Math 11 Lab #3
pdf
School
University of California, San Diego *
*We aren’t endorsed by this school
Course
11
Subject
Biology
Date
Dec 6, 2023
Type
Pages
5
Uploaded by DeanKnowledgeBoar4
Your preview ends here
Eager to read complete document? Join bartleby learn and gain access to the full version
- Access to all documents
- Unlimited textbook solutions
- 24/7 expert homework help
Recommended textbooks for you
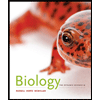
Biology: The Dynamic Science (MindTap Course List)
Biology
ISBN:9781305389892
Author:Peter J. Russell, Paul E. Hertz, Beverly McMillan
Publisher:Cengage Learning
Lifetime Physical Fitness & Wellness
Health & Nutrition
ISBN:9781337677509
Author:HOEGER
Publisher:Cengage
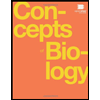
Concepts of Biology
Biology
ISBN:9781938168116
Author:Samantha Fowler, Rebecca Roush, James Wise
Publisher:OpenStax College
Recommended textbooks for you
- Biology: The Dynamic Science (MindTap Course List)BiologyISBN:9781305389892Author:Peter J. Russell, Paul E. Hertz, Beverly McMillanPublisher:Cengage Learning
- Lifetime Physical Fitness & WellnessHealth & NutritionISBN:9781337677509Author:HOEGERPublisher:CengageConcepts of BiologyBiologyISBN:9781938168116Author:Samantha Fowler, Rebecca Roush, James WisePublisher:OpenStax College
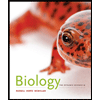
Biology: The Dynamic Science (MindTap Course List)
Biology
ISBN:9781305389892
Author:Peter J. Russell, Paul E. Hertz, Beverly McMillan
Publisher:Cengage Learning
Lifetime Physical Fitness & Wellness
Health & Nutrition
ISBN:9781337677509
Author:HOEGER
Publisher:Cengage
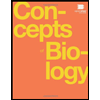
Concepts of Biology
Biology
ISBN:9781938168116
Author:Samantha Fowler, Rebecca Roush, James Wise
Publisher:OpenStax College