BIO259-Graphical-Data-Summaries_Tutorial
.pdf
keyboard_arrow_up
School
Toronto Metropolitan University *
*We aren’t endorsed by this school
Course
259
Subject
Statistics
Date
Apr 3, 2024
Type
Pages
31
Uploaded by DeanValorHornet7
BIO259-Graphical-Data-Summaries_Tutorial
October 16, 2023
Our ability to generate visually stimulating graphical representations that evoke appropriate re-
sponses from the audiences of our data is arguably the most important aspect of biological data
science. These graphical representations not only help us communicate our results to our audience,
but also enable us to gain greater insight into our own data through simplification.
By leveraging the power of computer programming, we can generate and manipulate figures in order
to produce an extremely wide range of plots that simply aren’t accessible through most graphic
user interfaces. We can also heavily parallelize our analyses and incorporate these visualizations
into pipelines that enable us to generate the same plots for different datasets.
In today’s tutorial we will learn how to generate several different types of graphical data summaries
in R using the
ggplot2
package.
We will also learn to perform some basic manipulations and
customize these plots where applicable. Our tutorial is divided into seven primary sections, each
of which will cover how to generate and customize a different type of plot:
1. Line Graphs
2. Bar Charts
3. Box and Whisker Plots (with dot plots)
4. Scatter Plots
5. Pie Charts
6. Histograms
7. Heat Maps
#1. Line typically used for time data
#2. Bar charts are good for looking at catagorical data and their numbers
#6. histogram y axis is typically count
#3. Look at the spread of your data
#4. Look at the relationship between 2 variables
#5. Visualize proportions or percentages
#7. infer visually correlation between 2 variables
[1]:
#Run the code block below in order to import our dataset, select a subset of
␣
,
→
the columns, and filter out undesirable location data.
#This will be the data that we will work with throughput this tutorial and in
␣
,
→
our practical.
library
(dplyr)
library
(ggplot2)
1
covid_df
<-
read.table
(
"/var/biojupyterhubdata/BIO259/owid-covid-data-15.06.
,
→
2022.csv"
, sep
=
","
, header
=
TRUE
, quote
=
""
)
covid_df
<-
covid_df
%>%
␣
,
→
select
(
c
(
"continent"
,
"location"
,
"date"
,
"total_cases"
,
"new_cases"
,
"total_deaths"
,
"new_deaths"
,
→
"total_cases_per_million"
,
"new_cases_per_million"
,
␣
,
→
"total_deaths_per_million"
,
"new_deaths_per_million"
,
"icu_patients"
,
␣
,
→
"icu_patients_per_million"
,
"hosp_patients"
,
"hosp_patients_per_million"
,
␣
,
→
"total_vaccinations"
,
"total_tests"
,
"new_tests"
,
"positive_rate"
,
␣
,
→
"total_vaccinations"
,
"people_vaccinated"
,
"people_fully_vaccinated"
,
␣
,
→
"total_boosters"
,
"new_vaccinations"
,
"population"
,
"population_density"
,
␣
,
→
"median_age"
,
"gdp_per_capita"
,
"diabetes_prevalence"
,
"life_expectancy"
))
covid_df
<-
covid_df
%>%
filter
(
!
location
%in%
c
(
'Africa'
,
'Asia'
,
'Europe'
,
␣
,
→
'North America'
,
'South America'
,
'Oceania'
,
'Low income'
,
'Lower middle
␣
,
→
income'
,
'Upper middle income'
,
'High income'
,
'European Union'
,
␣
,
→
'International'
,
'Northern Cyprus'
,
'World'
,
'Grand Total'
))
covid_df
<-
covid_df
%>%
mutate
(date
=
as.Date
(date, format
=
"%Y-%m-%d"
))
␣
,
→
#convert column to date object
covid_df
[is.na
(covid_df)]
= 0
#replace NA values with 0
Attaching package: ‘dplyr’
The following objects are masked from ‘package:stats’:
filter, lag
The following objects are masked from ‘package:base’:
intersect, setdiff, setequal, union
0.1
Part 1: Line Graphs
Line graphs are an effective means of displaying changes in a response variable through time. In
this section, we will use our Covid-19 dataset to plot the number of Sars-CoV-2 cases detected each
day through time in order to compare the pandemic curves for Canada and the USA.
[2]:
# Generate a line graph of daily cases (per million) in Canada using
# geom_line()
# The ggplot process is usually: create a data frame with the part of
#the data you want
# Then add a type of graph, with aesthetic parameters
#(such as values of x, values of y etc)
2
#colo(u)r will colour depending on the location column
# The answer should look like this, but with some 'corrections'
ggplot
(covid_df
%>%
filter
(location
==
"Canada"
))
+
#Create subset line graph
geom_line
(
aes
(x
=
date, y
=
new_cases_per_million, group
=
location,
␣
,
→
colour
=
location))
# Write your code here. Be careful to follow the instructions!
[3]:
# Compare line graph from Canada to one from the USA, normalizing for
␣
,
→
population. Note change in scale.
# The answer should look like this, but with some 'corrections'
3
ggplot
(covid_df
%>%
filter
(location
==
"Canada"
|
location
==
"United States"
))
␣
,
→
+
geom_line
(
aes
(x
=
date, y
=
new_cases_per_million,
group
=
location, colour
=
location))
# Write your code here. Be careful to follow the instructions!
[4]:
# Adjust your axes, legends, and colours to improve figure readability.
ggplot
(covid_df
%>%
filter
(location
==
"Canada"
|
location
==
"United States"
))
␣
,
→
+
geom_line
(
aes
(x
=
date, y
=
new_cases_per_million, group
=
location,
␣
,
→
colour
=
location))
+
4
theme
(axis.title
=
element_text
(size
=15
), axis.text
=
␣
,
→
element_text
(size
=15
), axis.ticks.length
=
unit
(
.1
,
"cm"
))
+
#each option in
␣
,
→
theme has several underlying options, here we are manipulating several
␣
,
→
aspects of our axes
xlab
(
"Date"
)
+
ylab
(
"New Cases Per Million"
)
+
#replace axis labels
theme
(legend.position
=
"bottom"
, legend.text
=
element_text
(size
=15
), legend.
,
→
title
=
element_blank
())
+
#manipulate legend
scale_color_manual
(values
=
c
(
"#ff0000"
,
"#0000FF"
))
#specify different
␣
,
→
"national" colours for each group
5
0.2
Part 2: Bar Charts
Bar charts utilize rectangular bars with lengths that are proportional to the values they represent
to compare categorical data.
Error bars are commonly added to bar charts to display variance.
In this section, we will compare the average number of hospitalized Covid-19 patients per million
across eight countries in Europe and learn how to include error bars on these plots.
[5]:
# We'll pivot our covid_df in order to calculate summary mean, sd, and sem
␣
,
→
values for subset of European countries.
# Our countries of interest are Belgium, France, Italy, Iceland, Netherlands,
␣
,
→
Portugal, Sweden, and the United Kingdom.
pivot_covid_df
<-
covid_df
%>%
group_by
(location)
%>%
filter
(location
==
␣
,
→
'Belgium'
|
location
==
'France'
|
location
==
'Italy'
|
location
==
␣
,
→
'Iceland'
|
location
==
'Netherlands'
|
location
==
'Portugal'
|
location
␣
,
→
==
'Sweden'
|
location
==
'United Kingdom'
)
%>%
summarise
(mean_hosp_patients
=
mean
(hosp_patients_per_million),
␣
,
→
sd_hosp_patients
=
sd
(hosp_patients_per_million), sem_hosp_patients
=
␣
,
→
sd
(hosp_patients_per_million)
/
sqrt
(
n
()))
pivot_covid_df
A tibble: 8 × 4
location
mean_hosp_patients
sd_hosp_patients
sem_hosp_patients
<chr>
<dbl>
<dbl>
<dbl>
Belgium
161.27678
133.90926
4.558324
France
244.34309
143.84840
4.865745
Iceland
36.31031
53.97307
1.863357
Italy
201.03494
177.41961
6.025486
Netherlands
59.19184
41.76282
1.440954
Portugal
110.12235
130.67054
4.516635
Sweden
87.08845
84.02470
2.855275
United Kingdom
136.17966
122.06000
4.145375
[6]:
# Generate a simple bar chart of mean hospitalized patients in
# each country. You can play around with geom_bar settings to see what they
␣
,
→
each do.
# Again the trick is to load the data to ggplot
# Then plot a bar graph with specific aesthetics
# geom_bar() can perform summaries for us, but we can also plot the pivot table
␣
,
→
directly.
# stat = "identity" we are giving it the y values to plot
#The answer should look like this, but with some 'corrections'
ggplot
(pivot_covid_df)
+
geom_bar
(
aes
(x
=
location, y
=
mean_hosp_patients), stat
=
"identity"
,
␣
,
→
fill
=
"gray"
, colour
=
"black"
, size
=1
, alpha
=1
)
# Write your code here. Be careful to follow the instructions!
6
Your preview ends here
Eager to read complete document? Join bartleby learn and gain access to the full version
- Access to all documents
- Unlimited textbook solutions
- 24/7 expert homework help
Related Questions
Define the process of mathematical modelling?
arrow_forward
Give an example of a refinement in mathematical modeling with contextual explanation.
arrow_forward
Define mathematical models.
arrow_forward
Discuss the importance of a model being well documented.
arrow_forward
What is the simultaneous equation bias? Give an example? What are the techniques used to estimate such model? What are the necessary conditions that are required to validly estimate the original models parameters?
arrow_forward
What connects both internal and external data in operations and supply chain analytics?
Ai
Danalytics
Teradata
Deep Learning.
arrow_forward
What is business analytics? Briefly describe the domain of the major fields of business analytics databases and data warehousing, descriptive, predictive, and prescriptive analytics.
arrow_forward
Alert dont submit AI generated answer.
arrow_forward
plz solve question (b) with explanation within 30-40 mins and get upvotes.
arrow_forward
What does a Model mean? Discuss FIVE characteristics of a Model.
arrow_forward
All analysis, calculations, and explanations must be done in a single Excel file (use separate Excel sheets for each question). Upload the completed Excel file using the file extension format Lastname_Firstname_RegressionProblem.
Regression Problem
Sarah Anderson, the business analyst at TV Revolution, is conducting research on the dealership’s various television brands. She has collected data over the past year (2022) on the manufacturer, screen size, and price of various television brands. The data is given in the file below.
You have been hired as an intern to run analyses on the data and report the results back to Sarah; the five questions that Sarah needs you to address are given below.
Does there appear to be a positive or negative relationship between price and screen size? Use a scatter plot to examine the relationship.
Determine and interpret the correlation coefficient between the two variables. In your interpretation, discuss the direction of the relationship (positive,…
arrow_forward
Differentiate between nominal data and ordinal data.
Give at least two examples of nominal and ordinal data.
arrow_forward
Conditions for consistency of Data.
arrow_forward
What is the main purpose of data presentation? Is it applicable for our daily lives? Give specific circumstance that you used data presentation in a real life situation.
arrow_forward
Why is it a critical and challenging part of the model-building process to determine the appropriate values?
arrow_forward
I need help please!
Describe the culture in America during the 1950s through 1970s. How did this culture influence mathematics education? What were the major shifts and changes that occurred during this time? How is it different from today's mathematics education?
arrow_forward
The Ministry of Tourism in Trinidad and Tobago is interested in developing a campaign to increase the number of visitors to the island. The Ministry in collaboration with the island’s hotels collected data to be used as a guide to determine what steps should be taken going forward. Using the data in the Microsoft Excel file attached you are required to use the knowledge you have acquired during the semester to answer the following question. Ensure that your responses are detailed and all the necessary steps are clearly outlined.
Derive a model for the estimation of the probability of returning to the island from the average money spent during the visit.
Discuss why regression analysis is important in decision-making.
arrow_forward
Bayesian modelling and analysis as a project. What are the basics I should include in my work?
arrow_forward
Define and draw unit circle. What is the significance of unit circle for system analysis?
arrow_forward
The quadratic model for the given data is wrong.
arrow_forward
What is model breakdown?
arrow_forward
Based on your learning. How does empowerment technlogy affects your learning process, and how does it empowers students in terms of thinking, communicating, socializing and integrating technology in everyday life?
arrow_forward
College GPA and Salary. Do students with higher college grade point averages (GPAs) earn more than those graduates with lower GPAs (CivicScience)? Consider the college GPA and salary data (10 years after graduation) provided in the file GPASalary.
Develop a scatter diagram for these data with college GPA as the independent variable. PLEASE MAKE SIMPLE GRAPH. What does the scatter diagram indicate about the relationship between the two variables?
Use these data to develop an estimated regression equation that can be used to predict annual salary 10 years after graduation given college GPA.
At the .05 level of significance, does there appear to be a significant statistical relationship between the two variables?
GPA
Salary
2.21
71000
2.28
49000
2.56
71000
2.58
63000
2.76
87000
2.85
97000
3.11
134000
3.35
130000
3.67
156000
3.69
161000
arrow_forward
plz solve question (c) with explanation within 30-40 mins and get upvotes
arrow_forward
SEE MORE QUESTIONS
Recommended textbooks for you
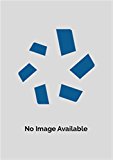
Big Ideas Math A Bridge To Success Algebra 1: Stu...
Algebra
ISBN:9781680331141
Author:HOUGHTON MIFFLIN HARCOURT
Publisher:Houghton Mifflin Harcourt
Algebra & Trigonometry with Analytic Geometry
Algebra
ISBN:9781133382119
Author:Swokowski
Publisher:Cengage
Related Questions
- Discuss the importance of a model being well documented.arrow_forwardWhat is the simultaneous equation bias? Give an example? What are the techniques used to estimate such model? What are the necessary conditions that are required to validly estimate the original models parameters?arrow_forwardWhat connects both internal and external data in operations and supply chain analytics? Ai Danalytics Teradata Deep Learning.arrow_forward
- What is business analytics? Briefly describe the domain of the major fields of business analytics databases and data warehousing, descriptive, predictive, and prescriptive analytics.arrow_forwardAlert dont submit AI generated answer.arrow_forwardplz solve question (b) with explanation within 30-40 mins and get upvotes.arrow_forward
- What does a Model mean? Discuss FIVE characteristics of a Model.arrow_forwardAll analysis, calculations, and explanations must be done in a single Excel file (use separate Excel sheets for each question). Upload the completed Excel file using the file extension format Lastname_Firstname_RegressionProblem. Regression Problem Sarah Anderson, the business analyst at TV Revolution, is conducting research on the dealership’s various television brands. She has collected data over the past year (2022) on the manufacturer, screen size, and price of various television brands. The data is given in the file below. You have been hired as an intern to run analyses on the data and report the results back to Sarah; the five questions that Sarah needs you to address are given below. Does there appear to be a positive or negative relationship between price and screen size? Use a scatter plot to examine the relationship. Determine and interpret the correlation coefficient between the two variables. In your interpretation, discuss the direction of the relationship (positive,…arrow_forwardDifferentiate between nominal data and ordinal data. Give at least two examples of nominal and ordinal data.arrow_forward
arrow_back_ios
SEE MORE QUESTIONS
arrow_forward_ios
Recommended textbooks for you
- Big Ideas Math A Bridge To Success Algebra 1: Stu...AlgebraISBN:9781680331141Author:HOUGHTON MIFFLIN HARCOURTPublisher:Houghton Mifflin HarcourtAlgebra & Trigonometry with Analytic GeometryAlgebraISBN:9781133382119Author:SwokowskiPublisher:Cengage
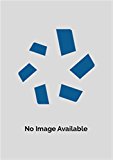
Big Ideas Math A Bridge To Success Algebra 1: Stu...
Algebra
ISBN:9781680331141
Author:HOUGHTON MIFFLIN HARCOURT
Publisher:Houghton Mifflin Harcourt
Algebra & Trigonometry with Analytic Geometry
Algebra
ISBN:9781133382119
Author:Swokowski
Publisher:Cengage