INTRODUCTION TO STATISTICAL LEARNING
21st Edition
ISBN: 9781071614174
Author: James
Publisher: SPRINGER
expand_more
expand_more
format_list_bulleted
Expert Solution & Answer
Chapter 3, Problem 11E
a.
Explanation of Solution
Simple linear regression
- A simple linear regression is performed on y onto x without an intercept...
b.
Explanation of Solution
Simple linear regression
- A simple linear regression is performed on y onto x without an intercept...
c.
Explanation of Solution
Simple linear regression
- The same value is obtained for the t-statistic and consequently the same value for the corresponding p-value...
d.
Explanation of Solution
Simple linear regression
- The regression of Y onto X without an intercept.
- Hence the result is verified numerically...
e.
Explanation of Solution
Simple linear regression
- It is easy to see that if xi is replac...
f.
Explanation of Solution
Simple linear regression
- Here the regression is performed with an intercept...
Expert Solution & Answer

Want to see the full answer?
Check out a sample textbook solution
Students have asked these similar questions
in a trained a logistic regression classifier. it outputs a new example x with a prediction ho(x) = 0.3. This means:
Select one:
Oa. Our estimate for P(y-1 | x)
Ob. Our estimate for Ply-0 | x)
Oc. Our estimate for P(y-1 | x)
Od. Our estimate for P(y=0 | x)
Consider a linear regression setting. Given a model's weights W E RD, we incorporate regularisation
into the loss function by adding an la regularisation function of the form-W;|*. Select all true
statements from below.
a. When q = 1, a solution to this problem tends to be sparse. I.e., most weights are driven to zero
with only a few weights that are not close to zero.
b. When q = 2, a solution to this problem tends to be sparse. I.e., most weights are driven to zero
with only a few weights that are not close to zero.
c. When q = 1, the problem can be solved analytically as in closed form.
d. When q = 2, the problem can be solved analytically as in closed form.
Linear regression aims to fit the parameters based on the training set
T.x =
1, 2,...,m} so that the hypothesis function he (x)
...... + Onxn can better predict the output y of a new input vector
x. Please derive the stochastic gradient descent update rule which can update
repeatedly to minimize the least squares cost function J(0).
D =
{(x(i),y(¹)), i
00+ 01x₁ + 0₂x₂+...
=
=
Chapter 3 Solutions
INTRODUCTION TO STATISTICAL LEARNING
Knowledge Booster
Similar questions
- For logistic regression, the gradient of the cost function is given by J(0) = (i) E (he (x) – y')x;). Write down mathematical expression(s) for the correct m gradient descent update for logistic regression with a learning rate of a. (In the expression, he(x^) should be replaced by the sigmoid function.)arrow_forwardIn R, write a function that produces plots of statistical power versus sample size for simple linear regression. The function should be of the form LinRegPower(N,B,A,sd,nrep), where N is a vector/list of sample sizes, B is the true slope, A is the true intercept, sd is the true standard deviation of the residuals, and nrep is the number of simulation replicates. The function should conduct simulations and then produce a plot of statistical power versus the sample sizes in N for the hypothesis test of whether the slope is different than zero. B and A can be vectors/lists of equal length. In this case, the plot should have separate lines for each pair of A and B values (A[1] with B[1], A[2] with B[2], etc). The function should produce an informative error message if A and B are not the same length. It should also give an informative error message if N only has a single value. Demonstrate your function with some sample plots. Find some cases where power varies from close to zero to near…arrow_forwardWe create a simple regression model and call the fit function as follows: Im=LinearRegression() Im.fit(X,Y) in a multilinear model we proceed in the same way: mlm=LinearRegression() mlm.fit(Z,Y) How does the linear regression model knows if we are doing a simple or multiple linear regression? Answer:arrow_forward
- Suppose you are running gradient descent to fit a logistic regression model with 0 E R+1, Which of the following is a reasonable way to make sure the learning rate c is set properly and that gradient descent is running correctly? Plot J(0) as a function of 0 and make sure it is convex. O b. Plot J(8) as a function of 0 and make sure it is decreasing on every iteration. O* Plot /(0) = -E [y®logho(x") + (1 – y®) log (1 – ħø(x"))] a a function of the number of iterations and make sure J(0) is decreasing on every iteration. O d. Plot J(8) =E(h,(x®) – y®)² as a function of the number of iterations (i.e. the horizontal axis is the iteration number) and make sure J(8) is decreasing on every iteration.arrow_forwardIn linear regression, 8 tells us how meaningful the corresponding x is in predicting the output. This means, ...... O if a particular 8-value is very small compared to others, the corresponding x plays a little role in predicting the output. O if a particular 8-value is very large compared to others, the corresponding x plays a little role in predicting the output.arrow_forwardLogistic regression aims to train the parameters from the training set D = {(x(i),y(i)), i 1,2,...,m, y ¤ {0,1}} so that the hypothesis function h(x) = g(0¹ x) 1 (here g(z) is the logistic or sigmod function g(z) can predict the probability of a 1+ e-z new instance x being labeled as 1. Please derive the following stochastic gradient ascent update rule for a logistic regression problem. 0j = 0j + a(y(¹) — hz(x)))x; ave. =arrow_forward
- You run a logistic regression model in R using the glm() function. The dependent variable is the factor variable Y and independent variables are X1 and X2 (in other words, the formula is Y~X1+X2). In the model output, the coefficient of the constant term is a0, the coefficient of X1 is a1, and the coefficient of X2 is a2. Assuming a cutoff = 0.5, which of the following defines the equation of a decision boundary? a0 + a1X1 + a2X2 = 0.5 exp(-(a0 + a1X1 + a2X2)) = 0 a0 + a1X1 + a2X2 = 0 O exp(-(a0 + a1X1 + a2X2)) = 0.5arrow_forwardThe following are all benefits of generalized additive models (GAMS), EXCEPT: GAMS are less computationally demanding than linear regression. GAMS can model non-linear relationships that standard linear regression will miss. GAMS can potentially make more accurate predictions of the response than linear regression can. One can examine the effect of each predictor on the response individually while holding all of the other predictors fixed.arrow_forwardThe task is to implement density estimation using the K-NN method. Obtain an iidsample of N ≥ 1 points from a univariate normal (Gaussian) distribution (let us callthe random variable X) centered at 1 and with variance 2. Now, empirically obtain anestimate of the density from the sample points using the K-NN method, for any valueof K, where 1 ≤ K ≤ N. Produce one plot for each of the following cases (each plotshould show the following three items: the N data points (instances or realizations ofX) and the true and estimated densities versus x for a large number – e.g., 1000, 10000– of discrete, linearly-spaced x values): (i) K = N = 1, (ii) K = 2, N = 10, (iii) K = 10,N = 10, (iv) K = 10, N= 1000, (v) K = 100, N= 1000, (vi) K = N = 50,000. Pleaseprovide appropriate axis labels and legends. Thus there should be a total of six figures(plots),arrow_forward
- Imagine a regression model on a single feature, defined by the function f (x) = wx + b where X, W, and b are scalars. We will use the MSE loss loss(w, b) = E;(f(x;) – t;)² . n Work out the gradient with respect to b. Which is the correct answer? Read the four equations carefully, so you notice all the differences. 1. E:(f(x;) – t;)x; 2.E(f(x;) – t;) n 3.- E;(wx; + b – t;)x; n 4. E: (wa; +b - t;) n 4arrow_forwardIn class, we have defined the hypothesis for univariate linear regression as he(x) = 00 + 0₁x and the mean-square cost function as 2 J(00,01) Σ (ho(x²) - y(i)) ² 2m i=1 We define the following terms: m `x), y= 10. ;Σy"), xy = - ² x(1) y(i) x²: (x(1)) ² m a. Show that the optimal values of the learning parameters 00 and 0₁ are given by: 00 = y-x xy−xy x²-x² 01 = ху-ху x²-x² b. What is the advantage of expressing the learning parameters in this format? =arrow_forwardConsider linear regression where y is our label vector, X is our data matrix, w is our model weights and o² is a measure of variance Using the squared error cost function has a probabilistic interpretation as: O O O Maximising the probability of the model predicting the input data, assuming our input data follows a Normal distribution N(X; Xw, o²) Maximising the probability of the model predicting the input data given the weights N(X; wy, o²) Minimising the probability of the model predicting the labels, assuming our prediction errors follow a Normal distribution N(y; Xw, o²) Maximising the values of the weights to minimise the input data N (y; w, o²) Maximising the probability of the model predicting the labels, assuming our prediction errors follow a Normal distribution N(y; Xw, o²)arrow_forward
arrow_back_ios
SEE MORE QUESTIONS
arrow_forward_ios
Recommended textbooks for you
- Database System ConceptsComputer ScienceISBN:9780078022159Author:Abraham Silberschatz Professor, Henry F. Korth, S. SudarshanPublisher:McGraw-Hill EducationStarting Out with Python (4th Edition)Computer ScienceISBN:9780134444321Author:Tony GaddisPublisher:PEARSONDigital Fundamentals (11th Edition)Computer ScienceISBN:9780132737968Author:Thomas L. FloydPublisher:PEARSON
- C How to Program (8th Edition)Computer ScienceISBN:9780133976892Author:Paul J. Deitel, Harvey DeitelPublisher:PEARSONDatabase Systems: Design, Implementation, & Manag...Computer ScienceISBN:9781337627900Author:Carlos Coronel, Steven MorrisPublisher:Cengage LearningProgrammable Logic ControllersComputer ScienceISBN:9780073373843Author:Frank D. PetruzellaPublisher:McGraw-Hill Education
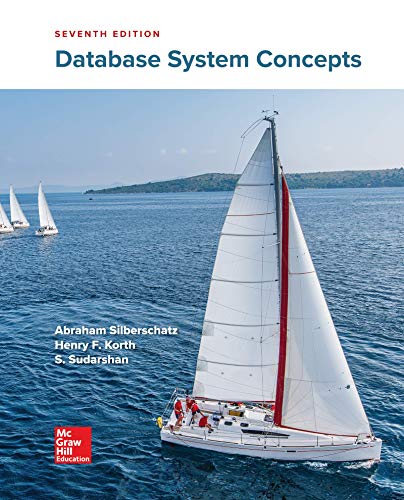
Database System Concepts
Computer Science
ISBN:9780078022159
Author:Abraham Silberschatz Professor, Henry F. Korth, S. Sudarshan
Publisher:McGraw-Hill Education
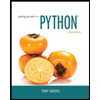
Starting Out with Python (4th Edition)
Computer Science
ISBN:9780134444321
Author:Tony Gaddis
Publisher:PEARSON
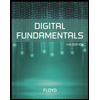
Digital Fundamentals (11th Edition)
Computer Science
ISBN:9780132737968
Author:Thomas L. Floyd
Publisher:PEARSON
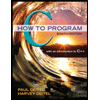
C How to Program (8th Edition)
Computer Science
ISBN:9780133976892
Author:Paul J. Deitel, Harvey Deitel
Publisher:PEARSON
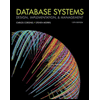
Database Systems: Design, Implementation, & Manag...
Computer Science
ISBN:9781337627900
Author:Carlos Coronel, Steven Morris
Publisher:Cengage Learning
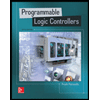
Programmable Logic Controllers
Computer Science
ISBN:9780073373843
Author:Frank D. Petruzella
Publisher:McGraw-Hill Education