MGMT 305 Assignment 3 Gabriel Chang
pdf
keyboard_arrow_up
School
Purdue University *
*We aren’t endorsed by this school
Course
305
Subject
Statistics
Date
Jan 9, 2024
Type
Pages
18
Uploaded by BailiffTurtle4356
Chapter 16
Exercise 1
A.
Regression Statistics
Multiple R
0.794631
6
R Square
0.631439
37
Adjusted R
Square
0.539299
22
Standard Error
7.269276
44
Observations
6
ANOVA
df
SS
MS
F
Significanc
e F
Regression
1
362.13048
362.1304
8
6.853031
23
0.0589334
4
Residual
4
211.36952
52.84238
Total
5
573.5
Coefficie
nts
Standard
Error
t Stat
P-value
Lower 95%
Upper
95%
Lower
95.0%
Upper
95.0%
Intercept
-6.77453
03
14.1709475
-0.47805
77
0.657562
78
-46.119388
32.57032
75
-46.11938
8
32.570327
5
x
1.229645
09
0.46971932
2.617829
49
0.058933
44
-0.0745048
2.533795
01
-0.074504
8
2.5337950
1
Regression Equation:
Y = -6.7745 + 1.2296x
B.
H0: There is no significant relationship between X & Y
Ha: There is a significant relationship between X & Y
P-value = 0.0589 > alpha = 0.05
Do not reject H0 and conclude that that there is no significant relationship between X & Y.
C.
Scatterplot suggests that the data is positively correlated
D.
SUMMARY OUTPUT
Regression Statistics
Multiple R
0.97201226
R Square
0.94480783
Adjusted R Square 0.90801305
Standard Error
3.24821546
Observations
6
ANOVA
df
SS
MS
F
Significance F
Regression
2
541.847289
270.923644 25.6777668 0.01296631
Residual
3
31.652711
10.5509037
Total
5
573.5
Coefficients Standard Error
t Stat
P-value
Lower 95%
Upper 95% Lower 95.0% Upper 95.0%
Intercept
-168.88484
39.7861933
-4.2448103
0.02394868 -295.50227
-42.267418
-295.50227
-42.267418
x
12.1870135 2.66323606
4.57601702 0.01958678 3.71140777
20.6626193 3.71140777
20.6626193
x^2
-0.1770358
0.04289548
-4.127143
0.02579792 -0.3135483
-0.0405232
-0.3135483
-0.0405232
Regression Equation:
Y = -168.8848 + 12.1870x - 0.1770x
2
E.
H0: Relationship between x, x
2
, and y are insignificant
Ha: Relationship between x, x
2
, and y are significant
T.S: F = 25.6777
P-value = 0.0129 < alpha - 0.05
Reject H0, conclude that the relationship between variables x, x
2
, and y are significant.
F.
Given x = 25
Y = -168.8848 + 12.1870(25) - 0.1770(25
2
)
= 25.1652
Your preview ends here
Eager to read complete document? Join bartleby learn and gain access to the full version
- Access to all documents
- Unlimited textbook solutions
- 24/7 expert homework help
Exercise 27
A.
The scatter plot approximately shows that the weight and price variables do not have a strong
relationship, with many of the data points plotted in a curved line. As such, a simple linear
regression model wouldn’t be appropriate.
B.
SUMMARY OUTPUT
Regression Statistics
Multiple R
0.87761985
R Square
0.77021661
Adjusted R Square 0.74149369
Standard Error
242.80435
Observations
19
ANOVA
df
SS
MS
F
Significance F
Regression
2
3161747.29
1580873.64 26.8153971 7.7723E-06
Residual
16
943263.237
58953.9523
Total
18
4105010.53
Coefficients Standard Error
t Stat
P-value
Lower 95%
Upper 95% Lower 95.0% Upper 95.0%
Intercept
11375.9988 2565.18531
4.43476687 0.00041612 5938.0489
16813.9488 5938.0489
16813.9488
Weight
-728.33455 193.68095
-3.7604862 0.0017095
-1138.9198
-317.74928 -1138.9198
-317.74928
Weight^2
11.9737375 3.53910869
3.38326357 0.00379172 4.47116223
19.4763127 4.47116223
19.4763127
Regression Equation:
Y = 11375.9988 - 728.33456x + 11.9737x
2
C.
SUMMARY OUTPUT
Regression Statistics
Multiple R
0.84691921
R Square
0.71727214
Adjusted R Square 0.68193116
Standard Error
269.327964
Observations
19
ANOVA
df
SS
MS
F
Significance F
Regression
2
2944409.69
1472204.85 20.2957614 4.0827E-05
Residual
16
1160600.83
72537.5521
Total
18
4105010.53
Coefficients Standard Error
t Stat
P-value
Lower 95%
Upper 95% Lower 95.0% Upper 95.0%
Intercept
1283.75
95.2218148
13.4816796 3.7432E-10 1081.88877
1485.61123 1081.88877
1485.61123
Fitness
-571.75
153.540563
-3.7237717 0.00184727 -897.24145
-246.25855 -897.24145
-246.25855
Comfort
-907.08333 145.453725
-6.2362331 1.1897E-05 -1215.4315
-598.73521 -1215.4315
-598.73521
Regression Equation:
Y = 1283.75 - 571.75(Fitness) - 907.08(Comfort)
Part
B.
Adjusted R
2
= 0.7415
Part
C.
Adjusted R
2
= 0.6819
Thus, the regression equation obtained in Part
B.
is better than the equation obtained in Part
C.
D.
SUMMARY OUTPUT
Regression Statistics
Multiple R
0.9167757
R Square
0.8404776
8
Adjusted R
Square
0.7791229
4
Standard Error
224.43789
6
Observations
19
ANOVA
df
SS
MS
F
Significance
F
Regression
5
3450169.73
690033.94
6
13.698659
8
8.5356E-05
Residual
13
654840.797
50372.369
Total
18
4105010.53
Your preview ends here
Eager to read complete document? Join bartleby learn and gain access to the full version
- Access to all documents
- Unlimited textbook solutions
- 24/7 expert homework help
Coefficien
ts
Standard
Error
t Stat
P-value
Lower 95%
Upper
95%
Lower
95.0%
Upper
95.0%
Intercept
5923.5443 1546.52226
3.8302354
1
0.0020842
1
2582.48609
9264.6025
1
2582.48609 9264.60251
Fitness
-6343.250
2
2596.16097
-2.443319
3
0.0295819
3
-11951.915
-734.5854 -11951.915 -734.5854
Comfort
-7232.062
8
2518.19339
-2.871925
1
0.0130946
8
-12672.289
-1791.836
8
-12672.289 -1791.8368
Weight*Comfort
266.40881
4
93.9802425
2.8347321
4
0.0140625 63.3768436
469.44078
4
63.3768436 469.440784
Weight*Fitness
261.32166
8
111.842303
2.3365190
2
0.0361219
2
19.7010622
502.94227
4
19.7010622 502.942274
Weight
-214.5569
6
71.4212811
-3.004104
1
0.0101584 -368.85326
-60.26066
5
-368.85326 -60.260665
Regression Equation:
Y = 5923.5443 - 6343.2502(Fitness) - 7232.0682(Comfort) + 266.4088(Weight*Comfort) +
261.3217(Weight*Fitness) - 214.5570(Weight)
Part
B.
Adjusted R
2
= 0.7415
Part
C.
Adjusted R
2
= 0.6819
Part
D.
Adjusted R
2
= 0.7791
This Regression Equation provides the highest Adjusted R
2
compared to the equation obtained
from previous parts. Therefore, this equation is the best predictor of Price.
Additional Exercises
1. Backward Elimination
A.
Scrambling will not be in the final model as its p-value = 0.149 exceeds the alpha
threshold at 0.05.
B.
Model 1:
Adjusted R-square = 18.34%
Multiple R-square: 21.64%
Model 2:
Adjusted R-square = 19.17%
Multiple R-square: 21.77%
Model 3:
Adjusted R-square = 19.40%
Multiple R-square: 21.40%
Therefore, Model 1 has the lowest Adjusted R-square and Model 3 has the lowest
Multiple R-square.
C. No, since the p-value for DrDist (at 0.448) is still higher than the new alpha = 0.32. Other
predictors will still be included as they still have a p-value less than the new alpha.
2. Best Subsets
Statement 2 – TRUE:
Win% vs 20+ has the 2nd lowest AIC value.
Statement 3 – TRUE:
40+R has a T-score > 1.
3. Categorical Predictors
A.
Fit = 70.09 + 0.58*35 - 3.06*1 + 0.52*1 = 87.85
B.
Every time it is a Seafood/Steakhouse-type restaurant, the score decreases by 3.06
C. The higher the average meal price, the higher the score; for every increase of a dollar in
average meal price, the rating goes up by 0.58.
D. Slope of the variable remains the same but the coefficient reverses; slope of the Italian
predictor = 3.06
4. CEO Salaries
A.
SUMMARY OUTPUT
Regression Statistics
Multiple R
0.82729329
R Square
0.68441418
Adjusted R Square
0.67126477
Standard Error
901.047754
Observations
26
ANOVA
df
SS
MS
F
Signific
anc
e F
Regression
1
42257945.3
42257945.3
52.0490444
1.8692E-07
Residual
24
19485289.3
811887.054
Total
25
61743234.6
Coeffi
ci
e
nt
s
Standar
d
Erro
r
t Stat
P-val
u
e
Lower
95
%
Uppe
r
9
5
%
Lower
95.
0%
Upper
95.
0%
Intercept
852.858741
200.465871
4.25438373
0.00027656
439.117518
1266.59996
439.117518
1266.59996
Sales ($Mil.)
0.0688151
0.00953844
7.21450236
1.8692E-07
0.04912873
0.08850148
0.04912873
0.08850148
Correlation
= 0.8273
B.
SUMMARY OUTPUT
Regression Statistics
Multiple R
0.92145649
R Square
0.84908206
Adjusted R Square
0.84279381
Standard Error
0.15231861
Observations
26
ANOVA
df
SS
MS
F
Signific
anc
e F
Regression
1
3.13275176
3.13275176
135.026822
2.4255E-11
Residual
24
0.55682302
0.02320096
Total
25
3.68957478
Coeffi
ci
e
nt
s
Standar
d
Erro
r
t Stat
P-val
u
e
Lower
95
%
Uppe
r
9
5
%
Lower
95.
0%
Upper
95.
0%
Intercept
1.65692143
0.1208026
13.7159419
7.4931E-13
1.40759712
1.90624574
1.40759712
1.90624574
Log Sales
0.41397085
0.0356254
11.6201042
2.4255E-11
0.34044364
0.48749807
0.34044364
0.48749807
Your preview ends here
Eager to read complete document? Join bartleby learn and gain access to the full version
- Access to all documents
- Unlimited textbook solutions
- 24/7 expert homework help
Correlation
= 0.9215
C. Part A. and B. does not have equal correlation as the logarithmic transformation in Part
B. does not preserve the linear characteristic of the original function.
D. *
Required outputs have been shown in Part A & B respectively.
The regression analysis shows that the residuals are less in the logarithmic scale
compared to the normal scale. This shows that there is a better linear relationship when
using the logarithmic scale.
E.
Normal Scale:
CEO Pay = 852.8587 + 0.0688(Sales)
= 852.8587 + 0.0688*7billion
= 481,600,852.86
Logarithmic Scale:
CEO Pay = 1.6569 + 0.4140*7billion
= 2,898,000,001.66
F.
XYZ should choose residuals closer to 0. In such a case, XYZ should choose the
logarithmic model.
5. Logistic Regression
A.
P(1) = (-3.623 + 0.0491*45 + 1.605) / [1+(-3.623 + 0.0491*45+1.605)] = 0.1607
B.
P(1) = -3.623 + 0.0491*45 / [1+(-3.623 + 0.0491*45)] = 3.4184
C. We must multiply the odds in part B by 1.605 to get the odds in part A.
D. For the income variable, for every unit increase of household income, the odds of
purchasing a cereal would increase by exp(0.0491) when ViewAd remains the same. For
the ViewAd variable; if the shopper watches an add, then the probability of purchasing a
cereal would increase by exp(1.605) when income remains the same
E.
The ViewAd_Yes variable is significant because its coefficient of 1.605 lies within the C.I
of (1.4855, 16.6835).
Interaction Effects
A.
Gambling Amount($) = 30.1 - 68.2 Sex - 0.481 Status + 1.088 Sex:Status + 4.970
Income
= 30.1 - 68.2(1) - 0.481(70) + 1.088(1*70) + 4.970(3.5)
= 21.785
B.
= 30.1 - 68.2(1) - 0.481(80) + 1.088(1*80) + 4.970(3.5)
= 27.855
C.
= 30.1 - 68.2(0) - 0.481(70) + 1.088(0) + 4.970(3.5)
= 13.825
D.
= 30.1 - 68.2(0) - 0.481(80) + 1.088(0) + 4.970(3.5)
= 9.015
E.
For females, through the sex:status variable, we can see that there is an increased effect
of status on gambling amount. Therefore, for every 1-unit increase in status, there is an
increase of $0.607 ($1.088 - $0.481) in gambling amount. As such, we can surmise that
for females, with all else constant, the higher the status level, the higher the gambling
amount will be. Different from males, with all else constant, the higher the status level,
the lower the gambling amount will be since the sex:status variable wouldn’t be applied.
Therefore, for every 1-unit increase in status, the gambling amount will be decreased by
0.481.
Trend and Lag Modeling
A.
SUMMARY OUTPUT
Regression Statistics
Multiple R
0.97176371
R Square
0.94432471
Adjusted R
Square
0.94246887
Standard Error
17.3943043
Observations
32
ANOVA
df
SS
MS
F
Significan
ce F
Regression
1
153955.165
153955.16
5
508.83870
6
2.2716E-20
Residual
30
9076.85461
302.56182
Total
31
163032.02
Coeffici
en
ts
Standard
Error
t Stat
P-valu
e
Lower
95%
Upper
9
5
%
Lower
95.
0%
Upper
95.
0%
Intercept
93.9995968 6.29684354
14.928050
2
1.9922E-15 81.1397267
106.859467 81.1397267
106.859467
Trend
7.51233504 0.33303117
22.557453
4
2.2716E-20 6.83219466
8.19247542 6.83219466
8.19247542
Regression Equation:
Y = 93.9996 + 7.5123(Trend)
SUMMARY OUTPUT
Regression Statistics
Multiple R
0.98578672
R Square
0.97177546
Adjusted R
Square
0.9708022
Standard Error
12.2841678
Observations
31
ANOVA
df
SS
MS
F
Significan
ce F
Regression
1
150670.605
150670.60
5
998.47466
5
5.0921E-24
Residual
29
4376.12258
150.90077
9
Total
30
155046.727
Coeffici
en
ts
Standard
Error
t Stat
P-valu
e
Lower
95%
Upper
9
5
%
Lower
95.
0%
Upper
95.
0%
Intercept
3.50603991 7.22165849
0.4854895
7
0.6309769
4
-11.26391
18.2759899 -11.26391
18.2759899
Lag 1
1.01632759 0.03216364
31.598649
7
5.0921E-24 0.95054556
1.08210962 0.95054556
1.08210962
Regression Equation:
Y = 3.5060 + 1.0163 (Lag1)
B.
Price vs Lag1 R-Square = 0.9718
Price vs Trend R-Square = 0.9443
Using the Price vs Lag1 Model is more accurate
C. Lag1, 2021 = 342.9595
Lag1, 2022 = 356.0701
Trend, 2021 = 334.3943
Trend, 2022 = 341.9067
Your preview ends here
Eager to read complete document? Join bartleby learn and gain access to the full version
- Access to all documents
- Unlimited textbook solutions
- 24/7 expert homework help
Chapter 17
Exercise 5
A.
A horizontal pattern exists in the data.
B.
Week
Value
Forecast
Forecast Error
Squared FE
1
18
2
13
3
16
4
11
15.6666667 -4.666666667
21.7777778
5
17
13.3333333 3.666666667
13.4444444
6
14
14.6666667 -0.666666667
0.44444444
Total
35.6666667
MSE
11.8888889
Forecast Week 7
14
C.
Week
Value
Forecast
Forecast Error
Squared FE
1
18
2
13
18
-5
25
3
16
17
-1
1
4
11
16.8
-5.8
33.64
5
17
15.64
1.36
1.85
6
14
15.91
-1.91
3.65
Total
65.14
MSE
13.03
Forecast Week 7
15.53
D.
The 3-week moving average provides a better forecast as its MSE is lower at 11.89 compared to
the smoothing forecast using a = 0.2 which results in a higher MSE at 13.03.
E.
Week
Value
Forecast
Forecast Error Squared FE
1
18
2
13
18
-5
25
3
16
16
0
0
4
11
16
-5
25
5
17
14
3
9.00
6
14
15.2
-1.2
1.44
Total
60.44
MSE
12.09
A smoothing constant of a = 0.4 results in a lower MSE of 12.09, thus providing a more accurate
forecast.
Exercise 11
A.
A horizontal pattern exists in the data.
B.
Three-month Moving Average
Month
Percentage ForecastFE
FE^2
1
80
2
82
3
84
4
83
82.00
1.00
1.00
5
83
83.00
0.00
0.00
6
84
83.33
0.67
0.44
7
85
83.33
1.67
2.78
Your preview ends here
Eager to read complete document? Join bartleby learn and gain access to the full version
- Access to all documents
- Unlimited textbook solutions
- 24/7 expert homework help
8
84
84.00
0.00
0.00
9
82
84.33
-2.33
5.44
10
83
83.67
-0.67
0.44
11
84
83.00
1.00
1.00
12
83
83.00
0.00
0.00
Total
11.11
MSE
1.23
Smoothing a = 0.2
Month
Percentage ForecastFE
FE^2
1
80
2
82
80
2.00
4.00
3
84
80
3.60
12.96
4
83
81
1.88
3.53
5
83
81
1.50
2.26
6
84
82
2.20
4.85
7
85
82
2.76
7.63
8
84
83
1.21
1.46
9
82
83
-1.03
1.06
10
83
83
0.17
0.03
11
84
83
1.14
1.30
12
83
83
-0.09
0.01
Total
39.11
MSE
3.56
The 3-month moving average provides a lower and therefore more accurate MSE at 1.23.
C.
Forecast for next month = (83 + 84 + 83) / 3 = 83.33
Exercise 25
A.
An upward, linear pattern exists in the data.
B.
Linear Equation: y = 16.779x + 11.465
C.
Quadratic Line Equation: y = 1.563x
2
+ 5.842x + 26.048
D.
Linear MSE = 15.3
Quadratic MSE = 0.11
The Quadratic Model is better due to its lower MSE.
E.
@period = 7
Using Linear, y = $128.9 million
Using Quadratic, y = $143.5 million
F.
I would use the forecast using the Quadratic model as it would provide a more accurate data
due to its lower MSE as calculated in part D.
Extra Credit
Internship Dataset
Full-time Job
No Full-time Job
Total
No Internship
458
316
774
1 Internship
600
87
687
2 Internship
319
11
330
Total
1377
414
1791
A student who did 1 internship before graduation increases their probability of getting a full-time
job before or within 6 months of graduation by (600/687 - 458/774) * 100% = 19.25%.
Research question:
Maximum Likelihood Estimation, used to estimate the coefficients in logistic regression.
Your preview ends here
Eager to read complete document? Join bartleby learn and gain access to the full version
- Access to all documents
- Unlimited textbook solutions
- 24/7 expert homework help
Related Documents
Recommended textbooks for you
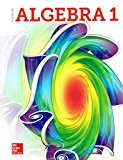
Glencoe Algebra 1, Student Edition, 9780079039897...
Algebra
ISBN:9780079039897
Author:Carter
Publisher:McGraw Hill
Recommended textbooks for you
- Glencoe Algebra 1, Student Edition, 9780079039897...AlgebraISBN:9780079039897Author:CarterPublisher:McGraw Hill
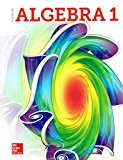
Glencoe Algebra 1, Student Edition, 9780079039897...
Algebra
ISBN:9780079039897
Author:Carter
Publisher:McGraw Hill