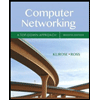
Computer Networking: A Top-Down Approach (7th Edition)
7th Edition
ISBN: 9780133594140
Author: James Kurose, Keith Ross
Publisher: PEARSON
expand_more
expand_more
format_list_bulleted
Question
Using Python,
Create a k-fold cross validation code with the number of folds being 10.
- The raw data needs to be split into training and testing.
- The number of folds is the number of times you should go through a for loop.
- Inside of the for loop, index into your raw data to pull out training and testing data based on the fold (think about how the folds are organized and how much data is in each fold, which is based on the total number of folds)
- Do normal linear regression model fitting
- Keep track of the R^2 scores of the folds
- Print the average and standard deviation of the R^2 value for the different folds
![Our libraries that we are importing:
# 1a. Importing all the libraries necessary for this assignment
import seaborn as sns
import pandas as pd
import numpy as np
import matplotlib.pyplot as plt
from sklearn.linear_model import LinearRegression
[7] # 1b. Loading the data
data = sns.load_dataset('geyser')
Let's check out this dataset.
[9] print('
data
1
duration waiting kind
3.600
79
long
1
1.800
54 short
2
3.333
74
long
3
2.283
62 short
4
4.533
85
long
267
4.117
81
long
268
2.150
46 short
269
4.417
90
long
270
1.817
46 short
271
4.467
74
long
272 rows x 3 columns
Imagine working at Yellowstone National Park as a scientist researching Old Faithful Geyser. The boss wants us to evaluate the predictiveness
of the relationship between duration of an eruption in minutes ("duration") and the duration in minutes until the next eruption ("waiting"). We
collected the data, but the internet in Yellowstone is not very good, so we cannot access the crossvalidation library in python](https://content.bartleby.com/qna-images/question/7c9fe6be-99fb-4863-a04f-7bda979173e6/8224c764-6eda-4fff-b719-d20b5a73993e/bejud5m_thumbnail.png)
Transcribed Image Text:Our libraries that we are importing:
# 1a. Importing all the libraries necessary for this assignment
import seaborn as sns
import pandas as pd
import numpy as np
import matplotlib.pyplot as plt
from sklearn.linear_model import LinearRegression
[7] # 1b. Loading the data
data = sns.load_dataset('geyser')
Let's check out this dataset.
[9] print('
data
1
duration waiting kind
3.600
79
long
1
1.800
54 short
2
3.333
74
long
3
2.283
62 short
4
4.533
85
long
267
4.117
81
long
268
2.150
46 short
269
4.417
90
long
270
1.817
46 short
271
4.467
74
long
272 rows x 3 columns
Imagine working at Yellowstone National Park as a scientist researching Old Faithful Geyser. The boss wants us to evaluate the predictiveness
of the relationship between duration of an eruption in minutes ("duration") and the duration in minutes until the next eruption ("waiting"). We
collected the data, but the internet in Yellowstone is not very good, so we cannot access the crossvalidation library in python
Expert Solution

This question has been solved!
Explore an expertly crafted, step-by-step solution for a thorough understanding of key concepts.
Step by stepSolved in 2 steps with 1 images

Knowledge Booster
Similar questions
- Please help (on jupyter note in python) Homecoming Shopping ListWe bought the following souvenirs for homecoming gathering: 5 shirts ( $ 10/item), $ 10 Polo Shirts ( $ 20.75/item), 5 trousers ( $ 29.95/item), 10 caps ( $ 11.95/item), 20 pens ( $ 1.99/item), 20 hoodies ( $ 24.95/item), and 30 chocoloates ( $ 2.95/item). Use Dot Product and NumPy arrays to compute the total cost of Homecoming.arrow_forwardWhen an author produces an index for his or her book, the first step in this process is to decide which words should go into the index; the second is to produce a list of the pages where each word occurs. Instead of trying to choose words out of our heads, we decided to let the computer produce a list of all the unique words used in the manuscript and their frequency of occurrence. We could then go over the list and choose which words to put into the index. The main object in this problem is a "word" with associated frequency. The tentative definition of "word" here is a string of alphanumeric characters between markers where markers are white space and all punctuation marks; anything non-alphanumeric stops the reading. If we skip all un-allowed characters before getting the string, we should have exactly what we want. Ignoring words of fewer than three letters will remove from consideration such as "a", "is", "to", "do", and "by" that do not belong in an index. In this project, you…arrow_forwardYou will be using on RV related review comments to train a Sentence completion model. You could use the code below. The code is written in Jupyter notebook python. please follow the following instructions: 1. Build a working sentence completion model. Training data is provided in train.csv. This model should take partial sentences (e.g., "hey how ar," "hello, please") and provide completion for that sentence. Not every input needs to be completed into a correct sentence (e.g., "asdfauaef" does not need to have a sentence completion). For this model's purposes, a sentence is considered completed if an "end of sentence" character (like a full stop, question mark, etc.) occurs within the next five words OR. We predict up to 5 words from the input. 2. BONUS: If you use word vectors or other techniques from what we shared in class using character encoding and have a working model. 3. The test data (test.csv) will be used to evaluate the model. The test file contains partial sentences. Use…arrow_forward
- Assume you need to store book data in a structured manner. Either more books should be added, or books that are already there should only be removed from one side of the shelf. Choose the best data structure for this case and describe it. Give a dynamic example of how books are added to a shelf. Additionally, what happens when there isn't enough room in a data structure for new data to be added?arrow_forwardUsing the given data use the R software to create a side-by-side boxplot for the weights of the offense vs the weights of the defense.arrow_forward"In this exercise we will use code that we already have. Use the fucntion you created to compute the precision of a model, remember that the precision of a model is:$precision=(tp/(tp+fp))$ where tp is the number of true positives and fp is the number of false positives. The file performance.txt has data from models that were used in an experiment, the data has the model id, number of true positives and number of false positives for each model. Remember again that the model is good if its precision is above 0.75.\n", "\n", "Open the file, read each model and print if the model is good or bad. At the end, print the id of the best model and its precision. Be sure to use a function to compute the precision of each model."arrow_forward
- Create a data type that allows you to insert, remove the maximum, and delete the minimum (all in logarithmic time), as well as locate the maximum and find the minimum (both in constant time). Use two piles as a hint.arrow_forwardThe second image shows the final result but I just want to know how we get there, thanks!arrow_forwardThe Marketing team of InstantRide wants to know that how many discounts have been offered for each ride. You need to calculate this information for each travel where a discount is applied and return two columns: TRAVEL_ID and DISCOUNT_AMOUNT. In addition, you need to return the calculation as a money value using the ROUND function to 2 decimals.arrow_forward
- Complete the docstring using the information attached (images):def upgrade_stations(threshold: int, num_bikes: int, stations: List["Station"]) -> int: """Modify each station in stations that has a capacity that is less than threshold by adding num_bikes to the capacity and bikes available counts. Modify each station at most once. Return the total number of bikes that were added to the bike share network. Precondition: num_bikes >= 0 >>> handout_copy = [HANDOUT_STATIONS[0][:], HANDOUT_STATIONS[1][:]] >>> upgrade_stations(25, 5, handout_copy) 5 >>> handout_copy[0] == HANDOUT_STATIONS[0] True >>> handout_copy[1] == [7001, 'Lower Jarvis St SMART / The Esplanade', \ 43.647992, -79.370907, 20, 10, 10] True """arrow_forwardSplit blood pressure into systolic and diastolic blood pressure with integer as data type readings and remove the original column. Examine the data and look for predictor columns that are indicator variables, 0 or 1. Convert the data type to Boolean. Using code prepare lists of predictor column names for each data type. Make sure all the predictor columns are included in one and only one list. Print the length of the list of each data type and the sum of the lengths and verify that all predictor variables are accounted for in the lists. Use f-string to align the numbers properly.arrow_forwardUse an appropriate scikit-learn library we used in class to create y_train, y_test, X_train and X_test by splitting the data into 70% train and 30% test datasets. Set random_state to 4 and stratify the subsamples so that train and test datasets have roughly equal proportions of the target's class labels Standardise the data using StandardScaler libraryarrow_forward
arrow_back_ios
SEE MORE QUESTIONS
arrow_forward_ios
Recommended textbooks for you
- Computer Networking: A Top-Down Approach (7th Edi...Computer EngineeringISBN:9780133594140Author:James Kurose, Keith RossPublisher:PEARSONComputer Organization and Design MIPS Edition, Fi...Computer EngineeringISBN:9780124077263Author:David A. Patterson, John L. HennessyPublisher:Elsevier ScienceNetwork+ Guide to Networks (MindTap Course List)Computer EngineeringISBN:9781337569330Author:Jill West, Tamara Dean, Jean AndrewsPublisher:Cengage Learning
- Concepts of Database ManagementComputer EngineeringISBN:9781337093422Author:Joy L. Starks, Philip J. Pratt, Mary Z. LastPublisher:Cengage LearningPrelude to ProgrammingComputer EngineeringISBN:9780133750423Author:VENIT, StewartPublisher:Pearson EducationSc Business Data Communications and Networking, T...Computer EngineeringISBN:9781119368830Author:FITZGERALDPublisher:WILEY
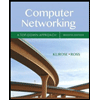
Computer Networking: A Top-Down Approach (7th Edi...
Computer Engineering
ISBN:9780133594140
Author:James Kurose, Keith Ross
Publisher:PEARSON
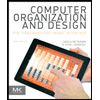
Computer Organization and Design MIPS Edition, Fi...
Computer Engineering
ISBN:9780124077263
Author:David A. Patterson, John L. Hennessy
Publisher:Elsevier Science
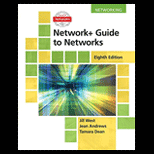
Network+ Guide to Networks (MindTap Course List)
Computer Engineering
ISBN:9781337569330
Author:Jill West, Tamara Dean, Jean Andrews
Publisher:Cengage Learning
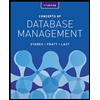
Concepts of Database Management
Computer Engineering
ISBN:9781337093422
Author:Joy L. Starks, Philip J. Pratt, Mary Z. Last
Publisher:Cengage Learning
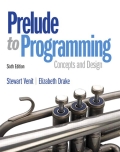
Prelude to Programming
Computer Engineering
ISBN:9780133750423
Author:VENIT, Stewart
Publisher:Pearson Education
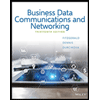
Sc Business Data Communications and Networking, T...
Computer Engineering
ISBN:9781119368830
Author:FITZGERALD
Publisher:WILEY