elpotfit
]
The correlation coefficient for the positive electrostatic
potentials of the carboxylic acids in relation to their pK
a
is: 0.943268
In[750]:=
HOMOdata
=
Transpose
[{
HOMO, pka
}]
;
In[751]:=
HOMOfunction
=
LinearModelFit
[
HOMOdata,
{
x1HOMO
}
,
{
x1HOMO
}]
Out[751]=
FittedModel
32.7437
+
2.65059 x1HOMO
In[753]:=
HOMOfit
=
HOMOfunction
[
"RSquared"
]
;
4
SalazarRodriguezCOOH.nb
Printed by Wolfram Mathematica Student Edition
In[755]:=
Print
[
"The correlation coefficient for the highest occupied molecular orbital
of the carboxylic acids in relation to their pK
a
is: ", HOMOfit
]
The correlation coefficient for the highest occupied molecular
orbital of the carboxylic acids in relation to their pK
a
is: 0.79046
In[756]:=
LUMOdata
=
Transpose
[{
LUMO, pka
}]
;
In[757]:=
LUMOfunction
=
LinearModelFit
[
LUMOdata,
{
x1LUMO
}
,
{
x1LUMO
}]
Out[757]=
FittedModel
4.20712
+
0.781843 x1LUMO
In[761]:=
LUMOfit
=
LUMOfunction
[
"RSquared"
]
;
In[762]:=
Print
[
"The correlation coefficient for the lowest unoccupied molecular orbital
of the carboxylic acids in relation to their pK
a
is: ", LUMOfit
]
The correlation coefficient for the lowest unoccupied molecular
orbital of the carboxylic acids in relation to their pK
a
is: 0.117154
In[764]:=
HLgapData
=
Transpose
[{
HLgap, pka
}]
;
In[765]:=
HLgapFunction
=
LinearModelFit
[
HLgapData,
{
x1HLgap
}
,
{
x1HLgap
}]
Out[765]=
FittedModel
0.876663
+
0.242448 x1HLgap
In[767]:=
HLgapFit
=
HLgapFunction
[
"RSquared"
]
;
In[770]:=
Print
[
"The correlation coefficient for the HOMO and LUMO gap energies
of the carboxylic acids in relation to their pK
a
is: ", HLgapFit
]
The correlation coefficient for the HOMO and LUMO gap
energies of the carboxylic acids in relation to their pK
a
is: 0.0295578
Data Analysis and Calculations: Predicting pK
a
Value for Unknown Carboxylic Acid
In[774]:=
unknownpKa
=
ElPotFunction
[
0.0352
]
;
SalazarRodriguezCOOH.nb
5
Printed by Wolfram Mathematica Student Edition
In[775]:=
Print
[
"The predicted pK
a
value of the sixth unknown carboxylic acid is ",
unknownpKa
]
The predicted pK
a
value of the sixth unknown carboxylic acid is 2.99253
In[778]:=
Alldata
=
1 1.23
-
154.87620
0.00
0.0138
-
11.594
-
0.979 10.615
2 4.19
-
36.4087
4.646 0.0482
-
10.62
-
0.7
10.894
3 3.51
-
107.25216
0.00
0.0373
-
11.081
-
1.659
8.961
4
3.1
-
121.67019 2.635 0.0428
-
11.483
-
1.746
9.395
5
4.7
-
70.27334
4.538 0.0556
-
10.677
-
0.423
11.06
6 2.99
-
99.33978
1.654 0.0352
-
11.485
0.962
11.639
;
In[782]:=
Grid
[
Prepend
[
Alldata,
{
"Carboxylic\nAcid", "pK
a
", "Energy\n
(
kcal
/
mol
)
",
"Dipole\nMoment", "Positive\nElectrostatic\nPotentials", "HOMO\n
(
eV
)
",
"LUMO\n
(
eV
)
", "HOMO
/
LUMO\nEnergy Gaps"
}]
, Alignment
→
Center, Frame
→
All
]
Out[782]=
Carboxylic
Acid
pK
a
Energy
(
kcal
/
mol
)
Dipole
Moment
Positive
Electrostatic
Potentials
HOMO
(
eV
)
LUMO
(
eV
)
HOMO
/
LUMO
Energy Gaps
1
1.23
-
154.876
0.
0.0138
-
11.594
-
0.979
10.615
2
4.19
-
36.4087
4.646
0.0482
-
10.62
-
0.7
10.894
3
3.51
-
107.252
0.
0.0373
-
11.081
-
1.659
8.961
4
3.1
-
121.67
2.635
0.0428
-
11.483
-
1.746
9.395
5
4.7
-
70.2733
4.538
0.0556
-
10.677
-
0.423
11.06
6
2.99
-
99.3398
1.654
0.0352
-
11.485
0.962
11.639
Figure 1. Completed data table of all six carboxylic acids, including predicted pK
a
value
Lab Abstract
In this lab, the question regarding the most accurate single quantum descriptor that provided the most
accurate predictor for the pKa of carboxylic acids as well as the predicted value for the sixth unknown
carboxylic acid was evaluated. The effect of the molecular orbital calculations on the six carboxylic
acids and the determination of slopes for all six of the quantum descriptors in correlation to pKa was
that the most accurate was positive electrostatic potential. The linear regression equation for this
calculation was 32.7437 + 2.65059 x1HOMO which resulted in a then substitution of the sixth carboxylic
acid positive electrostatic potential of 0.0352 that generated a predicted pKa value of 2.99. This lab
primarily focused on the method of PM3 utilizing the computer program MOPAC for optimization as
well as for the calculated theory of molecular orbital in the North Carolina High School Computational
Chemistry Server. The data acquired were analyzed via data tables and the visualization of molecular
orbitals through multiple linear regressions demonstrated mathematically.
6
SalazarRodriguezCOOH.nb
Printed by Wolfram Mathematica Student Edition
Your preview ends here
Eager to read complete document? Join bartleby learn and gain access to the full version
- Access to all documents
- Unlimited textbook solutions
- 24/7 expert homework help
Keywords: multiple linear regression, carboxylic acid, pK
a
,
quantum descriptors, correlation coefficient
SalazarRodriguezCOOH.nb
7
Printed by Wolfram Mathematica Student Edition
Related Documents
Related Questions
The leaves of the Brazilian Tree Senna multijunga contain a number of pryidine alkaloids that inhibit acetylcholinterinase. Two recentyl isolated isomeric compounds have the strcture have the strcture shown below. (NOTE: M=293) Use the mass spectral data provided to determine the precise location of the hydroxyl group in each isomer.
Isomer A: EI-MS, m/z(rel. int): 222(20), 150(10), 136(25), 123(100)
Isomer B:EI-MS, m/z(re;. int): 236(20), 150(10), 136(25), 123(100)
arrow_forward
Analyze the HNMR spectrum below and identify the compound
arrow_forward
can you please analyze this spectra (FTIR) for 2-phenylpropan-2-ol thank you.
arrow_forward
Each peak in the spectrum below can be used to provide a
measure of the mass of this protein, you can work out the charge associated with
each peak, and measures can be averaged to find the overall mass. Use
simultaneous equations to find the charge associated with each peak in the spectra
below, which will allow you to calculate the average mass of each species. Include
an estimate of the error associated with this measure.
arrow_forward
Analyze the HNMR spectrum below and identify the compound
arrow_forward
This is the IR for Carbon Dioxide. What do the peaks inside circled mean?
arrow_forward
ve Transmittance
0.6
3000
Anthracene
INFRARED SPECTRUM
P
Wavenumber (cm-1)
1000
NIST Chemistry WebBook (https://webbook.nist.gov/chemistry)
1- For the above shown IR-Spectrum (Indicate the structure of the organic molecule).
What are the characteristic peaks present?
2- Show (or draw) the IR spectrum, The NMR Spectrum and the Mass spectrum for
Methyl ethyl ester.
3- Show or hand draw the IR spectrum, The NMR Spectrum and the Mass spectrum
for Acetyl Salicylic acid (commonly known as Aspirin)
X
arrow_forward
Identify the peaks for this compound and what functional groups are present
arrow_forward
A food company has just received a vanilla extract shipment that quality assurance needs to approve. It is known that adulteration with ethyl vanillin (3X more intense thant vanillin) sometimes occurs when vanilla extract prices increase. From the NMR data below for this shipment, what do you tell purchasing? Should the company be concerned?
arrow_forward
Can you explain this nmr
arrow_forward
7) The correct structure that corresponds to the
spectroscopic data given below is [IR 2720, 1710
1 point
cm*1. 1H NMR 9.80(s, 1H), 7.50(dd, J = 8,0Hz, 2Hz, 1H),
7.40(d, J = 2.0Hz, 1H), 6.90(d, J = 8.0Hz, 1H), 3.90(s,
3H), 3.80(s, 3H)]
COOH
OMe
Me
CHO
OMe
OMe
CHO
OMe
OMe
COOH
OMe
Me
arrow_forward
What is the thaoretical yiekt of 2 methyl 2 tutene MW-70.13gimot d- 2 gim)y cted 23 2 chiore2 tytane MW 10 sma d-04 the ana
Кон
E2
OA199 nL
Oc36 mt
O0.none of the answers
OR164t
QUESTION 2
Which of the following would not incease the retentien time ot an aalyte ina GC t geyg o
whowss stationary phase
DA Jngthen the colimn
On incren the tomperature of the aven
OC AN of the above wodd incease the retention tie
OD. decrease the pressure of the mobile phase (He gas)
O merease te amount of stationary phase in the colun
arrow_forward
Please help
arrow_forward
10- If 3-Bromo-1-phenyl-1-propene shows a complex NMR spectrum in which thevinylic proton at C2 is couples with both the C1 vinylic proton (J = 16 Hz) and the C3methylene protons (J = 8 Hz) Draw a tree diagram for the C2 proton signal.
arrow_forward
can the frequency and singlts be found based on the two spectrs for 1HMR and 2CMR
and both of them be explained
arrow_forward
Describe the splitting pattern and assign and label the H of the spectrum of C4H8O
arrow_forward
SEE MORE QUESTIONS
Recommended textbooks for you
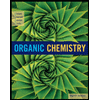
Organic Chemistry
Chemistry
ISBN:9781305580350
Author:William H. Brown, Brent L. Iverson, Eric Anslyn, Christopher S. Foote
Publisher:Cengage Learning
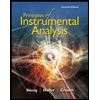
Principles of Instrumental Analysis
Chemistry
ISBN:9781305577213
Author:Douglas A. Skoog, F. James Holler, Stanley R. Crouch
Publisher:Cengage Learning
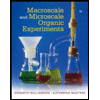
Macroscale and Microscale Organic Experiments
Chemistry
ISBN:9781305577190
Author:Kenneth L. Williamson, Katherine M. Masters
Publisher:Brooks Cole
Related Questions
- The leaves of the Brazilian Tree Senna multijunga contain a number of pryidine alkaloids that inhibit acetylcholinterinase. Two recentyl isolated isomeric compounds have the strcture have the strcture shown below. (NOTE: M=293) Use the mass spectral data provided to determine the precise location of the hydroxyl group in each isomer. Isomer A: EI-MS, m/z(rel. int): 222(20), 150(10), 136(25), 123(100) Isomer B:EI-MS, m/z(re;. int): 236(20), 150(10), 136(25), 123(100)arrow_forwardAnalyze the HNMR spectrum below and identify the compoundarrow_forwardcan you please analyze this spectra (FTIR) for 2-phenylpropan-2-ol thank you.arrow_forward
- Each peak in the spectrum below can be used to provide a measure of the mass of this protein, you can work out the charge associated with each peak, and measures can be averaged to find the overall mass. Use simultaneous equations to find the charge associated with each peak in the spectra below, which will allow you to calculate the average mass of each species. Include an estimate of the error associated with this measure.arrow_forwardAnalyze the HNMR spectrum below and identify the compoundarrow_forwardThis is the IR for Carbon Dioxide. What do the peaks inside circled mean?arrow_forward
- ve Transmittance 0.6 3000 Anthracene INFRARED SPECTRUM P Wavenumber (cm-1) 1000 NIST Chemistry WebBook (https://webbook.nist.gov/chemistry) 1- For the above shown IR-Spectrum (Indicate the structure of the organic molecule). What are the characteristic peaks present? 2- Show (or draw) the IR spectrum, The NMR Spectrum and the Mass spectrum for Methyl ethyl ester. 3- Show or hand draw the IR spectrum, The NMR Spectrum and the Mass spectrum for Acetyl Salicylic acid (commonly known as Aspirin) Xarrow_forwardIdentify the peaks for this compound and what functional groups are presentarrow_forwardA food company has just received a vanilla extract shipment that quality assurance needs to approve. It is known that adulteration with ethyl vanillin (3X more intense thant vanillin) sometimes occurs when vanilla extract prices increase. From the NMR data below for this shipment, what do you tell purchasing? Should the company be concerned?arrow_forward
- Can you explain this nmrarrow_forward7) The correct structure that corresponds to the spectroscopic data given below is [IR 2720, 1710 1 point cm*1. 1H NMR 9.80(s, 1H), 7.50(dd, J = 8,0Hz, 2Hz, 1H), 7.40(d, J = 2.0Hz, 1H), 6.90(d, J = 8.0Hz, 1H), 3.90(s, 3H), 3.80(s, 3H)] COOH OMe Me CHO OMe OMe CHO OMe OMe COOH OMe Mearrow_forwardWhat is the thaoretical yiekt of 2 methyl 2 tutene MW-70.13gimot d- 2 gim)y cted 23 2 chiore2 tytane MW 10 sma d-04 the ana Кон E2 OA199 nL Oc36 mt O0.none of the answers OR164t QUESTION 2 Which of the following would not incease the retentien time ot an aalyte ina GC t geyg o whowss stationary phase DA Jngthen the colimn On incren the tomperature of the aven OC AN of the above wodd incease the retention tie OD. decrease the pressure of the mobile phase (He gas) O merease te amount of stationary phase in the colunarrow_forward
arrow_back_ios
SEE MORE QUESTIONS
arrow_forward_ios
Recommended textbooks for you
- Organic ChemistryChemistryISBN:9781305580350Author:William H. Brown, Brent L. Iverson, Eric Anslyn, Christopher S. FootePublisher:Cengage LearningPrinciples of Instrumental AnalysisChemistryISBN:9781305577213Author:Douglas A. Skoog, F. James Holler, Stanley R. CrouchPublisher:Cengage LearningMacroscale and Microscale Organic ExperimentsChemistryISBN:9781305577190Author:Kenneth L. Williamson, Katherine M. MastersPublisher:Brooks Cole
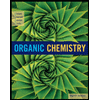
Organic Chemistry
Chemistry
ISBN:9781305580350
Author:William H. Brown, Brent L. Iverson, Eric Anslyn, Christopher S. Foote
Publisher:Cengage Learning
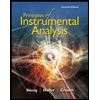
Principles of Instrumental Analysis
Chemistry
ISBN:9781305577213
Author:Douglas A. Skoog, F. James Holler, Stanley R. Crouch
Publisher:Cengage Learning
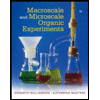
Macroscale and Microscale Organic Experiments
Chemistry
ISBN:9781305577190
Author:Kenneth L. Williamson, Katherine M. Masters
Publisher:Brooks Cole