Module 3_Part2
.docx
keyboard_arrow_up
School
Georgia Institute Of Technology *
*We aren’t endorsed by this school
Course
6414
Subject
Statistics
Date
Apr 3, 2024
Type
docx
Pages
3
Uploaded by Mattyboo
It is important to remember that in this model, we do not have an error term!
Slide 9:
What are the model assumptions? A first assumption is the linearity of the link function of the probability of a success in the predicted variables, that is we write the g function of the probability of a success as a linear combination of the predicting variables. Although I'm going to refer to this assumption still as a linearity assumption, it is a different assumption than the linearity assumption in the regression model we have learned in the previous modules since the g link function is a non-linear transformation of the probability of the success or of the expectation of the response variable. Similar to the standard regression model, we also assume independence in the response data.
The third assumption is specific to the logistic regression model. The logistic regression model assumes that the link function is the so-called logit function, provided here on the
slide. The link function g is the log of the ratio of p over one minus p, where p again is the probability of success. This is an assumption since the logit function is not the only function that yields s-shaped curves.
There are other s-shaped functions that are used in modeling binary responses, under a more general model framework called binomial model. We'll learn about other shape functions in a different lesson.
Slide 10:
I will continue with illustrating logistic regression with a data example I will be using throughout the lessons introducing the Basic Concepts of Logistic Regression. In 1972-
1974 a survey was taken in Whickham, a mixed urban and rural district near Newcastle,
United Kingdom. Twenty years later a follow-up study was conducted. Among the information obtained originally was whether a person was a smoker or not. It was found that twenty years later, 76.12% of the 582 smokers were still alive with only 68.58% of 732 nonsmokers were still alive. That is, smokers had a higher survival rate than non-
smokers.
That will make the story for Philip Morris. Smoking leads
to a longer life span.
This example was provided by Dr. Jeffrey Simonoff from New York University.
Slide 11:
This slide includes the R code to get you started with reading the data. Here is also the code for plotting the age versus the proportion of those that survived. We want to compare the relationship between age and the proportion of survival by smokers and nonsmokers separately.
The plot shows a non-linear relationship between age and survival proportion. In fact, this looks more like an S shape
,
as
I motivated in the previous lesson where I introduced
the logistic regression model.
Slide 13:
Next, I transformed the survival proportion using the logit function, which is the log of the ratio between the proportion of survival divided by 1 minus the proportion of survival, which is called logit transformation or link funciton. Here I'm plotting the age versus the logit of the proportion of survival.
I'm contrasting the plot that you saw in a previous slide on the left to the plot of the age
versus logit of the survival rate. The relationship between age and the transformed survival rate improved compared to the un-transformed survival proportion. We still see
a slight curvature. I will expand more on this when we're going to perform the logistic regression analysis on this example.
Your preview ends here
Eager to read complete document? Join bartleby learn and gain access to the full version
- Access to all documents
- Unlimited textbook solutions
- 24/7 expert homework help
Related Questions
You did not really answer my question. You only included dummy variables for MSS. You need to also include dummy variables for BOROUGH and the make the model variables for both MSS and BOROUGH.
You were asked to help with analysis of birth weights (BW) of 10,000 infants born in NYC during a certain period of time.
The aim of the analysis is to see whether the birth weights of the infants are associated with mothers AGE at birth (continuous variable in years) and mothers smoking status (the maternal smoking status MSS contains 4 categories “Non-smoker”, “Past-smoker”, “Passive-smoker”, “Smoker”) and NYC boroughs (the BOROUGH variable contains 5 categories “Manhattan”, “Bronx”, “Brooklyn”, “Queens” and “Staten Island”). Questions:
1.Write down the population model that estimates BW based on variables AGE and BOROUGH(with the dummy variables). Make sure that it is clear what each predictor means.
2.How many parallel lines are computed by model from population model you created above?…
arrow_forward
A company provides maintenance service for water-filtration systems throughout southern Florida. Customers contact the company with requests for maintenance service on their water-filtration systems. To estimate the service time and the service cost, the company's managers want to predict the repair time necessary for each maintenance request. Hence, repair time in hours is the dependent variable. Repair time is believed to be related to three factors, the number of months since the last maintenance service, the type of repair problem (mechanical or electrical), and the repairperson who performed the service. Data for a sample of 10 service calls are reported in the table below.
Repair Timein Hours
Months SinceLast Service
Type of Repair
Repairperson
2.9
2
Electrical
Dave Newton
3.0
6
Mechanical
Dave Newton
4.8
8
Electrical
Bob Jones
1.8
3
Mechanical
Dave Newton
2.5
2
Electrical
Dave Newton
4.9
7
Electrical
Bob Jones
4.6
9
Mechanical
Bob Jones
4.8
8
Mechanical
Bob…
arrow_forward
A company provides maintenance service for water-filtration systems throughout southern Florida. Customers contact the company with requests for maintenance service on their water-filtration systems. To estimate the service time and the service cost, the company's
managers want to predict the repair time necessary for each maintenance request. Hence, repair time in hours is the dependent variable. Repair time is believed to be related to three factors, the number of months since the last maintenance service, the type of repair
problem (mechanical or electrical), and the repairperson who performed the service. Data for a sample of 10 service calls are reported in the table below.
Repair Time Months Since
in Hours
Last Service
2.9
3.0
4.8
1.8
2.5
4.9
4.2
4.8
4.4
4.5
2
6
8
3
2
7
9
8
4
6
Type of Repair Repairperson
Electrical
Mechanical
Electrical
Mechanical
Electrical
Electrical
Mechanical
Mechanical
Electrical
Electrical
Dave Newton
ŷ =
X
Check which variable(s)/term(s) should be in your…
arrow_forward
he management of Lindiwe Accounting Services are concerned about the increase in overtime hours clocked by their senior staff over the past six months of 2022. As the company’s cost accountant, they have assigned you the task of coming up with a reliable way to predict overtime hours and costs for senior staff in the next six months of 2022. You have decided to use linear regression to solve the problem. You have also recorded the following monthly figures for the past six months of 2022. Month Overtime hours Total cost (R) January 478 41 000 February 322 32 000 March 405 37 000 April 308 28 200 May 505 43 500 June 308 29 600 Required: Construct a linear regression equation that your company management can use to predict overtime hours in the future (round all answers to two decimals)
arrow_forward
SEE MORE QUESTIONS
Recommended textbooks for you
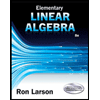
Elementary Linear Algebra (MindTap Course List)
Algebra
ISBN:9781305658004
Author:Ron Larson
Publisher:Cengage Learning
Algebra & Trigonometry with Analytic Geometry
Algebra
ISBN:9781133382119
Author:Swokowski
Publisher:Cengage
Related Questions
- You did not really answer my question. You only included dummy variables for MSS. You need to also include dummy variables for BOROUGH and the make the model variables for both MSS and BOROUGH. You were asked to help with analysis of birth weights (BW) of 10,000 infants born in NYC during a certain period of time. The aim of the analysis is to see whether the birth weights of the infants are associated with mothers AGE at birth (continuous variable in years) and mothers smoking status (the maternal smoking status MSS contains 4 categories “Non-smoker”, “Past-smoker”, “Passive-smoker”, “Smoker”) and NYC boroughs (the BOROUGH variable contains 5 categories “Manhattan”, “Bronx”, “Brooklyn”, “Queens” and “Staten Island”). Questions: 1.Write down the population model that estimates BW based on variables AGE and BOROUGH(with the dummy variables). Make sure that it is clear what each predictor means. 2.How many parallel lines are computed by model from population model you created above?…arrow_forwardA company provides maintenance service for water-filtration systems throughout southern Florida. Customers contact the company with requests for maintenance service on their water-filtration systems. To estimate the service time and the service cost, the company's managers want to predict the repair time necessary for each maintenance request. Hence, repair time in hours is the dependent variable. Repair time is believed to be related to three factors, the number of months since the last maintenance service, the type of repair problem (mechanical or electrical), and the repairperson who performed the service. Data for a sample of 10 service calls are reported in the table below. Repair Timein Hours Months SinceLast Service Type of Repair Repairperson 2.9 2 Electrical Dave Newton 3.0 6 Mechanical Dave Newton 4.8 8 Electrical Bob Jones 1.8 3 Mechanical Dave Newton 2.5 2 Electrical Dave Newton 4.9 7 Electrical Bob Jones 4.6 9 Mechanical Bob Jones 4.8 8 Mechanical Bob…arrow_forwardA company provides maintenance service for water-filtration systems throughout southern Florida. Customers contact the company with requests for maintenance service on their water-filtration systems. To estimate the service time and the service cost, the company's managers want to predict the repair time necessary for each maintenance request. Hence, repair time in hours is the dependent variable. Repair time is believed to be related to three factors, the number of months since the last maintenance service, the type of repair problem (mechanical or electrical), and the repairperson who performed the service. Data for a sample of 10 service calls are reported in the table below. Repair Time Months Since in Hours Last Service 2.9 3.0 4.8 1.8 2.5 4.9 4.2 4.8 4.4 4.5 2 6 8 3 2 7 9 8 4 6 Type of Repair Repairperson Electrical Mechanical Electrical Mechanical Electrical Electrical Mechanical Mechanical Electrical Electrical Dave Newton ŷ = X Check which variable(s)/term(s) should be in your…arrow_forward
arrow_back_ios
arrow_forward_ios
Recommended textbooks for you
- Elementary Linear Algebra (MindTap Course List)AlgebraISBN:9781305658004Author:Ron LarsonPublisher:Cengage LearningAlgebra & Trigonometry with Analytic GeometryAlgebraISBN:9781133382119Author:SwokowskiPublisher:Cengage
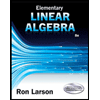
Elementary Linear Algebra (MindTap Course List)
Algebra
ISBN:9781305658004
Author:Ron Larson
Publisher:Cengage Learning
Algebra & Trigonometry with Analytic Geometry
Algebra
ISBN:9781133382119
Author:Swokowski
Publisher:Cengage