2. Independent parameters. How many independent parameters are required to uniquely define the CPD of E (the conditional probability distribution associated with the variable E) in the same graphical model as above, if A, B, and D are binary, and C and E have three values each? A B E If you haven't come across the term before, here's a brief explanation: A multinomial distribution over m possibilities 1,...,m has m parameters, but m-1 independent parameters, because we have the constraint that all parameters must sum to 1, so that if you specify m-1 of the parameters, the final one is fixed. In a CPD P(XY), if X has m values and Y has k values, then we have k distinct multinomial distributions, one for each value of Y, and we have m-1 independent parameters in each of them, for a total of k(m-1). More generally, in a CPD P(X|Y₁,..., Y), if each Y; has kį values, we have a total of k₁ X... x kr x (m-1) independent parameters. Example: Let's say we have a graphical model that just had X→Y, where both variables are binary. In this scenario, we need 1 parameter to define the CPD of X. The CPD of X contains two entries P(X = 0) and P(X = 1). Since the sum of these two entries has to be equal to 1, we only need one parameter to define the CPD. D Now we look at Y. The CPD for Y contains 4 entries which correspond to: P(Y=0|X = 0), P(Y = 1|X = 0), P(Y=0|X = 1), P(Y = 1|X = 1). Note that P(Y=0|X = 0) and P(Y = 1 X = 0) should sum to one, so we need 1 independent parameter to describe those two entries; likewise, P(Y=0|X = 1) and P(Y= 1|X = 1) should also sum to 1, so we need 1 independent parameter for those two entries. Therefore, we need 1 independent parameter to define the CPD of X and 2 independent parameters to define the CPD of Y. 18 11 12
2. Independent parameters. How many independent parameters are required to uniquely define the CPD of E (the conditional probability distribution associated with the variable E) in the same graphical model as above, if A, B, and D are binary, and C and E have three values each? A B E If you haven't come across the term before, here's a brief explanation: A multinomial distribution over m possibilities 1,...,m has m parameters, but m-1 independent parameters, because we have the constraint that all parameters must sum to 1, so that if you specify m-1 of the parameters, the final one is fixed. In a CPD P(XY), if X has m values and Y has k values, then we have k distinct multinomial distributions, one for each value of Y, and we have m-1 independent parameters in each of them, for a total of k(m-1). More generally, in a CPD P(X|Y₁,..., Y), if each Y; has kį values, we have a total of k₁ X... x kr x (m-1) independent parameters. Example: Let's say we have a graphical model that just had X→Y, where both variables are binary. In this scenario, we need 1 parameter to define the CPD of X. The CPD of X contains two entries P(X = 0) and P(X = 1). Since the sum of these two entries has to be equal to 1, we only need one parameter to define the CPD. D Now we look at Y. The CPD for Y contains 4 entries which correspond to: P(Y=0|X = 0), P(Y = 1|X = 0), P(Y=0|X = 1), P(Y = 1|X = 1). Note that P(Y=0|X = 0) and P(Y = 1 X = 0) should sum to one, so we need 1 independent parameter to describe those two entries; likewise, P(Y=0|X = 1) and P(Y= 1|X = 1) should also sum to 1, so we need 1 independent parameter for those two entries. Therefore, we need 1 independent parameter to define the CPD of X and 2 independent parameters to define the CPD of Y. 18 11 12
Algebra & Trigonometry with Analytic Geometry
13th Edition
ISBN:9781133382119
Author:Swokowski
Publisher:Swokowski
Chapter10: Sequences, Series, And Probability
Section10.8: Probability
Problem 29E
Related questions
Question
J 2
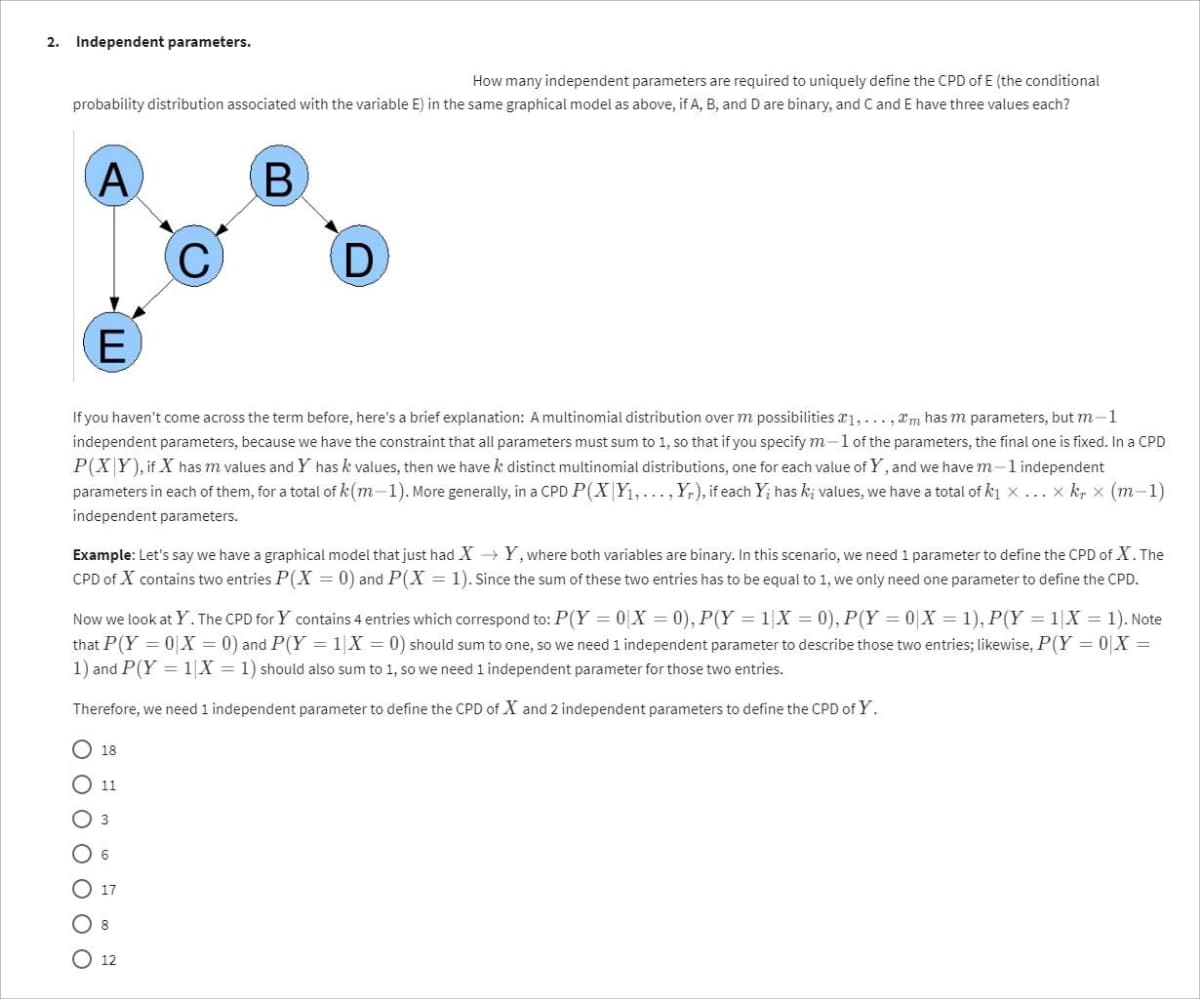
Transcribed Image Text:2. Independent parameters.
How many independent parameters are required to uniquely define the CPD of E (the conditional
probability distribution associated with the variable E) in the same graphical model as above, if A, B, and D are binary, and C and E have three values each?
A
B
E
If you haven't come across the term before, here's a brief explanation: A multinomial distribution over m possibilities 1,...,m has m parameters, but m-1
independent parameters, because we have the constraint that all parameters must sum to 1, so that if you specify m-1 of the parameters, the final one is fixed. In a CPD
P(XY), if X has m values and Y has k values, then we have k distinct multinomial distributions, one for each value of Y, and we have m-1 independent
parameters in each of them, for a total of k(m-1). More generally, in a CPD P(X|Y₁,..., Yr), if each Y; has kį values, we have a total of k₁ ×... x kr x (m−1)
independent parameters.
Example: Let's say we have a graphical model that just had X→Y, where both variables are binary. In this scenario, we need 1 parameter to define the CPD of X. The
CPD of X contains two entries P(X= 0) and P(X = 1). Since the sum of these two entries has to be equal to 1, we only need one parameter to define the CPD.
Now we look at Y. The CPD for Y contains 4 entries which correspond to: P(Y=0|X=0), P(Y = 1 X = 0), P(Y=0|X = 1), P(Y= 1|X = 1). Note
that P(Y=0|X = 0) and P(Y= 1 X = 0) should sum to one, so we need 1 independent parameter to describe those two entries; likewise, P(Y=0 X =
1) and P(Y = 1|X = 1) should also sum to 1, so we need 1 independent parameter for those two entries.
Therefore, we need 1 independent parameter to define the CPD of X and 2 independent parameters to define the CPD of Y.
0 0 0 0 0 0
D
18
11
12
Expert Solution

This question has been solved!
Explore an expertly crafted, step-by-step solution for a thorough understanding of key concepts.
Step by step
Solved in 2 steps with 1 images

Recommended textbooks for you
Algebra & Trigonometry with Analytic Geometry
Algebra
ISBN:
9781133382119
Author:
Swokowski
Publisher:
Cengage
Algebra & Trigonometry with Analytic Geometry
Algebra
ISBN:
9781133382119
Author:
Swokowski
Publisher:
Cengage