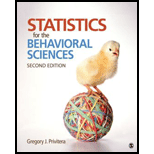
Concept explainers
(a)
Complete the F table.
Make a decision to retain or reject the null hypothesis that the multiple regression equation can be used to significantly predict health.
(a)

Answer to Problem 28CAP
The completed F table is,
Source of variation | SS | df | MS | |
Regression | 126.22 | 2 | 63.11 | 14.48 |
Residual (error) | 21.78 | 5 | 4.36 | |
Total | 148.00 | 7 |
The decision is to reject the null hypothesis.
Theregression equation significantly predicts variance in criterion variable (Y) health [BMI].
Explanation of Solution
Calculation:
The given information is that, the researcher has tested whether ‘daily intake of fat (in grams)’ and ‘amount of exercise (in minutes)’ can predict health (measured using a body mass index [BMI] scale.
The formula for test statistic is,
Decision rules:
- If the test statistic value is greater than the critical value, then reject the null hypothesis
- If the test statistic value is smaller than the critical value, then retain the null hypothesis
Null hypothesis:
Alternative hypothesis:
Software procedure:
Step by step procedure to obtain test statistic value using SPSS software is given as,
- Choose Variable view.
- Under the name, enter the name as Fat, Exercise, Health.
- Choose Data view, enter the data.
- Choose Analyze>Regression>Linear.
- In Dependents, enter the column of Health.
- In Independents, enter the column of Fat, and Exercise.
- Click OK.
Output using SPSS software is given below:
The table of F is,
Source of variation | SS | df | MS | |
Regression | 126.22 | 2 | 63.11 | 14.48 |
Residual (error) | 21.78 | 5 | 4.36 | |
Total | 148.00 | 7 |
Table 1
Critical value:
The considered significance level is
The degrees of freedom for regression are 2, the degrees of freedom for residual are 5.
From the Appendix B: Table B.3-Critical values for F distribution:
- Locate the value 2 in degrees of freedomnumerator row.
- Locate the value 5 in degrees of freedomdenominator row.
- Locate the 0.05 level of significance (value in lightface type) in combined row.
- The intersecting value that corresponds to the (2, 5) with level of significance 0.05 is 5.79.
Thus, the critical value for
Conclusion:
The value of test statistic is 14.48.
The critical value is 5.79.
The test statistic value is greater than the critical value.
The test statistic value falls under critical region.
Hence the null hypothesis is rejected.
Thus, the regression equation significantly predicts variance in criterion variable (Y) health [BMI].
(b)
Determine which predictor variable or variables, when added to the multiple regression equation, significantly contributed to predictions in Y (health).
(b)

Answer to Problem 28CAP
The predictor variable daily intake of fat significantly contributed to predictions in Y (health) when added to the multiple regression equation.
Explanation of Solution
Calculation:
The given information is that, a sample of 8 scores is recorded. The predictor variables are ‘daily intake of fat (in grams)
Relative contribution to fat
Null hypothesis:
Alternative hypothesis:
The predictor variable fat is tested. The other predictor variable that is not tested is ‘Exercise’. Calculate the correlation between ‘Exercise’ and ‘Health[BMI]’.
Software procedure:
Step by step procedure to obtain correlation using SPSS software is given as,
- Choose Variable view.
- Under the name, enter the name as Health, Exercise.
- Choose Data view, enter the data.
- Choose Analyze>Correlate>Bivariate.
- In variables, enter the Health, and Exercise.
- Click OK.
Output using SPSS software is given below:
The correlation between ‘Exercise’ and ‘Health [BMI]’ is –0.789.
The value of
The formula for
The calculation of sums of squares is,
Health [BMI] (Y) | |
32 | 1,024 |
34 | 1,156 |
23 | 529 |
33 | 1,089 |
28 | 784 |
27 | 729 |
25 | 625 |
22 | 484 |
Table 2
Substitute,
The contribution of
From the F table, the value of
The contribution of
Reproduce the F table by replacing,
Since there would be only one predictor variable, the degrees of freedom for regression would be 1.
The mean sums of squares for regression is,
The F statistic value is,
The change F table with
Source of variation | SS | df | MS | |
Regression | 34.09 | 1 | 34.09 | 7.82 |
Residual (error) | 21.78 | 5 | 4.36 | |
Total | 148.00 | 7 |
Critical value:
The considered significance level is
The degrees of freedom for regression are 1, the degrees of freedom for residual are 5.
From the Appendix B: Table B.3-Critical values for F distribution:
- Locate the value 1 in degrees of freedom numerator row.
- Locate the value 5 in degrees of freedom denominator row.
- Locate the 0.05 level of significance (value in lightface type) in combined row.
- The intersecting value that corresponds to the (1, 5) with level of significance 0.05 is 6.61.
Thus, the critical value for
Conclusion:
The value of test statistic is 7.82.
The critical value is 6.61.
The test statistic value is greater than the critical value.
The test statistic value falls under critical region.
Hence the null hypothesis is rejected.
Thus, adding fat
Relative contribution to exercise
Null hypothesis:
Alternative hypothesis:
The predictor variable exercise is tested. The other predictor variable that is not tested is ‘fat’. Calculate the correlation between ‘fat’ and ‘Health [BMI]’.
Software procedure:
Step by step procedure to obtain correlation using SPSS software is given as,
- Choose Variable view.
- Under the name, enter the name as Health, Fat.
- Choose Data view, enter the data.
- Choose Analyze>Correlate>Bivariate.
- In variables, enter the Health, and Fat.
- Click OK.
Output using SPSS software is given below:
The correlation between ‘fat’ and ‘Health [BMI]’ is 0.923.
The value of
The value for
From the F table, the value of
The contribution of
Reproduce the F table by replacing,
Since there would be only one predictor variable, the degrees of freedom for regression would be 1.
The mean sums of squares for regression is,
The F statistic value is,
The change F table with
Source of variation | SS | df | MS | |
Regression | 0.14 | 1 | 0.14 | 0.03 |
Residual (error) | 21.78 | 5 | 4.36 | |
Total | 148.00 | 7 |
The critical value for F table with
Conclusion:
The value of test statistic is 0.03.
The critical value is 6.61.
The test statistic value is less than the critical value.
The test statistic value does not fall under critical region.
Hence the null hypothesis is retained.
Thus, adding exercise
Hence, the predictor variable daily intake of fat significantly contributed to predictions in Y (health) when added to the multiple regression equation.
Want to see more full solutions like this?
Chapter 16 Solutions
Statistics for the Behavioral Sciences
- Olympic Pole Vault The graph in Figure 7 indicates that in recent years the winning Olympic men’s pole vault height has fallen below the value predicted by the regression line in Example 2. This might have occurred because when the pole vault was a new event there was much room for improvement in vaulters’ performances, whereas now even the best training can produce only incremental advances. Let’s see whether concentrating on more recent results gives a better predictor of future records. (a) Use the data in Table 2 (page 176) to complete the table of winning pole vault heights shown in the margin. (Note that we are using x=0 to correspond to the year 1972, where this restricted data set begins.) (b) Find the regression line for the data in part ‚(a). (c) Plot the data and the regression line on the same axes. Does the regression line seem to provide a good model for the data? (d) What does the regression line predict as the winning pole vault height for the 2012 Olympics? Compare this predicted value to the actual 2012 winning height of 5.97 m, as described on page 177. Has this new regression line provided a better prediction than the line in Example 2?arrow_forwardA psychological study aimed at predicting Al Ain secondary school students’ mental health scores via their scores in the life satisfaction scale. The researcher examines the following null hypothesis: there is no significant relationship between Al Ain secondary school students’ mental health and their life satisfaction scores at 0.05 level of significance. Use the following data to establish the required regression equation. Student # Mental health score out of 50 Life- satisfaction score out of 100 1 40 80 2 41 87 3 34 90 4 30 78 5 44 89 6 42 85 7 45 88 8 32 77 9 47 90 10 22 57 11 30 78 12 28 77 13 35 76 14 40 84 15 31 76 16 39 80 17 41 84 18 24 60 19 22 50 20 37 75 21 40 80 22 38 78 23 29 60 24 24 55 25 24 62 26 29 61 27 32 65 28 34 66 29 28 67…arrow_forwardA mail-order business selling personal computer supplies, software and hardware maintains a centralized warehouse. Management is currently examining the process of distribution from the warehouse and wants to study the factors that affect the warehouse distribution costs. Data collected over 24 random months contain the warehouse’s distribution cost (in thousands of Rands), the sales (in thousands of Rands) and the number of orders received. A multiple linear regression model was fitted to the data by using Stat1.2. Use the output to answer the questions that follow by typing only the letter of the correct option in the answer boxes. Variablesy: Warehouse Distribution Costx1: Salesx2: Number of Orders Model Fitting StatisticsR2 = 0.8504Adj R2: ? Regression Coefficients Beta Parameter Standard b Parameter Standard Estimates…arrow_forward
- Do students with higher college grade point averages (GPAs) earn more than those graduates with lower GPAs?† Consider the following hypothetical college GPA and salary data (10 years after graduation). GPA Salary ($) 2.22 72,000 2.27 48,000 2.57 72,000 2.59 62,000 2.77 86,000 2.85 96,000 3.12 133,000 3.35 130,000 3.66 157,000 3.68 162,000 #1) Use these data to develop an estimated regression equation that can be used to predict annual salary 10 years after graduation given college GPA. (Let x = GPA, and let y = salary (in $). Round your numerical values to the nearest integer.) ŷ = #2) Find the value of the test statistic. (Round your answer to two decimal places.) #3)Find the p-value. (Round your answer to three decimal places.) p-value =arrow_forwarda) For United States, provide data for the variables below over the years 1993 – 2007: (i) Net migration rate (per 1,000 population) (ii) Total fertility rate (live births per woman) (iii)Unemployment, general level (Thousands) (iv) Wages (v) Life expectancy at birth for both sexes combined (years) Data can be obtained from the UN database http://data.un.org/Explorer.aspx Using R-Studio, estimate a regression equation to determine the effect of unemployment, general level, wages and life expectancy at birth for both sexes on the net migration rate. (All codes and regression output should be provided).(i) Write down the regression equation. (ii) Interpret the coefficients and determine which of the individual coefficients in theregression model are statistically significant. In responding, construct and test anyappropriate hypothesis. (iii) Interpret the coefficient of determination. (iv) Using the 10% level of significance, determine and discuss whether the overallregression equation…arrow_forward(a) For United States, provide data for the variables below over the years 1993 – 2007: (i) Net migration rate (per 1,000 population) (ii) Total fertility rate (live births per woman) (iii)Unemployment, general level (Thousands) (iv) Wages (v) Life expectancy at birth for both sexes combined (years) Data can be obtained from the UN database http://data.un.org/Explorer.aspx Using R-Studio, estimate a regression equation to determine the effect of unemployment, general level, wages and life expectancy at birth for both sexes on the net migration rate. (All codes and regression output should be provided).(b) Using R-Studio redo the regression analysis with the total fertility rate as an additionalindependent variable. (All codes and regression output should be provided).(i) Write down the regression equation. (ii) Use the 5% level of significance, determine and discuss whether the total fertilityrate has a significant impact on the net migration rate in your assigned country.…arrow_forward
- (a) For United States, provide data for the variables below over the years 1993 – 2007: (i) Net migration rate (per 1,000 population) (ii) Total fertility rate (live births per woman) (iii)Unemployment, general level (Thousands) (iv) Wages (v) Life expectancy at birth for both sexes combined (years) Data can be obtained from the UN database http://data.un.org/Explorer.aspx Using R-Studio, estimate a regression equation to determine the effect of unemployment, general level, wages and life expectancy at birth for both sexes on the net migration rate. (All codes and regression output should be provided). (iv) Using the 10% level of significance, determine and discuss whether the overall regression equation is statistically significant. In responding, construct and test any appropriate hypothesis. (v) Determine and interpret the confidence interval for the independent variable(s).arrow_forwardThe Mayor of texas whom is partners with a local agriculturalist wants to know how the amount of fertilizer and the amount of water given to plants affect their growth. The results were inputted into MINITAB so as to fit the model a) Write out the regression equation b) What is the sample size used in this investigation? c) Determine the values of *, ** and ***, **** d) Conduct a hypothesis test, at the 5% level of significance, to determine whether ? is significant. e) What would be the growth of the plant if 4g of fertilizer and 7g of ater was given to it daily? f) Carry out an F -test at the 1% significance level to determine whether the model is significantarrow_forwardThe owner of Showtime Movie Theaters, Inc. would like to predict weekly gross revenue as a function of advertising expenditures. Historical data for a sample of eight weeks follow. Weekly GrossRevenue($1000s) TelevisonAdvertising($1000s) NewspaperAdvertising($1000s) Part a 96 5.0 1.5 Develop an estimated regression equation with the amount of television advertising as the independent variable. 90 2.0 2.0 95 4.0 1.5 92 2.5 2.5 95 3.0 3.3 94 3.5 2.3 94 2.5 4.2 94 3.0 2.5 Part b Develop an estimated regression equation with both television advertising and news paper advertising as independent variables.…arrow_forward
- The use of multiple logistic regression is warranted when there are two or more independent quantitative or nominal variables and one dichotomous dependent variable. a. True b. Falsearrow_forwardGiven a generic data set (x,y) with a linear regression. How do you determine if the y(dependent) will be less/greater than a certain value at a decided value of x?arrow_forwardA statistics consulting center at a major university analyzed data on normal woodchucks for the university's veterinary school. The variables of interest were body weight in grams and heart weight in grams. It was desired to develop a linear regression equation in order to determine if there is a significant linear relationship between heart weight and total body weight. Use this information to answer the questions. Determine the test statistic. Body Weight Heart Weight4050 11.12435 10.13135 15.85740 10.92555 10.73665 11.42035 13.44260 13.92990 11.54935 15.83690 10.12880 12.82760 10.62160 15.42360 14.82040 13.62055 12.92650 15.62665 13.8arrow_forward
- College AlgebraAlgebraISBN:9781305115545Author:James Stewart, Lothar Redlin, Saleem WatsonPublisher:Cengage Learning
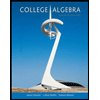