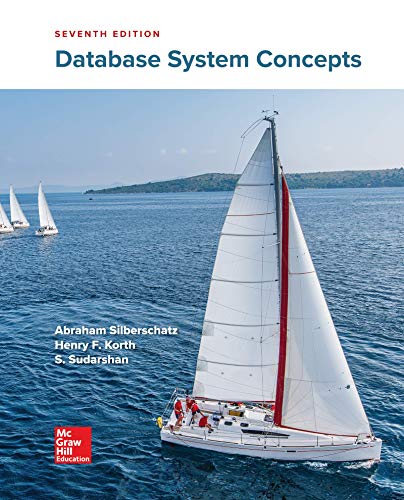
from matplotlib import cm
import matplotlib.pyplot as plt
import platform
import numpy as np
from mlxtend.data import loadlocal_mnist
from sklearn.datasets import load_digits
from sklearn.model_selection import train_test_split
import pickle
plt.rcParams.update({'font.size': 12})
plt.rcParams['figure.figsize'] = [8, 4]
X, y = loadlocal_mnist(images_path='train-images-idx3-ubyte', labels_path='train-labels-idx1-ubyte')
# Keeping only digits 0 and 1
index = y <2
X = X[index]
y = y[index]
# Normalizing the data
X = X/X.max()
# Splitting to training and testing groups.
X_train, X_test, y_train, y_test = train_test_split(X, y, test_size=0.2, shuffle=False)
def sigmoid(t):
reasonable = (t>-100).astype(float)
t = t*reasonable
s = 1/(1+np.exp(-t))
s = s*reasonable
return s
Nsamples,Nfeatures = X_train.shape
Nclasses = 2
a = np.random.randn(Nfeatures+1,Nclasses-1)
Xtilde = np.concatenate((X_train,np.ones((Nsamples,1))),axis=1)
gamma = 1e-1
for iter in range(1500):
# YOUR CODE HERE
a = a - gamma*gradient
if(np.mod(iter,100)==0):
print("Error = ",np.sum(error**2)/)
fig,ax = plt.subplots(1,2)
ax[0].plot(s[:,0:200].T)
ax[0].plot(y_train[0:200])
ax[0].set_title('True and predicted labels')
ax[1].plot(error)
ax[1].set_title('Prediction Errors')
plt.show()
plt.imshow(np.reshape(a[:-1],(28,28)))
plt.title("weights")
Nsamples,Nfeatures = X_test.shape
Xtilde = np.concatenate((X_test,np.ones((Nsamples,1))),axis=1).T
s_test = sigmoid(a.T@Xtilde)
error = (s_test - y_test).T
print(np.sum(error**2))
plt.figure()
plt.plot(s_test[:,400:700].T)
plt.plot(y_test[400:700])
plt.title("True and predicted labels")
plt.show()
plt.figure()
plt.plot(error)
plt.title("Error")
wrong_indices = np.where(np.abs(error[:,0])>0)[0]
Xwrong = X_test[wrong_indices]
fig, axs = plt.subplots(1, len(wrong_indices))
for i in range(len(wrong_indices)):
axs[i].imshow(np.reshape(Xwrong[i],(28,28)))
axs[i].set_title("Misclassified")
This code has only one part missing where your code here is written. I need help with completion of that part. Can someone please help me with the same?

It seems that the missing part involves computing the gradient of the binary logistic regression loss function with respect to the weight parameter vector a
.
Trending nowThis is a popular solution!
Step by stepSolved in 5 steps with 3 images

- In java: containerdata.txt: 438118Vela7335 58025357780Guangzhou7821 49562228320Calumet8268 42566166336Canadian Miner8154 53127688309Empire MacMahon7148 78880272124Costa Marina8216 48293144769Carl D. Bradley7702 69160345120Baie Comeau7928 59558154640W.H. Gilcher7432 52093569191Burns Harbor7304 31962732239Mesabi Miner7906 52416579241SS Minnow7511 32289732723Western Reserve7457 55462342817Osaka Express7015 48816353071Gudrun Mrsk7965 20312110167Georgic8394 51067293443Gallic8294 44519621580Monterey7672 35540101609Napoli8385 58804376662Nuria7619 57902603981Pamela8022 96674603603Robert S Pierson7620 59019259076Sabrina7389 57665569887William C. Moreland8089 55155313818McKay5148 61598 Cargo Container Report. You will be producing a program which will allow the user to generate the container report on the following page in Container Number order or Ship Name order. The program will also the user to lookup the data for any given Container by number. The % cargo weight is the percentage of…arrow_forwardwhy is this function throwing an error? Please show correct versionarrow_forwarddef upgrade_stations(threshold: int, num_bikes: int, stations: List["Station"]) -> int: """Modify each station in stations that has a capacity that is less than threshold by adding num_bikes to the capacity and bikes available counts. Modify each station at most once. Return the total number of bikes that were added to the bike share network. Precondition: num_bikes >= 0arrow_forward
- What are the Javadoc comments for each class? I am strugglingarrow_forwardHere is my entire code(average is not working yet). The response I got from the professor is that I have to create a DRY solution replacing the five display_functions with a single get_array function you call five times like common_array = get_array(plant_xml, "COMMON") etc. from urllib.request import urlopenfrom xml.etree.ElementTree import parse def read_data(data_file): plant_url = urlopen(data_file) plant_xml = parse(plant_url) return plant_xml def display_common_items(plant_xml): plant_root = plant_xml.getroot() common_array = [] for item in plant_root.findall("PLANT"): common_array.append(item.find("COMMON").text) return common_array def display_botanical_items(plant_xml): plant_root = plant_xml.getroot() botanical_array = [] for item in plant_root.findall("PLANT"): botanical_array.append(item.find("BOTANICAL").text) return botanical_array def display_zone_items(plant_xml): plant_root = plant_xml.getroot() zone_array = []…arrow_forwardCode to ::::implement a SnapshotArray that supports the following interface: SnapshotArray(int length) initializes an array-like data structure with the given length. Initially, each element equals 0. void set(index, val) sets the element at the given index to be equal to val. int snap() takes a snapshot of the array and returns the snap_id: the total number of times we called snap() minus 1. int get(index, snap_id) returns the value at the given index, at the time we took the snapshot with the given snap_id Example 1: Input: ["SnapshotArray","set","snap","set","get"] [[3],[0,5],[],[0,6],[0,0]] Output: [null,null,0,null,5] Explanation: SnapshotArray snapshotArr = new SnapshotArray(3); // set the length to be 3 snapshotArr.set(0,5); // Set array[0] = 5 snapshotArr.snap(); // Take a snapshot, return snap_id = 0 snapshotArr.set(0,6); snapshotArr.get(0,0); // Get the value of array[0] with snap_id = 0, return 5...arrow_forward
- import javax.swing.*; import java.util.ArrayList; import java.util.Collections; import java.util.Random; import java.awt.*; import java.awt.event.*; public class memory extends JFrame implements ActionListener { private JButton[] cards; private ImageIcon[] icons; private int[] iconIDs; private JButton firstButton; private ImageIcon firstIcon; private int numMatches; public memory() { setTitle("Memory Matching Game"); setSize(800, 600); setLayout(new BorderLayout()); JPanel boardPanel = new JPanel(new GridLayout(4, 4)); add(boardPanel, BorderLayout.CENTER); icons = new ImageIcon[8]; for (int i = 1; i <= 8; i++) { icons[i-1] = new ImageIcon("image" + i + ".png"); } iconIDs = new int[16]; for (int i = 0; i < 8; i++) { iconIDs[2*i] = i; iconIDs[2*i+1] = i; } Random rand = new Random(); for (int i = 0; i < 16;…arrow_forwardhow to add color to country 10]: Index(['Deaths Per 1000 ', 'Dates', 'Country'], dtype='object')No artists with labels found to put in legend. Note that artists whose label start with an underscore are ignored when lege nd() is called with no argument.Deaths Per 1000 People (2005-2021)arrow_forwardBEFPULSEMIN 70 75 80 82 65 75 74 73 65 67 80 72 69 81 82 83 68 73 74 76 81 64 85 65 66 62 81 82 74 71 72 76 79 80 84 85 73 68 68 67 68 75 77 72 76 78 82 67 80 76 74 77 73 74 72 76 74 81 75 70 TATISTICSSTUDENTSSURVEYFORR contains the column BEFPULSEMIN (a numerical variable that measures student pulses before completing an online survey). For education purposes, consider this dataset to be a sample of size 60 taken from a much wider population for statistics students. Use R to find a 99% confidence interval for the mean of the population. Choose the most correct (closest) statement below. a. Your confidence interval is (72.38912, 76.41088) beats per minute and it is narrower than a 90% confidence interval created from this data. b. Your confidence interval is (72.88831, 75.91169) beats per minute and is narrower than a 90% confidence interval. c. Your…arrow_forward
- Database System ConceptsComputer ScienceISBN:9780078022159Author:Abraham Silberschatz Professor, Henry F. Korth, S. SudarshanPublisher:McGraw-Hill EducationStarting Out with Python (4th Edition)Computer ScienceISBN:9780134444321Author:Tony GaddisPublisher:PEARSONDigital Fundamentals (11th Edition)Computer ScienceISBN:9780132737968Author:Thomas L. FloydPublisher:PEARSON
- C How to Program (8th Edition)Computer ScienceISBN:9780133976892Author:Paul J. Deitel, Harvey DeitelPublisher:PEARSONDatabase Systems: Design, Implementation, & Manag...Computer ScienceISBN:9781337627900Author:Carlos Coronel, Steven MorrisPublisher:Cengage LearningProgrammable Logic ControllersComputer ScienceISBN:9780073373843Author:Frank D. PetruzellaPublisher:McGraw-Hill Education
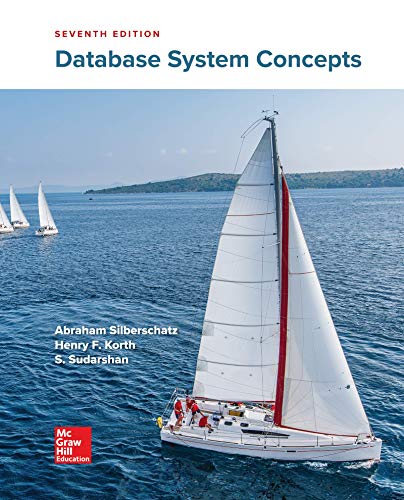
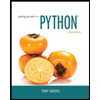
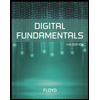
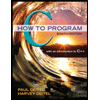
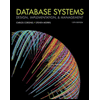
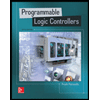