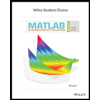
The variable descriptions and Stata outputs from the simple and multiple linear regression are available in the exam handout file. Use this file to answer the following questions.
1. Firstly, report the results from the regression of wage on educ in the form of a fitted line, with the standard error of coefficients presented in parentheses underneath the corresponding coefficients. Round the numbers to two decimal places.
2. Is the coefficient of educ statistically significant?
3. Now consider the multiple linear regression that includes KWW as one of the explanatory variables. Between this regression and the simple linear regression in part (a), which model is more likely to measure the ceteris paribus effect of education on wages? Explain and when possible use evidence to support your answer.
4. What happened to the standard error of educ after adding KWW to the model? Discuss.
- Do you agree or disagree with the following statement? “If the log of the dependent variable appears in the regression, changing the unit of measurement of any independent variable affects both the slope and intercept coefficients”. Discuss
![reg wage educ
Source
Model
Residual
Total
wage
educ
_cons
Source
Model
Residual
Total
educ
KWW
__cons
reg wage educ KWW
reg educ KWW
Source
Model
Residual
Figure (1)
Variable name Variable description
monthly earnings
years of education
Total
wage
educ
KWW
KWW
_cons
SS
16340644.5
136375524
152716168
60.21428
146.9524
SS
23473093.6
129243075
Coefficient Std. err.
152716168
General test of work-
related skills
SS
Figure (2)
678.944165
3827.87509
df
wage Coefficient Std. err.
4506.81925
16340644.5
1
933 146168.836
934 163507.675
5.694982
77.71496
Figure (3)
df
43.46013 6.018896
12.41303 1.730828
-71.09109 81.57352
934
2 11736546.8
932 138672.827
Figure (4)
MS
df
educ Coefficient Std. err.
t P>|t|
.1116141 .0086764
9.478871 .3171285
10.57
0.000
1.89 0.059
MS
163507.675
678.944165
1
933 4.10276001
MS
934 4.82528828
Number of obs
F(1, 933)
Prob> F
R-squared
Adj R-squared
Root MSE
t P>|t|
7.22 0.000
7.17 0.000
-0.87 0.384
t
Number of obs
F(2, 932)
Prob F
R-squared
Adj R-squared
Root MSE
P>|t|
12.86 0.000
29.89 0.000
49.03783
-5.56393
=
31.64797
9.016253
-231.1802
=
=
=
[95% conf. interval]
.0945866
8.856503
=
=
=
=
Number of obs
F(1, 933)
Prob > F
R-squared
Adj R-squared =
Root MSE
=
=
[95% conf. interval]
55.27229
15.8098
88.99797
=
935
111.79
0.0000
0.1070
0.1060
382.32
=
=
71.39074
299.4688
935
84.63
0.0000
0.1537
0.1519
372.39
935
165.48
0.0000
0.1506
0.1497
2.0255
[95% conf. interval]
.1286417
10.10124](https://content.bartleby.com/qna-images/question/244be6eb-07da-4aa9-92ad-dac5735e946a/1fa67b11-b3ac-4af5-a593-a76f674433f7/waz4ks_thumbnail.png)

Step by stepSolved in 5 steps with 5 images

- The regression line for the given data is = 6.91x + 46.26. Determine the residual of a data point for which x = 4 and y = 75.arrow_forwardLet price denote a price index for the goods sold by a restaurant, advert the amount spent on advertising, sales the sales for the restaurant, and consider the following two regressions First regression: sales = B1 + B2price + B3price? + B4advert + ßsadvert? + e, Second regression: sales = B1 + B2price + B3price? + e We estimate both regressions using a sample of 105 observations. The sum of square residuals (E ê) from the first regression equals 50, while the sum of square Li=1 residuals from the second regression equals 70. Suppose we are interested in testing the null hypothesis that expected sales do not depend on advertising. What is the F- statistic for this null hypothesis? Recall the F-statistic is given by ((SSER - SSEU)/J)/(SSEy/(n – K)). O a. -15 O b. 42 Oc. 21 O d. 20 O e. All other options are incorrect.arrow_forwardFor a multiple regression analysis with 2 and 12 degrees of freedom, MS regression is 135 and MS residual is 15. What is the decision for this test?arrow_forward
- There are 6 parts for this Question. Please helparrow_forwardI recently asked this question and was wondering if you could show me how to do it, not just putting it in a caculator or excel but written out equations and seeing my information plugged in so I can understand. Thank you. Calculate the simple linear regression to determine if the amount of time spent on homework can be predicted by amount of sleep. Graph the relationship and determine, numerically, if there are any outliers. Interpret all results in a paragraph citing the appropriate statisitcs.arrow_forwardListed below are the overhead widths (cm) of seals measured from photographs and weights (kg) of the seals. Find the regression equation, letting the overhead width be the predictor (x) variable. Find the best predicted weight of a seal if the overhead width measured from a photograph is 1.8 cm, using the regression equation. Can the prediction be correct? If not, what is wrong? Use a significance level of 0.05. Overhead Width (cm) 7.3 7.4 9.8 9.5 8.8 8.5 Weight (kg) 152 187 286 247 237 231 The regression equation is y =+ (x. (Round the y-intercept to the nearest integer as needed. Round the slope to one decimal place as needed.)arrow_forward
- Please show all working manuallyarrow_forwardplease assist with this non graded problem.arrow_forwardThe accompanying scatterplot shows the relationship between the age of an internet user and the amount of time spent browsing the internet per week (in minutes). The accompanying residual plot is also shown along with the QQ plot of the residuals. Choose the statement that best describes whether the condition for Normality of errors does or does not hold for the linear regression model. Choose the statement that best describes whether the condition for Normality of errors does or does not hold for the linear regression model. A.The residual plot displays a fan shape; therefore the Normality condition is not satisfied.B.The QQ plot mostly follows a straight line; therefore the Normality condition is satisfied.C.The scatterplot shows a negative trend; therefore the Normality condition is satisfied.D.The residual plot shows no trend; therefore the Normality condition is not satisfied.arrow_forward
- MATLAB: An Introduction with ApplicationsStatisticsISBN:9781119256830Author:Amos GilatPublisher:John Wiley & Sons IncProbability and Statistics for Engineering and th...StatisticsISBN:9781305251809Author:Jay L. DevorePublisher:Cengage LearningStatistics for The Behavioral Sciences (MindTap C...StatisticsISBN:9781305504912Author:Frederick J Gravetter, Larry B. WallnauPublisher:Cengage Learning
- Elementary Statistics: Picturing the World (7th E...StatisticsISBN:9780134683416Author:Ron Larson, Betsy FarberPublisher:PEARSONThe Basic Practice of StatisticsStatisticsISBN:9781319042578Author:David S. Moore, William I. Notz, Michael A. FlignerPublisher:W. H. FreemanIntroduction to the Practice of StatisticsStatisticsISBN:9781319013387Author:David S. Moore, George P. McCabe, Bruce A. CraigPublisher:W. H. Freeman
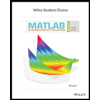
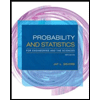
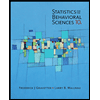
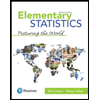
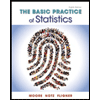
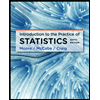