S23 - Assignment #3 - Solutions
.pdf
keyboard_arrow_up
School
University of Waterloo *
*We aren’t endorsed by this school
Course
371
Subject
Statistics
Date
Apr 3, 2024
Type
Pages
9
Uploaded by BrigadierAntelopePerson2879
STAT 371 S23 Assignment #3 (Submission deadline: 11:59 pm Fri. Jul. 14th)
Solutions ( /70) In this assignment, we will continue with developing a suitable regression model for the CEO data from Assignment #2, beginning with your fitted model used in 2e) of Assignment #2 (i.e. model without Background variate). 1) [5] Plot the residuals vs the fitted values, as well as a QQ plot. Comment on the adequacy of the fitted model, in terms of the model assumptions. We do not appear to have an adequate model. The pattern evident in the plot of the residuals vs the fitted values reveals a misspecification of the functional form and/or non-constant variance. The departure from a straight line relationship in the qq plot is in contradiction to the assumption of normal errors. 2) One approach to stabilize the variance of the residuals and/or more adequately describe the relationship between a response variate and the explanatory variates is with an appropriate transformation of the response variate. a) [3] Create a histogram of CEO compensation. What characteristic of this variate might lead you to suspect that a log transformation may be suitable? The right-skewness in the distribution suggests that a log transformation might help to normalize the response.
b) [2] Refit the data using the (natural) log transformation of compensation. Call: lm(formula = log(COMP) ~ AGE + EDUCATN + TENURE + EXPER + SALES + VAL + PCNTOWN + PROF) Coefficients: Estimate Std. Error t value Pr(>|t|) (Intercept) 6.897e+00 7.211e-01 9.565 7.02e-13 AGE -1.938e-03 1.208e-02 -0.160 0.87324 EDUCATN -3.082e-01 1.160e-01 -2.658 0.01054 TENURE 7.004e-03 6.981e-03 1.003 0.32051 EXPER 1.533e-02 9.554e-03 1.605 0.11489 SALES 2.508e-05 1.636e-05 1.533 0.13151 VAL 1.236e-03 6.158e-04 2.008 0.05011 PCNTOWN -7.308e-02 2.699e-02 -2.708 0.00924 PROF 2.325e-04 3.502e-04 0.664 0.50968 --- Residual standard error: 0.4705 on 50 degrees of freedom Multiple R-squared: 0.4178, Adjusted R-squared: 0.3246 F-statistic: 4.485 on 8 and 50 DF, p-value: 0.0003771 c) [2] Compare the overall fit of the model and significance of the individual parameters with that of the original (untransformed) model. An R-squared value of .4178 indicates that less than 42% of the variation in (log) compensation is accounted for by the variables in the model. This is a slight improvement over the fit of the untransformed model (.4031) PCNTOWN and EDUCATN appear to be the only variable with a significant relationship with compensation, after accounting for the other variables. Note that EXPER has been rendered insignificant by the transformation. d) [4] Replot the two residual plots in 1). Has the transformation helped to address the issues with the adequacy of the (untransformed) model? Yes, the transformation has certainly helped to address the model adequacy issues. The plot of the residuals vs fitted is more randomly scattered. Improvement is also seen in the QQ plot.
3) We can also investigate the suitability of transformations of one or more of the explanatory variates by looking at scatterplots of the variates vs the response (log(COMP), in this case). a) [3] Create a scatterplot of SALES vs log(COMP). Does a linear model seem appropriate for these two variates? No, the relationship between log(COMP) and SALES is not linear. b) [3] Create a scatterplot of log(SALES) vs log(COMP). Comment. The relationship between log(COMP) and log(SALES) appears much more linear (although there appears to be some non-linearity in the relationship for high sales) c) [4] Refit the model once again, this time taking the log transformation of compensation as well as of the variates SALES, VAL, PCNTOWN and PROF. We will use this model going forward. Comment on the effect these transformations have on the overall fit of the model, and on the p-values of the associated variates.
Call: lm(formula = log(COMP) ~ AGE + EDUCATN + TENURE + EXPER + log(SALES) + log(VAL) + log(PCNTOWN) + log(PROF)) Coefficients: Estimate Std. Error t value Pr(>|t|) (Intercept) 5.531845 0.897256 6.165 1.21e-07 AGE 0.002864 0.012122 0.236 0.81418 EDUCATN -0.300500 0.114331 -2.628 0.01137 TENURE -0.003343 0.006511 -0.513 0.60993 EXPER 0.015146 0.010056 1.506 0.13830 log(SALES) 0.188393 0.080064 2.353 0.02260 log(VAL) 0.315447 0.096467 3.270 0.00195 log(PCNTOWN) -0.351228 0.105022 -3.344 0.00157 log(PROF) -0.221603 0.104300 -2.125 0.03858 --- Residual standard error: 0.4428 on 50 degrees of freedom Multiple R-squared: 0.4842, Adjusted R-squared: 0.4017 F-statistic: 5.867 on 8 and 50 DF, p-value: 2.732e-05 The transformations of the explanatory variables appears to have improved the fit of the model substantially, as indicated by the increased R-squared value of .4842 (some of you may not experience the same increase, depending on your sample). There are several variables with associated p-values < .05, including education level, and all the log transformed variables (SALES, VAL, PCNTWN, PROF). 4) [4] Plot the residuals vs the fitted values and the QQ plot for the model in 3). Comment on the effect of the transformations on the model assumptions. The transformations have improved model adequacy considerable. The model appears to be well specified with a relatively constant variance (based on the plot of the residuals vs fitted values), and the qq plot suggests that the assumption of normal errors appears to be more reasonably met than with the untransformed variables.
Your preview ends here
Eager to read complete document? Join bartleby learn and gain access to the full version
- Access to all documents
- Unlimited textbook solutions
- 24/7 expert homework help
Related Questions
A sample consists of 500 houses sold in Karachi between Jamuary 2020 and December 2020. The
multiple linear regression analysis is carried out to predict the house prices for investment in
residential properties in Karachi, Pakistan. The output below is produced using SPSS. (300 words)
Table: Coefficients
Model
Unstandardized
Coefficients
VIF
Constant
14.208
5.736
Age of house
-0.299
-2.322
1.58
Square footage of the house
0.364
2.931
1.71
Income of families in the area
p.004
0.392
1.01
Transportation time to major markets
-0.337
-2.619
1.90
R? = 0.67; DW = 2.08
Dependent Variable: House price (Pakistani rupees in Million)
a) You are required to write the multiple regression equation.
b) How would you interpret the above Output' of a regression analysis performed in SPSS?
c) From the above results, what can you say about the nature of autocorrelation?
d) Is there multicollinearity in regression? How do you know?
arrow_forward
(20 pts) Below are the results of a linear regression model which is built on the Tips.csv data.
Use the results to answer the following questions.
> tipsmodel summary(tipsmodel)
Call:
lm(formula
Residuals:
=
tip
~
=
tip total bill + size + smoker, data = D0)
~
total bill + size + smoker, data = DO)
1Q Median
3Q
Max
4.0573
Min
-2.8965 -0.5601 -0.0722 0.5030
Coefficients:
Estimate Std. Error t value Pr(>Itl)
total_bill
size
0.093942
0.187122
(Intercept) 0.687335 0.207385 3.314
smoker Yes -0.079215
0.009385 10.010
0.088742 2.109
0.139256 -0.569 0.57000
0.00106 **
< 2e-16 ***
0.03603 *
Signif. codes:
0 ***** 0.001 *** 0.01 *** 0.05' 0.1 '1
Residual standard error: 1.019 on 237 degrees of freedom
(3 observations deleted due to missingness)
Multiple R-squared: 0.4713, Adjusted R-squared: 0.4646
F-statistic: 70.41 on 3 and 237 DF, p-value: < 2.2e-16
a) What is the linear regression equation obtained from R?
b) What is the relationship between “tip” and “total_bill” in the linear regression…
arrow_forward
Fit these three regression models and then discuss the similarities and differences between them, particularly as relates to slope estimates (use CI’s) and R2. Also address why this is a “special case” and we wouldn’t necessarily expect to see these model characteristics for a typical dataset.
a) Additive model including both predictors (output attached)
b) Model including only Moisture (output attached)
c) Model including only Sweetness
BrandLiking = 68.62 + 4.38 Sweetness
Term 95% CI P-ValueConstant (50.16, 87.09) 0.000Sweetness (-1.46, 10.21) 0.130
S R-sq R-sq(adj)10.8915 15.57% 9.54%
arrow_forward
The accompanying Minitab regression output is based on data that appeared in the article "Application of Design of Experiments for Modeling Surface Roughness in Ultrasonic Vibration Turning."+ The response variable is surface roughness (um), and the independent variables are vibration amplitude (um), depth of cut (mm), feed rate (mm/rev), and cutting speed (m/min), respectively.
The regression equation is
Ra = -0.972 - 0.0312a + 0.557d + 18.3f + 0.00282v
Predictor
Coef
SE Coef
Constant
-0.9723
0.3923
-2.48
0.015
-0.03117
0.01864
-1.67
0.099
d
0.5568
0.3185
1.75
0.084
18.2602
0.7536
24.23
0.000
0.002822
0.003977
0.71
0.480
S = 0.822059
Source
R-Sq = 88.6
R-Sq (adj) = 88.04
DS
MS
0.000
Regression
Residual Error
4
401.02
100.25
148.35
76
51.36
0.68
Total
80
452.38
(a) How many observations were there in the data set?
observations
(b) Interpret the coefficient of multiple determination.
O 8.0% of the observed variation in feed rate can be explained by the model relationship with vibration…
arrow_forward
We estimate a simple regression Grade-B,+B,Effort + u where the red line represents
Qur estimate for Grade relative to Effort, and the dots represent our data points. Which
OLS assumption is violated in this regression?
arrow_forward
The ols() method in statsmodels module is used to fit a multiple regression model using “Quality” as the response variable and “Speed” and “Angle” as the predictor variables. The output is shown below. A text version is available. What is the correct regression equation based on this output? What is the coefficient of determination? Select one.
arrow_forward
Can you help me answer this question please
arrow_forward
Which of the variables is the indepenent variable and dependent variable for the following question.
fit a simple linear regression model to predict latitudes using average monthly range
lat= latitudes
range= the average monthly range between mean montly maximum and minimum temperatures for a selected set of US cities.
arrow_forward
Use the given dataset*note: Gender takes on a value of 1 if the student is male, and 0 otherwise
Estimate a linear regression model relating overall grade weighted average (OGWA) of student to their gender, available internet speed (mbps) and previous term’s grade weighted average (lgwa)a. Interpret the slope coefficients (discuss their values and statistical significance)b. Are the coefficients jointly statistically significant? Explain your answer.c. How much of the variability of the overall grade weighted average is explained by the variability of the model?
arrow_forward
I need from part 4-9.
arrow_forward
SEE MORE QUESTIONS
Recommended textbooks for you
Algebra & Trigonometry with Analytic Geometry
Algebra
ISBN:9781133382119
Author:Swokowski
Publisher:Cengage
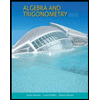
Algebra and Trigonometry (MindTap Course List)
Algebra
ISBN:9781305071742
Author:James Stewart, Lothar Redlin, Saleem Watson
Publisher:Cengage Learning
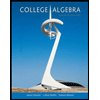
College Algebra
Algebra
ISBN:9781305115545
Author:James Stewart, Lothar Redlin, Saleem Watson
Publisher:Cengage Learning
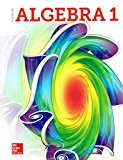
Glencoe Algebra 1, Student Edition, 9780079039897...
Algebra
ISBN:9780079039897
Author:Carter
Publisher:McGraw Hill
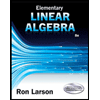
Elementary Linear Algebra (MindTap Course List)
Algebra
ISBN:9781305658004
Author:Ron Larson
Publisher:Cengage Learning
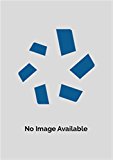
Big Ideas Math A Bridge To Success Algebra 1: Stu...
Algebra
ISBN:9781680331141
Author:HOUGHTON MIFFLIN HARCOURT
Publisher:Houghton Mifflin Harcourt
Related Questions
- A sample consists of 500 houses sold in Karachi between Jamuary 2020 and December 2020. The multiple linear regression analysis is carried out to predict the house prices for investment in residential properties in Karachi, Pakistan. The output below is produced using SPSS. (300 words) Table: Coefficients Model Unstandardized Coefficients VIF Constant 14.208 5.736 Age of house -0.299 -2.322 1.58 Square footage of the house 0.364 2.931 1.71 Income of families in the area p.004 0.392 1.01 Transportation time to major markets -0.337 -2.619 1.90 R? = 0.67; DW = 2.08 Dependent Variable: House price (Pakistani rupees in Million) a) You are required to write the multiple regression equation. b) How would you interpret the above Output' of a regression analysis performed in SPSS? c) From the above results, what can you say about the nature of autocorrelation? d) Is there multicollinearity in regression? How do you know?arrow_forward(20 pts) Below are the results of a linear regression model which is built on the Tips.csv data. Use the results to answer the following questions. > tipsmodel summary(tipsmodel) Call: lm(formula Residuals: = tip ~ = tip total bill + size + smoker, data = D0) ~ total bill + size + smoker, data = DO) 1Q Median 3Q Max 4.0573 Min -2.8965 -0.5601 -0.0722 0.5030 Coefficients: Estimate Std. Error t value Pr(>Itl) total_bill size 0.093942 0.187122 (Intercept) 0.687335 0.207385 3.314 smoker Yes -0.079215 0.009385 10.010 0.088742 2.109 0.139256 -0.569 0.57000 0.00106 ** < 2e-16 *** 0.03603 * Signif. codes: 0 ***** 0.001 *** 0.01 *** 0.05' 0.1 '1 Residual standard error: 1.019 on 237 degrees of freedom (3 observations deleted due to missingness) Multiple R-squared: 0.4713, Adjusted R-squared: 0.4646 F-statistic: 70.41 on 3 and 237 DF, p-value: < 2.2e-16 a) What is the linear regression equation obtained from R? b) What is the relationship between “tip” and “total_bill” in the linear regression…arrow_forwardFit these three regression models and then discuss the similarities and differences between them, particularly as relates to slope estimates (use CI’s) and R2. Also address why this is a “special case” and we wouldn’t necessarily expect to see these model characteristics for a typical dataset. a) Additive model including both predictors (output attached) b) Model including only Moisture (output attached) c) Model including only Sweetness BrandLiking = 68.62 + 4.38 Sweetness Term 95% CI P-ValueConstant (50.16, 87.09) 0.000Sweetness (-1.46, 10.21) 0.130 S R-sq R-sq(adj)10.8915 15.57% 9.54%arrow_forward
- The accompanying Minitab regression output is based on data that appeared in the article "Application of Design of Experiments for Modeling Surface Roughness in Ultrasonic Vibration Turning."+ The response variable is surface roughness (um), and the independent variables are vibration amplitude (um), depth of cut (mm), feed rate (mm/rev), and cutting speed (m/min), respectively. The regression equation is Ra = -0.972 - 0.0312a + 0.557d + 18.3f + 0.00282v Predictor Coef SE Coef Constant -0.9723 0.3923 -2.48 0.015 -0.03117 0.01864 -1.67 0.099 d 0.5568 0.3185 1.75 0.084 18.2602 0.7536 24.23 0.000 0.002822 0.003977 0.71 0.480 S = 0.822059 Source R-Sq = 88.6 R-Sq (adj) = 88.04 DS MS 0.000 Regression Residual Error 4 401.02 100.25 148.35 76 51.36 0.68 Total 80 452.38 (a) How many observations were there in the data set? observations (b) Interpret the coefficient of multiple determination. O 8.0% of the observed variation in feed rate can be explained by the model relationship with vibration…arrow_forwardWe estimate a simple regression Grade-B,+B,Effort + u where the red line represents Qur estimate for Grade relative to Effort, and the dots represent our data points. Which OLS assumption is violated in this regression?arrow_forwardThe ols() method in statsmodels module is used to fit a multiple regression model using “Quality” as the response variable and “Speed” and “Angle” as the predictor variables. The output is shown below. A text version is available. What is the correct regression equation based on this output? What is the coefficient of determination? Select one.arrow_forward
- Can you help me answer this question pleasearrow_forwardWhich of the variables is the indepenent variable and dependent variable for the following question. fit a simple linear regression model to predict latitudes using average monthly range lat= latitudes range= the average monthly range between mean montly maximum and minimum temperatures for a selected set of US cities.arrow_forwardUse the given dataset*note: Gender takes on a value of 1 if the student is male, and 0 otherwise Estimate a linear regression model relating overall grade weighted average (OGWA) of student to their gender, available internet speed (mbps) and previous term’s grade weighted average (lgwa)a. Interpret the slope coefficients (discuss their values and statistical significance)b. Are the coefficients jointly statistically significant? Explain your answer.c. How much of the variability of the overall grade weighted average is explained by the variability of the model?arrow_forward
arrow_back_ios
arrow_forward_ios
Recommended textbooks for you
- Algebra & Trigonometry with Analytic GeometryAlgebraISBN:9781133382119Author:SwokowskiPublisher:CengageAlgebra and Trigonometry (MindTap Course List)AlgebraISBN:9781305071742Author:James Stewart, Lothar Redlin, Saleem WatsonPublisher:Cengage LearningCollege AlgebraAlgebraISBN:9781305115545Author:James Stewart, Lothar Redlin, Saleem WatsonPublisher:Cengage Learning
- Glencoe Algebra 1, Student Edition, 9780079039897...AlgebraISBN:9780079039897Author:CarterPublisher:McGraw HillElementary Linear Algebra (MindTap Course List)AlgebraISBN:9781305658004Author:Ron LarsonPublisher:Cengage LearningBig Ideas Math A Bridge To Success Algebra 1: Stu...AlgebraISBN:9781680331141Author:HOUGHTON MIFFLIN HARCOURTPublisher:Houghton Mifflin Harcourt
Algebra & Trigonometry with Analytic Geometry
Algebra
ISBN:9781133382119
Author:Swokowski
Publisher:Cengage
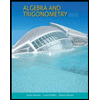
Algebra and Trigonometry (MindTap Course List)
Algebra
ISBN:9781305071742
Author:James Stewart, Lothar Redlin, Saleem Watson
Publisher:Cengage Learning
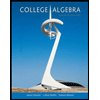
College Algebra
Algebra
ISBN:9781305115545
Author:James Stewart, Lothar Redlin, Saleem Watson
Publisher:Cengage Learning
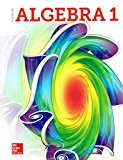
Glencoe Algebra 1, Student Edition, 9780079039897...
Algebra
ISBN:9780079039897
Author:Carter
Publisher:McGraw Hill
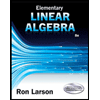
Elementary Linear Algebra (MindTap Course List)
Algebra
ISBN:9781305658004
Author:Ron Larson
Publisher:Cengage Learning
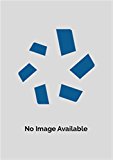
Big Ideas Math A Bridge To Success Algebra 1: Stu...
Algebra
ISBN:9781680331141
Author:HOUGHTON MIFFLIN HARCOURT
Publisher:Houghton Mifflin Harcourt