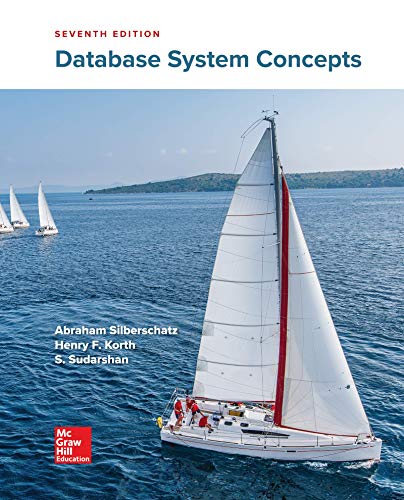
Database System Concepts
7th Edition
ISBN: 9780078022159
Author: Abraham Silberschatz Professor, Henry F. Korth, S. Sudarshan
Publisher: McGraw-Hill Education
expand_more
expand_more
format_list_bulleted
Question
I am trying to solve this but getting stuck into an infinity loop. Can someone please help me resolve this code.
![Logistic regression: iterative algorithm
The algorithm alternates between the following four steps untol convergence
1. Estimate s; = o(a¹ x); i = 1,.., Nsamples
2. Evaluate the error e = s, -y, where y, are the true labels
3. Evaluate the gradient g =
X(S₁ - y₁)
4. Update a → a- yG using gradient descent with a step size g
Here, x, are the vectorized digits of dimension 784 x 1. X; are vectors of length 785 x 1, obtained by adding a 1 to the end. Note that the above operations
can be computed efficiently in the matrix form as
1. Estimate s = o(a¹ X), where s is a 1xN matrix.
2. Evaluate the error e = s-y
3. Evaluate the gradient X(s - y)
4. Update a → a- yG using gradient descent with a step size g
Complete the code below
Nsamples, Nfeatures = X_train.shape
Nclasses = 2
a = np.random.randn(Nfeatures+1,Nclasses-1)
Xtilde = np.concatenate((X_train, np.ones ((Nsamples,1))),axis=1). T
gamma = 1e-1
for iter in range(1500):
z = np.dot(a.T,Xtilde)
y_pred = sigmoid(z)
error = y_train - y_pred. T
gradient = -np.dot (Xtilde, error)/Nsamples
a = a - gamma* gradient
if(np.mod (iter, 100)==0):
print("Error
fig, ax = plt.subplots (1,2)
ax[0].plot(s[:,0:200].T)
ax[0].plot(y_train [0:200])
ax[0].set_title('True and predicted labels')
ax[1].plot(error)
ax[1].set_title('Prediction Errors')
plt.show()
=
", np.sum(error**2))
plt.imshow(np.reshape(a[:-1], (28,28)))
plt.title("weights")](https://content.bartleby.com/qna-images/question/a5602bda-7b39-4a2b-a1a8-06efc64b3411/3607c1fb-3d39-4e7a-b371-572eefff4d32/irg64yj_thumbnail.png)
Transcribed Image Text:Logistic regression: iterative algorithm
The algorithm alternates between the following four steps untol convergence
1. Estimate s; = o(a¹ x); i = 1,.., Nsamples
2. Evaluate the error e = s, -y, where y, are the true labels
3. Evaluate the gradient g =
X(S₁ - y₁)
4. Update a → a- yG using gradient descent with a step size g
Here, x, are the vectorized digits of dimension 784 x 1. X; are vectors of length 785 x 1, obtained by adding a 1 to the end. Note that the above operations
can be computed efficiently in the matrix form as
1. Estimate s = o(a¹ X), where s is a 1xN matrix.
2. Evaluate the error e = s-y
3. Evaluate the gradient X(s - y)
4. Update a → a- yG using gradient descent with a step size g
Complete the code below
Nsamples, Nfeatures = X_train.shape
Nclasses = 2
a = np.random.randn(Nfeatures+1,Nclasses-1)
Xtilde = np.concatenate((X_train, np.ones ((Nsamples,1))),axis=1). T
gamma = 1e-1
for iter in range(1500):
z = np.dot(a.T,Xtilde)
y_pred = sigmoid(z)
error = y_train - y_pred. T
gradient = -np.dot (Xtilde, error)/Nsamples
a = a - gamma* gradient
if(np.mod (iter, 100)==0):
print("Error
fig, ax = plt.subplots (1,2)
ax[0].plot(s[:,0:200].T)
ax[0].plot(y_train [0:200])
ax[0].set_title('True and predicted labels')
ax[1].plot(error)
ax[1].set_title('Prediction Errors')
plt.show()
=
", np.sum(error**2))
plt.imshow(np.reshape(a[:-1], (28,28)))
plt.title("weights")
Expert Solution

This question has been solved!
Explore an expertly crafted, step-by-step solution for a thorough understanding of key concepts.
This is a popular solution
Trending nowThis is a popular solution!
Step by stepSolved in 4 steps with 4 images

Knowledge Booster
Learn more about
Need a deep-dive on the concept behind this application? Look no further. Learn more about this topic, computer-science and related others by exploring similar questions and additional content below.Similar questions
- Continue your investigations into Pythagorean triples and/or Euler Bricks. Possible topics include: • Connections between Fibonacci numbers and Pythagorean triples. The number 5 is both a Pythagorean hypoteneuse and Fibonacci number. Do other numbers share these properties? • Pythagorean quadruples (x, y, z, w) where x² + y² + z² = w². ● Let x + y + z be the perimeter of the triple (x, y, z). In 1900, Derrick Lehmer proved that if N(p) is the number of primitive Pythagorean triples of perimeter ≤p, then N (p) log 2 P πT² as p→∞.arrow_forwardHow Kruskal's Algorithm Works?arrow_forwardCan someone explain to me the concept behind Karnaugh maps? Please use simplest detail possible since I'm a beginner.arrow_forward
arrow_back_ios
arrow_forward_ios
Recommended textbooks for you
- Database System ConceptsComputer ScienceISBN:9780078022159Author:Abraham Silberschatz Professor, Henry F. Korth, S. SudarshanPublisher:McGraw-Hill EducationStarting Out with Python (4th Edition)Computer ScienceISBN:9780134444321Author:Tony GaddisPublisher:PEARSONDigital Fundamentals (11th Edition)Computer ScienceISBN:9780132737968Author:Thomas L. FloydPublisher:PEARSON
- C How to Program (8th Edition)Computer ScienceISBN:9780133976892Author:Paul J. Deitel, Harvey DeitelPublisher:PEARSONDatabase Systems: Design, Implementation, & Manag...Computer ScienceISBN:9781337627900Author:Carlos Coronel, Steven MorrisPublisher:Cengage LearningProgrammable Logic ControllersComputer ScienceISBN:9780073373843Author:Frank D. PetruzellaPublisher:McGraw-Hill Education
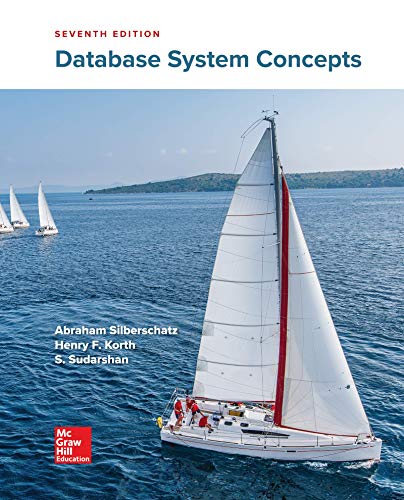
Database System Concepts
Computer Science
ISBN:9780078022159
Author:Abraham Silberschatz Professor, Henry F. Korth, S. Sudarshan
Publisher:McGraw-Hill Education
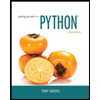
Starting Out with Python (4th Edition)
Computer Science
ISBN:9780134444321
Author:Tony Gaddis
Publisher:PEARSON
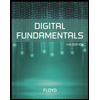
Digital Fundamentals (11th Edition)
Computer Science
ISBN:9780132737968
Author:Thomas L. Floyd
Publisher:PEARSON
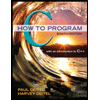
C How to Program (8th Edition)
Computer Science
ISBN:9780133976892
Author:Paul J. Deitel, Harvey Deitel
Publisher:PEARSON
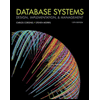
Database Systems: Design, Implementation, & Manag...
Computer Science
ISBN:9781337627900
Author:Carlos Coronel, Steven Morris
Publisher:Cengage Learning
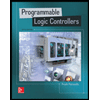
Programmable Logic Controllers
Computer Science
ISBN:9780073373843
Author:Frank D. Petruzella
Publisher:McGraw-Hill Education