Final_solution_2023
pdf
School
University of Florida *
*We aren’t endorsed by this school
Course
401
Subject
Business
Date
Jan 9, 2024
Type
Pages
15
Uploaded by ConstableElectronGrasshopper43
Business 36106 (Autumn 2023)
Final (Solutions)
36106: Managerial Decision Modeling
Autumn 2023
FINAL (Solutions)
December 2023
May the force be with
Harry Q. Bovik
I pledge my honor that I have not violated the Chicago Booth Honor Code during this examination. I
have not shared, transmitted or received written or electronic answers with/to/from other classmates.
(Sign):
H.Q. Bovik
Q1 (7 pts)
Q2 (5 pts)
Q3 (7 pts)
Q4 (11 pts)
TOTAL (30)
Instructions
1. This is a
timed exam with 72 hour duration
. You will have 72 hours from the time you accessed
this exam to upload your submission. However, you should submit your exam
no later than 11:59
pm on Thursday, December 7, 2023
.
2. There are 4 questions and
13
numbered pages. Maximum possible score is 39.
3. The information contained in this documents is confidential, privileged and only for the information
of the intended recipient and may not be used, published or redistributed without the prior written
consent of the instructor.
4.
This exam is to be done individually.
You are not to receive help from or give help to others.
There should be no discussion of the exam or the topics covered, nor any discussion of course-
related software, nor any sharing of course or exam-related files, between the time you download
the exam and the time you hand in your completed work. You may not discuss this exam with
anybody who has not already completed it.
5. You may not save any exam-related files on shared disk space and may not access others’ exam-
related files.
6. This exam is open book and open notes.
This means that you may use your course cases,
handouts, notes, and assignments received and/or produced while taking this course. You may
i
Business 36106 (Autumn 2023)
Final (Solutions)
access course files (slides, examples, etc.) posted on Canvas during the exam. You may not use
notes or materials from this course (or similar courses) in other years or in other programs. You
may not use any materials from the internet other than those posted on Canvas.
7. Do not post information or ask questions related to the exam or course to any electronic bulletin
board, forum, or discussion group (or on Facebook, twitter, etc.) during the period of the exam.
8.
Answer sheets:
Scan and upload your answer sheets to Canvas by the deadline. You can also
use any pdf editing software to type your solutions. You can insert extra pages if you need more
space to write your answers, or to include screenshots of your results. Upload a single pdf file,
and make sure that your pdf is readable by Adobe Acrobat.
If you are running out of time in
scanning your answersheet, upload photos of the answersheet, and email us your pdf answersheet
within 24 hours.
9.
Excel files:
Download the excel files from Canvas or create a new file as indicated in the questions,
implement your Solver/PrecisionTree/RISK models in them, and upload these files with your
answersheet to Canvas by the deadline.
10. Write your answers in the space indicated.
Where asked for constraints or objective function,
write a very short English description followed by a precise mathematical expression. Example:
Capacity constraint for Plant 1 :
X
11
+
X
12
+
X
13
≤
4000
Rambling, unfocused qualitative answers will be penalized.
11. For your @RISK simulations, unless specified, choose number of iterations as 1000. It is encouraged
that you include screenshots of your simulation outputs (e.g., histograms, RiskSimTable outcomes)
in either your uploaded Excel workbooks, or in your answersheet.
ii
Business 36106 (Autumn 2023)
Final (Solutions)
1
LLC’s Strategic Alliance Problem [7 pts]
Create a file
LLC
Strategic
alliance.xlsx
. Build any PrecisionTree models in this workbook
and upload with the rest of your submission.
As she was driving home Friday evening, Yiduo Chen, Vice President of Sales & Marketing at Lexing-
ton Laser Corporation (LLC), kept thinking about the interesting opportunity she had stumbled upon
during last week’s west-coast business development trip. It seems that Aspen Networks, an emerging
company in the network business, has a requirement for a custom laser transmitter for one of their new
transmission systems.
Because Aspen’s strength is in communications systems and not in opto-electronic components, they
indicated that they are interested in forging a strategic supply arrangement with LLC (or perhaps some
other opto-electronic component manufacturer) for the supply of custom laser transmitters. Aspen is
confident that they can carve out a leadership position in the market with their new system which could
translate into
10 million in laser transmitter orders for LLC over three years.
Yiduo was definitely
excited about this potential opportunity for LLC.
After reviewing the technical specifications for the custom part with Peter Williams (VP of Engineering),
it appeared that with minimal engineering investment LLC could adapt one of their existing designs to
obtain the requisite functionality. Yiduo then started working with the manufacturing folks: Julie Weller
(VP of Manufacturing) and Steve Lo (Manufacturing Engineering Manager). Julie and Steve felt that
although there was sufficient excess capacity at LLC to produce the required laser chips, the rest of the
manufacturing process for the custom lasers would require establishing a small dedicated manufacturing
line at a cost of
800,000. It would take four months to build this facility – just three months before
LLC would expect the first orders from Aspen.
Yiduo then tried to look more carefully at the numbers. The Aspen management team was optimistic
that they could capture enough business to provide LLC with orders for 10,000 lasers over three years.
Indeed, at a price of
1,000 per laser (giving the
10 million revenue mentioned earlier) and 20% after-
tax profit margins, this opportunity seemed profitable. However, as Yiduo studied the network market
a bit closer, she learned that while Aspen had some leading edge technologies and a novel approach,
Aspen also had two established competitors, and the optimistic scenario above may not materialize.
Yiduo also thought that given the rapid pace of technological innovation and commercialization in the
communications industry, this custom laser would most probably have a relatively brief lifecycle of three
years, and that much of the dedicated manufacturing facility would not have an alternate use beyond
the third year. With input from Aspen and their customers, Yiduo estimated the best-case and worst-
case revenues (corresponding to the range of Aspen’s acceptance in the market) and post-tax profit
projections as follows:
Best-case:
Year 1 revenue
2 million, Year 2 revenue
5 million, Year 3 revenue
3 million. This gave
a total revenue of
10 million over three years yielding after-tax profits (excluding the cost of setting
up the dedicated manufacturing line) of
2 million.
Worst-case:
Year 1 revenue
0.5 million, Year 2 revenue
1.25 million, Year 3 revenue
0.75 million.
This gave a total revenue of
2.5 million over three years yielding after-tax profits (excluding the cost
of dedicated manufacturing line) of
0.5 million.
1
Your preview ends here
Eager to read complete document? Join bartleby learn and gain access to the full version
- Access to all documents
- Unlimited textbook solutions
- 24/7 expert homework help
Business 36106 (Autumn 2023)
Final (Solutions)
The probability of “Best-case” is subjectively estimated to be 70%.
At a meeting with the Aspen team, Yiduo explained her company’s reluctance to invest in a custom
manufacturing facility with an uncertain future.
Hank Philips (purchasing manager of Aspen) then
explained that Aspen has also been talking with another laser manufacturer to explore contract manu-
facturing of custom lasers. Although LLC was Aspen’s first choice, the other manufacturer had already
committed the resources for a dedicated line, and Aspen had even placed preliminary orders.
Yiduo
then brought up the possibility for LLC to wait six months prior to committing to the dedicated line, in
order to see how Aspen’s new system fares in the marketplace. Hank explained that while they would
be willing to work with LLC for the following two years, the laser orders they would place with LLC for
years two and three would then be smaller by approximately 30%. That is,
Best-case under strategy of waiting six months before committing:
Year 1 revenue
0, Year 2
revenue
3.5 million, Year 3 revenue
2.1 million.
Worst-case under strategy of waiting six months before committing:
Year 1 revenue
0, Year 2
revenue
0.875 million, Year 3 revenue
0.525 million.
While the revenue opportunity was lower in the more conservative approach of waiting six months prior
to building the line, it would allow LLC to know exactly whether Aspen would be successful (“best-case”)
or not (“worst-case”) in the marketplace. In the event Aspen was not successful, LLC would be spared
the investment in a manufacturing facility that would not pay for itself.
As Yiduo drove home, she mulled over the options. Build the facility or not? Hold off for six months
to see whether Aspen is successful?
(a)
[1 pts]
What is the expected payoff (including the cost to setup the facility) if LLC decides to go
ahead with the alliance with Aspen now? Assume a discount rate of 0% throughout this ques-
tion. You need not create a PrecisionTree model for this question, but you should explain your
calculations.
Answer:
There is an immediate cost of
800,000 to set up the facility; and subsequently a profit of
2
million with 70% chance and
0.5 million with 30% chance. Overall expected payoff
=
−
0
.
8 + (0
.
7
×
2 + 0
.
3
×
0
.
5) = 0
.
75
million
.
(b)
[1 pts]
What is the expected payoff if LLC decides to wait 6 months?
You need not create a
PrecisionTree model for this question, but you should explain your calculations.
Answer:
If Aspen will be successful, the profit for LLC would be
0
.
2
×
(3
.
5 + 2
.
1) = 1
.
12
million, therefore
in this Aspen would invest the fixed cost in setting up the facility. If Aspen will be unsuccessful,
LLC will not form the alliance and payoff would be 0. Overall expected payoff
= 0
.
7
×
(
−
0
.
8 + 1
.
12) + 0
.
3
×
0 = 0
.
224
million
.
2
Business 36106 (Autumn 2023)
Final (Solutions)
(c)
[1 pts]
The chance of Aspen’s success
p
success
= 70%
was a subjective estimate. LLC would like
to know at what value for the chance of success of Aspen would the expected payoffs under the
“proceed now” and “wait 6 months” options be equal. You need not create a PrecisionTree model
for this question, but you should explain your calculations.
Answer:
The payoff if LLC proceeds now is
=
−
0
.
8 + 2
p
+ 0
.
5(1
−
p
)
and if LLC waits is
=
p
(
−
0
.
8 + 1
.
12) = 0
.
32
p.
Equating the two, we get
p
=
0
.
8
−
0
.
5
1
.
5
−
0
.
32
=
0
.
3
1
.
18
= 0
.
254
.
That is, if Aspen’s probability of success is higher than 25.4% then LLC should go ahead with the
alliance now instead of waiting (assuming LLC is risk neutral and expected payoff maximizer).
(d)
[1 pts]
Suppose LLC could access perfect forecast about Aspen’s success/failure in the mar-
ketplace, how much should LLC be willing to pay for this forecast?
You need not create a
PrecisionTree model for this question, but you should explain your calculations carefully.
Answer:
With perfect forecast, LLC would have a payoff of
−
0
.
8 + 2 = 1
.
2
million in the case Aspen is
successful and a payoff of 0 if Apsen is not successful. The expected payoff would be
0
.
7
×
1
.
2 =
0
.
84
million.
Without this perfect forecast, the optimal decision for LLC is to form an alliance now, with an
expected payoff of
0
.
75
million.
Therefore the value of this perfect forecast is
0
.
84
−
0
.
75 = 0
.
09
million, or
$90
,
000
.
(e)
[3 pts]
Yiduo called her friend Frank Sullivan, who runs a market research firm focusing in fiber
optic communications and networking. Frank was well-versed in the dynamics of Aspen’s market,
and he seemed to have a good sense of their target customers.
He felt that by putting three
analysts on the problem for two months, his company could give Yiduo an excellent assessment of
Aspen’s prospect in this market. The study would cost LLC
150,000. Of course, Yiduo was not
as excited as Frank was about commissioning a market research study. Yiduo’s previous experience
indicated that such predictions are wrong 30% of the time. That is, if the true outcome would
have been ‘success’, the prediction would be ‘success’ only 70% of the time, and if true outcome
would been ‘not successful’ the prediction would be ‘not successful’ only 70% of the time.
Should Yiduo commission the study? Build a Precision Tree model to solve for Yiduo’s optimal
strategy given she commissions the study, and use the solution to answer this question.
Hint:
You first need to compute the probabilities that the study will report the outcomes as
‘success’ or ‘not successful’ based on the information above.
From these, you then need to
compute the probability that the true outcome is ‘success’ given that the report says ‘success’
using elementary probability.
3
Business 36106 (Autumn 2023)
Final (Solutions)
Answer:
The optimal tree is given below. The optimal decision given either market report is to continue
with alliance now, which is also the decision without the market report.
Therefore there is no
value for this imperfect forecast.
4
Your preview ends here
Eager to read complete document? Join bartleby learn and gain access to the full version
- Access to all documents
- Unlimited textbook solutions
- 24/7 expert homework help
Business 36106 (Autumn 2023)
Final (Solutions)
2
LLC’s Yield Problem [5 pts]
Download the workbook
LLC
yield
data.xlsx
. Implement your @RISK model in this file and
upload to Canvas with the rest of your Final submission.
“What? Again? Julie, this is the third time this year we’re missing a shipment to Aspen. What’s going
on?” Yiduo Chen could not believe it. At the weekly meeting of the Manufacturing and Sales groups,
Julie Weller (VP of Manufacturing) explained that they will have to miss another shipment to Aspen
Networks. As the primary interface between LLC and Aspen, Yiduo was not happy about delivering this
bad news again.
Julie explained: “Yiduo, we’ve gone over this before. Your forecast keeps changing, and our cycle time
which, by the way, we’ve successfully reduced to three weeks is still too long to react to last-minute sales
orders. After all, our manufacturing process yields vary. The best we can do is estimate how many lasers
we need to start each week, based on how many we need to yield. When you throw demand variability
into the mix, it’s not surprising we miss an occasional shipment. Look at the numbers: Aspen needs
500 lasers per week on average; I’m starting 800 every week, and yielding nearly 600 and we usually
meet their demand.”
Julie had a good point.
The manufacturing operation had done a lot to reduce cycle time and was
operating efficiently. The real culprits were the fluctuations in customer demand and in process yields.
They both realized, however, that rapidly filling orders was critical to LLC’s long-term success.
Yiduo thought out loud:
“Julie, we know Aspen needs roughly 500 lasers per week and sometimes
10-20% more. Why don’t we just start enough lasers to yield enough for them, with an extra margin
of safety?”
Julie reluctantly acknowledged that this might be their only option for the near-term.
However, she did point out:
“If we don’t pick the right quantities, our inventories will go through
the roof. Furthermore, creating excess capacity is expensive, in terms of both capital equipment and
staffing. This is clearly a trade-off between carrying excess inventory and customer satisfaction.”
“Aha, now we’re getting somewhere. Julie, let’s take the guess-work out of this process. Why don’t we
simulate the production process and the customer demand, and quantify this trade-off between customer
satisfaction and production starts? Why don’t we take a week to collect the data on our manufacturing
processes and demand fluctuations, and reconvene to make the decision.
The Data
The manufacturing process for the Aspen lasers consists of three steps: submount assembly, module
assembly, and final test. Parts that successfully pass through one step go on to the next step. Those
parts that fail are scrapped. The weekly demand from Aspen for lasers has been 500 lasers on average,
but it fluctuates. It is approximately Normally distributed, with a standard deviation of 50 lasers per
week. The data collected on yields of the manufacturing process steps is as follows:
The submount assembly yield is approximately Normally distributed, with mean = 80% and
standard deviation = 5%. (That is, if
n
lasers start the submount assembly step, the number that
pass is
Y
×
n
where
Y
is approximately Normal with mean
0
.
8
and standard deviation
0
.
05
).
The module assembly yield is approximately Binomially distributed, with the Prob
(
pass
) = 95%
.
The final test yield appears to be Uniformly distributed between 90% and 100%.
5
Business 36106 (Autumn 2023)
Final (Solutions)
(a)
[3.5 pts]
Your first task is to build a simulation model to estimate the distribution of the weekly
output of the manufacturing process and the distribution of the supply-demand surplus/deficit
assuming the current policy of 800 starts per week. Briefly (2-3 sentences) describe the logic of
your model and any assumptions you make. Build a RISK simulation model in the excel workbook
for this question. Answer the questions below based on one simulation run with 1000 iterations.
Answer:
Model Logic:
Given the number of starts, let us call this
S
0
, we first sample the first step yield
from Normal(0.8,0.05) truncated below at 0 and above at 1, and then multiply this by
S
0
and
round it to an integer to get the number of units that pass step 1 as
S
1
. We need
S
1
to be a
non-negative integer so that we can use the Binomial distribution in step 2. The number of units
that pass the second stage is samples as
S
2
=Binomial(
S
1
, 0.95).
Finally, the number of units
that pass the third stage is obtained by first sampling the yield from Uniform(0.9,1), multiplying
this by
S
2
and rounding this product to get
S
3
.
What is the mean number successful lasers produced per week (report 90% confidence interval)?
577.6
±
2.1
What are the 10th and 90th percentiles of the number of successful lasers produced per week?
511, 643
What is the mean of the weekly surplus/deficit in supply/demand (90% confidence interval)?
77.55
±
3.3
How often (fraction of weeks) will LLC miss a shipment according to your model?
12.4%
(b)
[1.5 pts]
How many starts per week are required in order to guarantee that demand will be met
95% of the time? 99% of the time? 99.5% of the time? You can report your answer within
±
20 (i.e., build a RiskSimTable where you list candidate values for number of starts in increments
of 20 and report the closest candidate).
Since in this question we are looking for likelihood of
rare events (0.5% chance of missed shipments) set the number of iterations to 5000 to get a
reasonable sample size.
Answer:
For 95%, I got number of starts between 840 and 860. For 99%, I got between 900 and 920. For
99.5% I got between 920 and 940.
6
Business 36106 (Autumn 2023)
Final (Solutions)
3
LLC’s Inventory Management Problem [7 pts]
Download the workbook
LLC
inventory
data.xlsx
. Build your @RISK model in this file and
upload to Canvas with the rest of your Final submission.
Yiduo thought more about the solution of increasing the number of starts each week and realized that
this would indeed lead to the inventory shooting up since most weeks LLC would end up manufacturing
more laser units than required by Aspen. In consultation with Julie Weller, she decided that a better
solution might be to
(i)
cap the production once the inventory, after serving that week’s demand, ex-
ceeded a set threshold
T
(this is a decision variable to be optimized), and
(ii)
use overtime labor in a
week if the yield from the regular number of starts does not fulfill the week’s demand.
For this question we will assume the following simpler model of the yield of the manufacturing process
in each week:
The combined yield (after all the three steps) is approximately Normally distributed, with mean = 80%
and standard deviation = 5%. (That is, if
n
lasers start the submount assembly step, the number that
pass is
Y
×
n
where
Y
is approximately Normal with mean
0
.
8
and standard deviation
0
.
05
). The yield
Y
in each week is independent of yields of other weeks, and obeys the Normal distribution.
Here is how Julie and Yiduo have decided to operate the manufacturing process of lasers (see the ex-
amples below for clarity): They would begin by installing a regular production capacity of
C
starts per
week (this is also a decision variable they would like to optimize). Each week
n
would begin with some
finished inventory carried over from the previous week (
I
n
−
1
), and some demand from Aspen for this
week (
D
n
). We will assume the demand for each week is approximately Normally distributed with mean
of 500 and standard deviation of 50. The manufacturing unit uses the regular production capacity of
C
starts so that no more than the threshold
T
units of inventory are carried to the next week after
meeting the demand
D
n
.
However, if it turns out that even after using the full production capacity
of
C
the demand for the current month is not met, then overtime must be used. Since highly skilled
labor is required for the process, overtime costs 4 times as much (per start) than regular capacity and
therefore overtime is only used to the extent that the demand for the week is met. Overtime is never
used to produce extra units to carry in inventory for the next week.
The cost parameters are as follows: Installation of regular capacity costs
$100
per unit per week. This
cost is incurred even if the entire regular production capacity is not utilized. Overtime costs
$400
per
unit started, which is only utilized if overtime production is used. The inventory carrying cost is
$30
per
unit per week.
Here are a couple of examples to explain the above. Imagine that LLC installs a regular capacity of
C
= 600 starts per week, and uses a threshold
T
= 50 units for the maximum inventory carried at the
end of any week.
Example 1
: The week begins with
I
n
−
1
=30 units carried from the previous week, and a demand of
D
n
= 460
units. Let the random yield for this month be
Y
n
= 0
.
9
. LLC only needs
460
−
30 = 430
units to pass quality control to satisfy the week’s demand. If LLC starts with
C
= 600
units, then with
the yield of
0
.
9
, the number of units that will pass quality control woud be
600
×
0
.
9 = 540
. In this case
LLC would have to carry an ending inventory of
540
−
430 = 110
units. However, LLC can only carry
T
= 50
units. Therefore the number of starts will be adjusted to
430+50
0
.
9
≈
533
. The total cost incurred
in the week would be
600
×
$100
for regular capacity (even though we don’t use all the capacity) plus
50
×
$30
inventory holding cost. There is no overtime cost.
7
Your preview ends here
Eager to read complete document? Join bartleby learn and gain access to the full version
- Access to all documents
- Unlimited textbook solutions
- 24/7 expert homework help
Business 36106 (Autumn 2023)
Final (Solutions)
Example 2
: The week begins with
I
n
−
1
=10 units carried from the previous week, and a demand of
D
n
= 510
units. Let the random yield for this month be
Y
n
= 0
.
75
. LLC only needs
510
−
10 = 500
units to pass quality control to satisfy the week’s demand. If LLC starts with
C
= 600
units, then with
the yield of
0
.
75
, the number of units that will pass quality control woud be
600
×
0
.
75 = 450
. This
is not enough to meet the demand for the current week. In this case LLC would need to use overtime
production to meet the deficity of
500
−
450 = 50
units.
Given the yield of
0
.
75
, this means LLC
would need to start overtime production with
50
0
.
75
≈
67
starts.
The total cost incurred in the week
would be
600
×
$100
for regular capacity, plus
67
×
$400
overtime cost. There is no inventory holding cost.
We will assume that the manufacturing process runs for 20 weeks (so your simulation model should be
for 20 weeks), we begin with an inventory of 0 in Week 1, and we would like to end with an inventory
of 0 at the end of Week 20. In your simulation model, do not worry about rounding demand, number
of units started, and production to integers.
(a)
[5 pts]
Your first task is to build a simulation model to estimate the distribution of the total
cost for a given choice of regular production capacity
C
, and inventory threshold
T
. For now, set
C
= 625
and
T
= 50
. Set number of iterations to 1000.
Answer:
What is the mean of the total cost for 20 weeks (report 90% confidence interval)?
1,423,426
±
4,006
What are the 10th and 90th percentiles of the total cost?
1,311,594 ; 1,558,120.
(b)
[2 pts]
Use RiskSimTable to try
C
=
{
600
,
625
,
650
,
675
}
and
T
=
{
50
,
75
,
100
,
125
,
150
}
. Re-
port the best combination that minimizes the mean total cost, and the mean cost under this
combination based on your simulation.
Answer:
Among these, the best combination I found was
C
= 650
, T
= 100
. The 90% confidence interval
for the mean total cost is 1,385,937
±
2,184.
8
Business 36106 (Autumn 2023)
Final (Solutions)
4
Allocating Production Personnel at LLC [11 pts]
Download the workbook
LLC
staffing
data.xlsx
from Canvas.
Build your Solver model in
this workbook and upload to Canvas along with the rest of your submission.
When Julie Weller joined LLC, the company only produced high-end components in its product line.
Much of the assembly was handled by highly-skilled operators, many of whom had been with LLC for
7-10 years. On the contrary, their two recent acquisitions had been in less complex products, with more
dependence on automated assembly techniques and equipment, and less dependence on skilled workers.
LLC’s Manufacturing Department had made good progress over the past 18 months with the integration
of the two companies. The move to the larger facility that housed all three assembly lines had gone
smoothly. Operational metrics, yields, cycle times, delivery schedules were maintained throughout the
transition.
Although Julie was proud of her team’s progress, she was confident that the operational
efficiency could be improved further.
As a result of the acquisitions, LLC’s three production lines had different types of workers, with different
levels of skills, productivity, and wage scales. Table 1 in the excel workbook summarizes this information.
Table 2 shows the hourly allocation of the workers across pay grades and product lines given that each
operator works an average of 40 hours per week.
Given the fluctuations in demand, Julie had felt strongly that operators across all three product lines
should be cross-trained on each line’s processes. That way, if demand for one product line falls, those
operators would be able to help out on another line.
After several months, all operators had been
cross-trained on all of the processes across the three production lines.
Despite the cross-training, Steve Lo (Manufacturing Engineering Manager) noticed that there were still
productivity differences between the workers, largely related to the experience and skill of the operators
at the different pay grades. After reviewing the production records over the past several months, Steve
put together the productivity table shown in Table 3.
Table 4 shows the weekly production plan for next quarter that is used for planning purposes.
While Julie was confident that she could meet this production plan with her current staffing and alloca-
tion, she suspected that she could more efficiently utilize the workers. With the current allocation, there
was no room for error; the workers were allocated 100% of their time. E.g., with the current allocation of
workers to Long-Haul Telecom, the production capacity equals
160
×
2
.
00 + 360
×
1
.
80 + 600
×
1
.
62 =
1940
, which exactly matches the planned production of Long-Haul Telecom parts.
There was thus
neither time for other projects, nor spare capacity (which often came in handy during end-of-quarter
production rushes).
Your goal is to build a
linear Solver models (and use SimplexLP as the solution method)
to help
with several variants of the staffing problem that Julie is contemplating.
(a)
[3.5 pts]
Identify the most efficient allocation of the
existing staff
required to meet the production
plan.
While it is true that the total wages paid is constant,
minimize the direct labor cost
required to meet this production plan. You can assume that the number of hours allocated can
be fractional, and that the hours of one employee can be split across multiple product lines. How
9
Business 36106 (Autumn 2023)
Final (Solutions)
much money does this reallocation save relative to the existing staffing allocation?
(i) Write down your decision variables, and formulas for objective function and constraints
as mathematical expressions/inequalities involving the symbols you have defined for your
decision variables. Be as precise as possible about the definition of your variables
[0.5 pts]
Decision variables (A):
Answer:
Symbol
Meaning
X
ij
hours allocated from employees of pay grade
i
=
{
1
,
2
, . . . ,
6
}
to
product line
j
=
{
1
,
2
,
3
}
(for Long-Haul, Short-Haul, Datacom)
[0.5 pts] Objective Function (B):
Answer:
Minimize Direct Labor Cost
= 15
×
(
X
11
+
X
12
+
X
13
) + 14
.
50
×
(
X
21
+
X
22
+
X
23
)
+
· · ·
+ 9
.
75
×
(
X
61
+
X
62
+
X
63
)
[0.5 pts]:
Write out the constraints that enforce the condition that the allocation you
find can be satisfied by existing staffing levels.
Answer:
Total hours allocated from pay-grade
i
is at most the number of hours available from
current staffing level of pay-grade
i
. E.g., for pay-grade 1
X
11
+
X
12
+
X
13
≤
160
[0.5 pts]:
Write out the constraint that enforces the condition that the allocation you
find satisfies the planned production of Long-Haul Telecom.
Answer:
The SUMPRODUCT of hours allocated to product line
j
and the productivity of the
different pay grades for product line
j
is at least the production plan for that produce
line. E.g., for Long-Haul
2
.
00
×
X
11
+ 1
.
80
×
X
21
+
· · ·
+ 1
.
3
×
X
61
≥
1940
(ii)
[1.5 pts]
Create a worksheet
‘Solution a’
in the excel workbook, and set up a Solver
model to find the optimal allocation. Write down the direct labor cost of your allocation.
What are the savings compared to the existing allocation?
Answer:
The optimal direct labor cost is:
$99
,
152
.
2
. This is
3
.
15%
smaller than the existing alloca-
tion.
10
Your preview ends here
Eager to read complete document? Join bartleby learn and gain access to the full version
- Access to all documents
- Unlimited textbook solutions
- 24/7 expert homework help
Business 36106 (Autumn 2023)
Final (Solutions)
(b)
[3.5 pts]
Identify a reallocation of the
existing staff
to the three product lines that maximizes
the minimum slack capacity across the three lines. For example, suppose under your reallocation,
the capacities for Long-Haul, Short-Haul, and Datacom are 2000, 1050, 10450 units, then the
minimum slack is
min
2000
1940
,
1050
1000
,
10450
10060
≈
1
.
031
.
Your goal is to find the reallocation that maximizes this minimum slack. You can assume that
the number of hours allocated can be fractional, and that the hours of one employee can be split
across multiple product lines.
(i) Write down all your decision variables, and formulas for objective function and constraints
as mathematical expressions/inequalities involving the symbols you have defined for your
decision variables. Note that this part may require additional decision variables compared to
the previous part. Be as precise as possible about the definition of your variables
[1 pts]
Decision variables (A):
Answer:
Symbol
Meaning
X
ij
hours allocated from employees of pay grade
i
=
{
1
,
2
, . . . ,
6
}
to
product line
j
=
{
1
,
2
,
3
}
(for Long-Haul, Short-Haul, Datacom)
Z
decision variable that stands for the minimum slack across the three
product lines
[0.5 pts] Objective Function (B):
Answer:
Maximize Minimum Slack
=
Z
[0.5 pts]:
Write out the constraints/logic that force your objective to be the minimum
slack across the three product lines.
Answer:
The new decision variable
Z
must be smaller than the slacks for all the three product
lines. Since Solver is maximizing
Z
, at the optimal solution
Z
will take the value of the
minimum slack. E.g., for Long-Haul Telecom:
Z
≤
SUMPRODUCT
(
{
2
,
1
.
8
, . . . ,
1
.
3
}
,
{
X
11
, X
21
, . . . , X
61
}
)
1940
.
(ii)
[1.5 pts]
Create a worksheet
‘Solution b’
in the excel workbook, and set up a Solver
model to find the optimal reallocation. What is the optimal slack of your proposed alloca-
tion?
Answer:
11
Business 36106 (Autumn 2023)
Final (Solutions)
The optimal minimum slack is: 1.032. That is, an extra capacity of 3.2% for each of the
product lines.
(c)
[4 pts]
As with any reorganization initiative, hard decisions must eventually be made regarding
which employees to retain, which employees to lay off, and where to bring in new staff. Identify
the most efficient (i.e., minimum cost) staffing and allocation required to meet the production
plan,
without the constraint of keeping the existing staff
. You can assume that additional
workers at any pay grade are available for hiring, and new workers in each pay grade will have
similar productivity as the existing workers in that pay grade. The numbers of workers hired in
each grade must be integer, and they must be paid wages for the full 40 hours even if they do
not work the full 40 hours. How much money does this reallocation save relative to the existing
staffing allocation?
(i) Write down all your decision variables, and formulas for objective function and constraints
as mathematical expressions/inequalities involving the symbols you have defined for your
decision variables. Note that this part may require additional decision variables compared to
the previous part. Be as precise as possible about the definition of your variables
[0.5 pts]
Decision variables (A):
Answer:
Symbol
Meaning
X
ij
hours allocated from employees of pay grade
i
=
{
1
,
2
, . . . ,
6
}
to
product line
j
=
{
1
,
2
,
3
}
(for Long-Haul, Short-Haul, Datacom)
Y
i
(Integer) number of employees hired for pay grade
i
[0.5 pts] Objective Function (B):
Answer:
Minimize Total Staffing Cost
= 40
×
(15
×
Y
1
+ 14
.
5
×
Y
2
+
· · ·
+ 9
.
75
×
Y
6
)
[1.5 pts]:
Write out the constraints.
Answer:
1. Number of workers hired is non-negative and integer:
Y
i
≥
0
2.
Total number of hours allocated for each paygrade/product combination are non-
negative:
X
ij
≥
0
3. Total number of hours allocated for each paygrade is at most the available hours.
E.g., for paygrade 1:
X
11
+
X
12
+
X
13
≤
40
×
Y
1
.
4. Total capacity available for each product line is at least the planned production. E.g.,
for Long-Haul:
2
.
00
×
X
11
+ 1
.
80
×
X
21
+
· · ·
+ 1
.
3
×
X
61
≥
1940
.
12
Business 36106 (Autumn 2023)
Final (Solutions)
(ii)
[1.5 pts]
Create a worksheet
‘Solution c’
in the excel workbook, and set up a Solver
model to find the optimal staffing and allocation. What is the optimal staffing cost?
Answer:
The optimal minimum cost is:
93280, which is 8.89% smaller than the existing cost of
102,380.
13
Your preview ends here
Eager to read complete document? Join bartleby learn and gain access to the full version
- Access to all documents
- Unlimited textbook solutions
- 24/7 expert homework help
Related Documents
Recommended textbooks for you
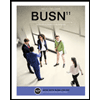
BUSN 11 Introduction to Business Student Edition
Business
ISBN:9781337407137
Author:Kelly
Publisher:Cengage Learning
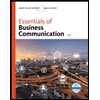
Essentials of Business Communication (MindTap Cou...
Business
ISBN:9781337386494
Author:Mary Ellen Guffey, Dana Loewy
Publisher:Cengage Learning
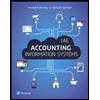
Accounting Information Systems (14th Edition)
Business
ISBN:9780134474021
Author:Marshall B. Romney, Paul J. Steinbart
Publisher:PEARSON
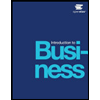
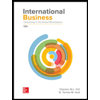
International Business: Competing in the Global M...
Business
ISBN:9781259929441
Author:Charles W. L. Hill Dr, G. Tomas M. Hult
Publisher:McGraw-Hill Education
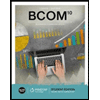
Recommended textbooks for you
- BUSN 11 Introduction to Business Student EditionBusinessISBN:9781337407137Author:KellyPublisher:Cengage LearningEssentials of Business Communication (MindTap Cou...BusinessISBN:9781337386494Author:Mary Ellen Guffey, Dana LoewyPublisher:Cengage LearningAccounting Information Systems (14th Edition)BusinessISBN:9780134474021Author:Marshall B. Romney, Paul J. SteinbartPublisher:PEARSON
- International Business: Competing in the Global M...BusinessISBN:9781259929441Author:Charles W. L. Hill Dr, G. Tomas M. HultPublisher:McGraw-Hill Education
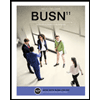
BUSN 11 Introduction to Business Student Edition
Business
ISBN:9781337407137
Author:Kelly
Publisher:Cengage Learning
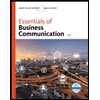
Essentials of Business Communication (MindTap Cou...
Business
ISBN:9781337386494
Author:Mary Ellen Guffey, Dana Loewy
Publisher:Cengage Learning
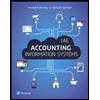
Accounting Information Systems (14th Edition)
Business
ISBN:9780134474021
Author:Marshall B. Romney, Paul J. Steinbart
Publisher:PEARSON
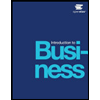
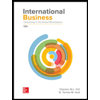
International Business: Competing in the Global M...
Business
ISBN:9781259929441
Author:Charles W. L. Hill Dr, G. Tomas M. Hult
Publisher:McGraw-Hill Education
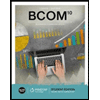