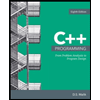
C++ Programming: From Problem Analysis to Program Design
8th Edition
ISBN: 9781337102087
Author: D. S. Malik
Publisher: Cengage Learning
expand_more
expand_more
format_list_bulleted
Question
My
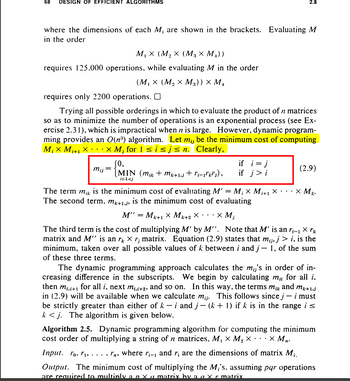
Transcribed Image Text:68 DESIGN OF EFFICIENT ALGORITHMS
2.8
where the dimensions of each M, are shown in the brackets. Evaluating M
in the order
M₁ X (MX (M3 × M₁))
requires 125.000 operations, while evaluating M in the order
(M, X (MM;)) × M₁
requires only 2200 operations. ☐
Trying all possible orderings in which to evaluate the product of n matrices
so as to minimize the number of operations is an exponential process (see Ex-
ercise 2.31), which is impractical when n is large. However, dynamic program-
ming provides an O(n³) algorithm. Let m₁, be the minimum cost of computing
M₁ × Mi+1 × ·× M; for 1 ≤ i ≤ j ≤n. Clearly,
(0,
if i=j
(2.9)
mij =
(MIN (mik + M+1, +1-1),
if j>i
ish<j
The term m is the minimum cost of evaluating M' = M; × M¡+1 × · · · × Mk.
The second term, m+1.), is the minimum cost of evaluating
M = M+Mk+2 × · · · × M;
The third term is the cost of multiplying M' by M". Note that M' is an ri-1 × rk
matrix and M" is an r X r; matrix. Equation (2.9) states that mij, j > i, is the
minimum, taken over all possible values of k between i and j- 1, of the sum
of these three terms.
The dynamic programming approach calculates the m₁'s in order of in-
creasing difference in the subscripts. We begin by calculating m₁ for all i,
then mi,i+1 for all i, next mi,i+2, and so on. In this way, the terms m₁ and mk+1,j
in (2.9) will be available when we calculate mij. This follows since j - i must
be strictly greater than either of k-i and j― (k+1) if k is in the range i ≤
k<j. The algorithm is given below.
Algorithm 2.5. Dynamic programming algorithm for computing the minimum
cost order of multiplying a string of n matrices, M₁ × M₂ ×···× M„.
Input. Fo...", where ;-, and r; are the dimensions of matrix M₁.
Output. The minimum cost of multiplying the M,'s, assuming pqr operations
are required to multiply an X a matrix by a a X r matrix.
![2.8 DYNAMIC PROGRAMMING
Recursive techniques are useful if a problem can be divided into subproblems
with reasonable effort and the sum of the sizes of the subproblems can be
kept small. Recall from Theorem 2.1 that if the sum of the sizes of the sub-
problems is an, for some constant a > 1, the recursive algorithm is likely to
be polynomial in time complexity. However, if the obvious division of a prob-
lem of size n results in n problems of size n - 1, then a recursive algorithm is
likely to have exponential growth. In this case a tabular technique called
dynamic programming often results in a more efficient algorithm.
In essence, dynamic programming calculates the solution to all subprob-
lems. The computation proceeds from the small subproblems to the larger
subproblems, storing the answers in a table. The advantage of the method
lies in the fact that once a subproblem is solved, the answer is stored and
never recalculated. The technique is easily understood from a simple example.
Consider the evaluation of the product of n matrices
M = M₁x M₂ × · · · × M„
where each M, is a matrix with 1-1 rows and r; columns. The order in which
the matrices are multiplied together can have a significant effect on the total
number of operations required to evaluate M, no matter what matrix multi-
plication algorithm is used.
Example 2.7. Assume that the multiplication of a p× q matrix by a q× r
matrix requires pqr operations, as it does in the "usual" algorithm. and con-
sider the product
M =
M₁
[10 × 20]
M2
[20 x 50]
M3
[501]
MA
(2.8)
[1 × 100]](https://content.bartleby.com/qna-images/question/20f20d76-365f-4e43-a779-2a5ce112e22f/11d21cc1-eb3f-474b-8ada-54109b85257e/4sg69xe_thumbnail.png)
Transcribed Image Text:2.8 DYNAMIC PROGRAMMING
Recursive techniques are useful if a problem can be divided into subproblems
with reasonable effort and the sum of the sizes of the subproblems can be
kept small. Recall from Theorem 2.1 that if the sum of the sizes of the sub-
problems is an, for some constant a > 1, the recursive algorithm is likely to
be polynomial in time complexity. However, if the obvious division of a prob-
lem of size n results in n problems of size n - 1, then a recursive algorithm is
likely to have exponential growth. In this case a tabular technique called
dynamic programming often results in a more efficient algorithm.
In essence, dynamic programming calculates the solution to all subprob-
lems. The computation proceeds from the small subproblems to the larger
subproblems, storing the answers in a table. The advantage of the method
lies in the fact that once a subproblem is solved, the answer is stored and
never recalculated. The technique is easily understood from a simple example.
Consider the evaluation of the product of n matrices
M = M₁x M₂ × · · · × M„
where each M, is a matrix with 1-1 rows and r; columns. The order in which
the matrices are multiplied together can have a significant effect on the total
number of operations required to evaluate M, no matter what matrix multi-
plication algorithm is used.
Example 2.7. Assume that the multiplication of a p× q matrix by a q× r
matrix requires pqr operations, as it does in the "usual" algorithm. and con-
sider the product
M =
M₁
[10 × 20]
M2
[20 x 50]
M3
[501]
MA
(2.8)
[1 × 100]
Expert Solution

This question has been solved!
Explore an expertly crafted, step-by-step solution for a thorough understanding of key concepts.
Step by stepSolved in 2 steps

Knowledge Booster
Similar questions
- (Practice) State whether the following are valid function names and if so, whether they’re mnemonic names that convey some idea of the function’s purpose. If they are invalid names, state why. powerdensity m1234 newamp 1234 abcd total tangent absval computed b34a 34ab volts$ a2B3 while minVal sine $sine cosine speed netdistance sum return stackarrow_forward(Numerical analysis) Here’s a challenging problem for those who know a little calculus. The Newton-Raphson method can be used to find the roots of any equation y(x)=0. In this method, the (i+1)stapproximation,xi+1,toarootofy(x)=0 is given in terms of the ith approximation, xi, by the following formula, where y’ denotes the derivative of y(x) with respect to x: xi+1=xiy(xi)/y(xi) For example, if y(x)=3x2+2x2,theny(x)=6x+2 , and the roots are found by making a reasonable guess for a first approximation x1 and iterating by using this equation: xi+1=xi(3xi2+2xi2)/(6xi+2) a. Using the Newton-Raphson method, find the two roots of the equation 3x2+2x2=0. (Hint: There’s one positive root and one negative root.) b. Extend the program written for Exercise 6a so that it finds the roots of any function y(x)=0, when the function for y(x) and the derivative of y(x) are placed in the code.arrow_forward(Program) Write a program that tests the effectiveness of the rand() library function. Start by initializing 10 counters, such as zerocount, onecount, twocount, and so forth, to 0. Then generate a large number of pseudorandom integers between 0 and 9. Each time 0 occurs, increment zerocount; when 1 occurs, increment onecount; and so on. Finally, display the number of 0s, 1s, 2s, and so on that occurred and the percentage of time they occurred.arrow_forward
- (Thermodynamics) The work, W, performed by a single piston in an engine can be determined by this formula: W=Fd F is the force provided by the piston in Newtons. d is the distance the piston moves in meters. a. Determine the units of W by calculating the units resulting from the right side of the formula. Check that your answer corresponds to the units for work listed in Table 1.1. b. Determine the work performed by a piston that provides a force of 1000 N over a distance of 15 centimeters.arrow_forward(Practice) Determine names for functions that do the following: a. Find the average of a set of numbers. b. Find the area of a rectangle. c. Find the minimum value in a set of numbers. d. Find the density of a steel door. e. Sort a set of numbers from lowest to highestarrow_forward
arrow_back_ios
arrow_forward_ios
Recommended textbooks for you
- C++ Programming: From Problem Analysis to Program...Computer ScienceISBN:9781337102087Author:D. S. MalikPublisher:Cengage LearningOperations Research : Applications and AlgorithmsComputer ScienceISBN:9780534380588Author:Wayne L. WinstonPublisher:Brooks ColeC++ for Engineers and ScientistsComputer ScienceISBN:9781133187844Author:Bronson, Gary J.Publisher:Course Technology Ptr
- Systems ArchitectureComputer ScienceISBN:9781305080195Author:Stephen D. BurdPublisher:Cengage LearningLINUX+ AND LPIC-1 GDE.TO LINUX CERTIF.Computer ScienceISBN:9781337569798Author:ECKERTPublisher:CENGAGE LProgramming Logic & Design ComprehensiveComputer ScienceISBN:9781337669405Author:FARRELLPublisher:Cengage
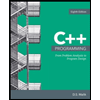
C++ Programming: From Problem Analysis to Program...
Computer Science
ISBN:9781337102087
Author:D. S. Malik
Publisher:Cengage Learning
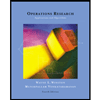
Operations Research : Applications and Algorithms
Computer Science
ISBN:9780534380588
Author:Wayne L. Winston
Publisher:Brooks Cole
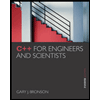
C++ for Engineers and Scientists
Computer Science
ISBN:9781133187844
Author:Bronson, Gary J.
Publisher:Course Technology Ptr
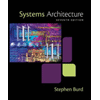
Systems Architecture
Computer Science
ISBN:9781305080195
Author:Stephen D. Burd
Publisher:Cengage Learning
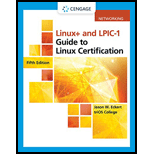
LINUX+ AND LPIC-1 GDE.TO LINUX CERTIF.
Computer Science
ISBN:9781337569798
Author:ECKERT
Publisher:CENGAGE L
Programming Logic & Design Comprehensive
Computer Science
ISBN:9781337669405
Author:FARRELL
Publisher:Cengage